Decoupling Trends and Changepoint Analysis
semanticscholar(2022)
摘要
This paper introduces a new Bayesian changepoint approach called the decoupled approach that separates the process of modeling and changepoint analysis. The approach utilizes a Bayesian dynamic linear model (DLM) for the modeling step and a weighted penalized likelihood estimator on the posterior of the Bayesian DLM to identify changepoints. A Bayesian DLM, with shrinkage priors, can provide smooth estimates of the underlying trend in presence of complex noise components; however, the inability to shrink exactly to zero make changepoint analysis difficult. Penalized likelihood estimators can be effective in estimating location of changepoints; however, they require a relatively smooth estimate of the data. The decoupled approach combines the flexibility of the Bayesian DLM along with the hard thresholding property of penalized likelihood estimator to extend application of changepoint analysis. The approach provides a robust framework that allows for identification of changepoints in highly complex Bayesian models. The approach can identify changes in mean, higher order trends and regression coefficients. We illustrate the approach’s flexibility and robustness by comparing against several alternative methods in a wide range of simulations and two real world
更多查看译文
AI 理解论文
溯源树
样例
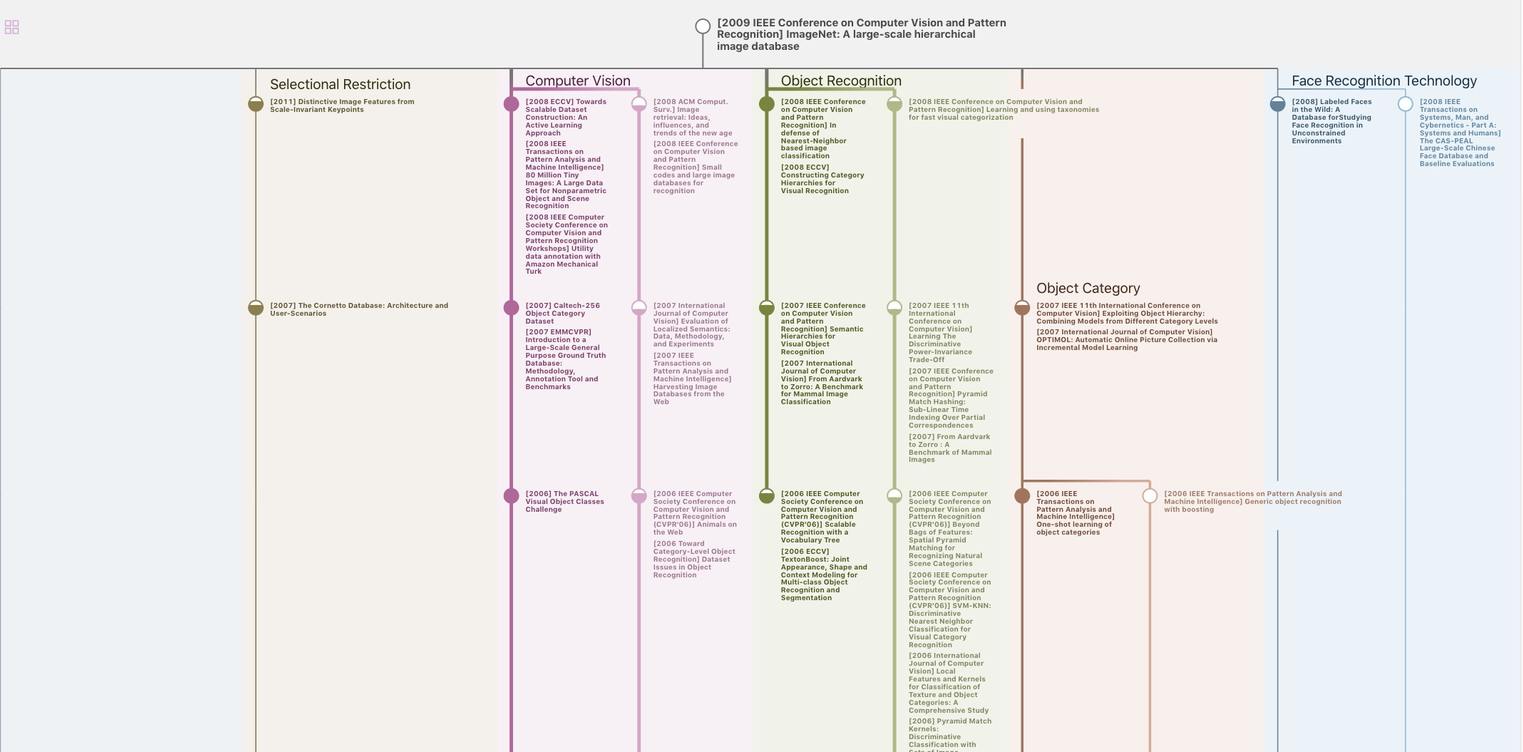
生成溯源树,研究论文发展脉络
Chat Paper
正在生成论文摘要