Machine Learning-Based Prediction of Post-Thrombotic Syndrome: A Model Development and Validation Study
SSRN Electronic Journal(2022)
摘要
Background: Prevention plays a key role in reducing the incidence of post-thrombotic syndrome (PTS). We aimed to develop accurate models with machine learning (ML) algorithms to predict the occurrence of PTS in 24 months.Methods: The clinical data used for model building was obtained from ATTRACT study and the validation cohort was acquired from Sun Yat-sen Memorial hospital in China. The main outcome was defined as occurrence of PTS event (Villalta score ≥5). 23 clinical variables were comprised and four ML algorithms were applied to build models. Discrimination and calibration, F score were exploited to evaluate the prediction ability of the models. Based on risk estimate deciles, the validation cohort was divided into ten groups to identify the hazard threshold.Findings: 555 deep vein thrombosis (DVT) patients were included to build models with ML algorithms and the models were further validated in a Chinese cohort comprising 117 patients. When predicting PTS in 2 years after acute DVT, logistic regression (LR) based on gradient descent and L1 regularization got highest area under the curve (AUC) of 0.83 (95% CI: 0.76-0.89) in external validation. When considering model performance in both training and validation cohort, eXtreme gradient boosting (XGBoost) and gradient boosting decision tree (GBDT) models had similar results and presented better stability and generalization. The validation cohort was divided into low, intermediate, high-risk groups with the prediction probability of 0.3 and 0.4 as critical points.Interpretation: ML models built for PTS had accurate prediction ability and stable generalization, which can further facilitate clinical decision-making, with potentially important implications on selecting patients that will benefit from endovascular surgery.Funding: The research was funded by National Natural Science Foundation of China [Project approval number is 81800420].Declaration of Interest: None to declare. Ethical Approval: Patient informed consent was obtained as approved by the Ethics Committee of Sun Yet-sen Memorial Hospital and in accordance with the ethical principles for medical research involving human subjects as set out in the Declaration of Helsinki.
更多查看译文
关键词
prediction,learning-based,post-thrombotic
AI 理解论文
溯源树
样例
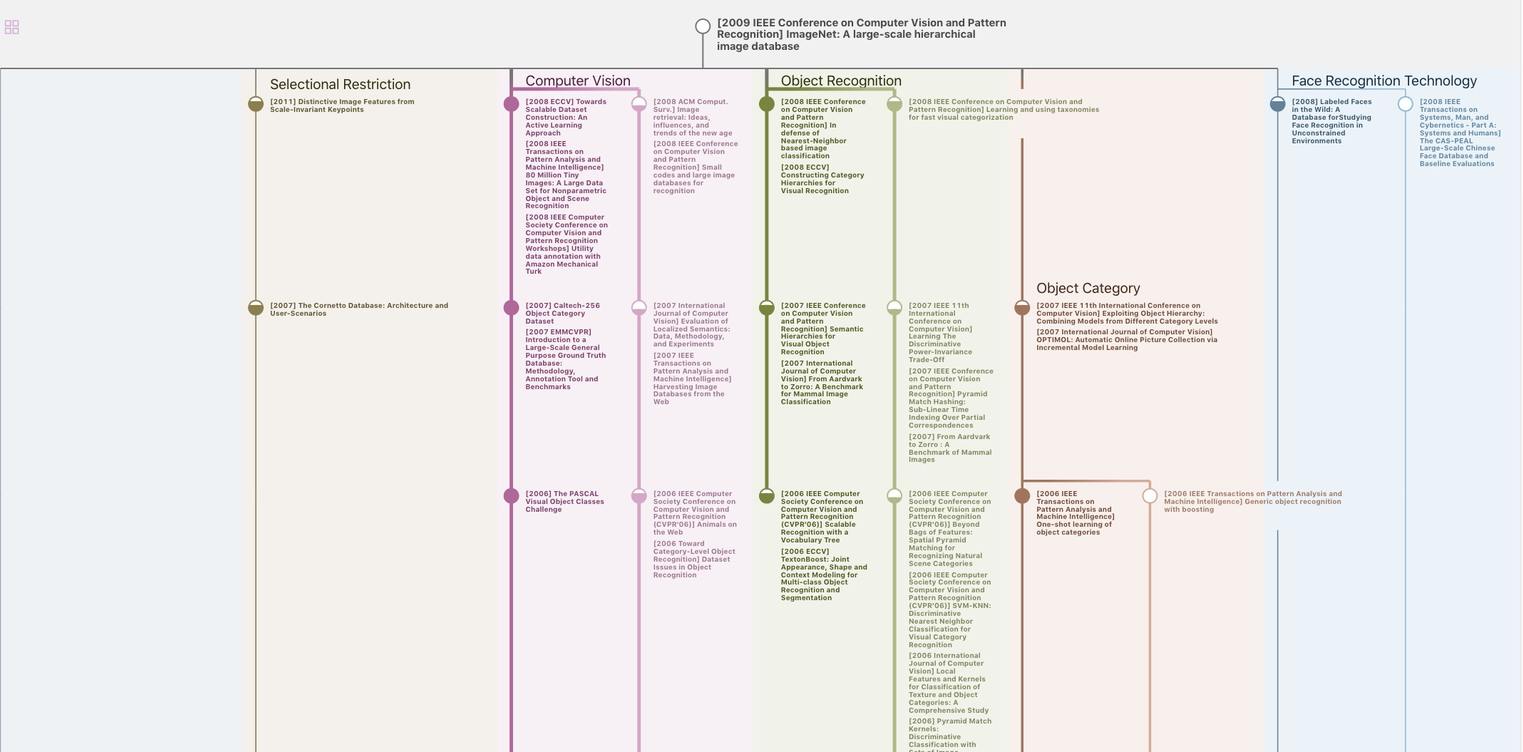
生成溯源树,研究论文发展脉络
Chat Paper
正在生成论文摘要