Environmental Factors Assisted the Evaluation of Entropy Water Quality Indices with Efficient Machine Learning Technique
Water Resources Management(2022)
摘要
Water is an indispensable resource for human production and life. The evaluation of water quality by scientific methods provides sufficient support for the regeneration and recycling of water resources. In this study, entropy theory was used to evaluate water quality and overcomes the limitations of traditional water quality assessment, which does not consider the impact of different environmental factors on water quality. Considering the complexity of the traditional evaluation process, two typical machine learning (ML) methods – generalized regression neural network (GRNN) and support vector machine (SVM) – were used to predict the entropy water quality index (EWQI). Correlation analysis was applied to divide environmental factors into different combinations that subsequently acted as the input vector for the ML model. According to the results of the root mean squared error (RMSE), the SVM was selected as the better prediction model. Then, four different types of optimization algorithms were used to optimize the SVM to calculate nonlinear regression predictions and classifications of water quality. After analyzing the prediction results with different types of scientific evaluation indicators, the algorithm of differential evaluation and gray wolf optimization (DE-GWO) achieved markedly better performance than the other three algorithms, which has important advantages in avoiding the prediction model falling into a local optimal solution. The results of this study have significant guidance for water quality prediction and could make further contributions to the rational use and protection of water resources.
更多查看译文
关键词
Water quality,Correlation analysis,Machine learning method,Nonlinear regression prediction,Nonlinear regression classification
AI 理解论文
溯源树
样例
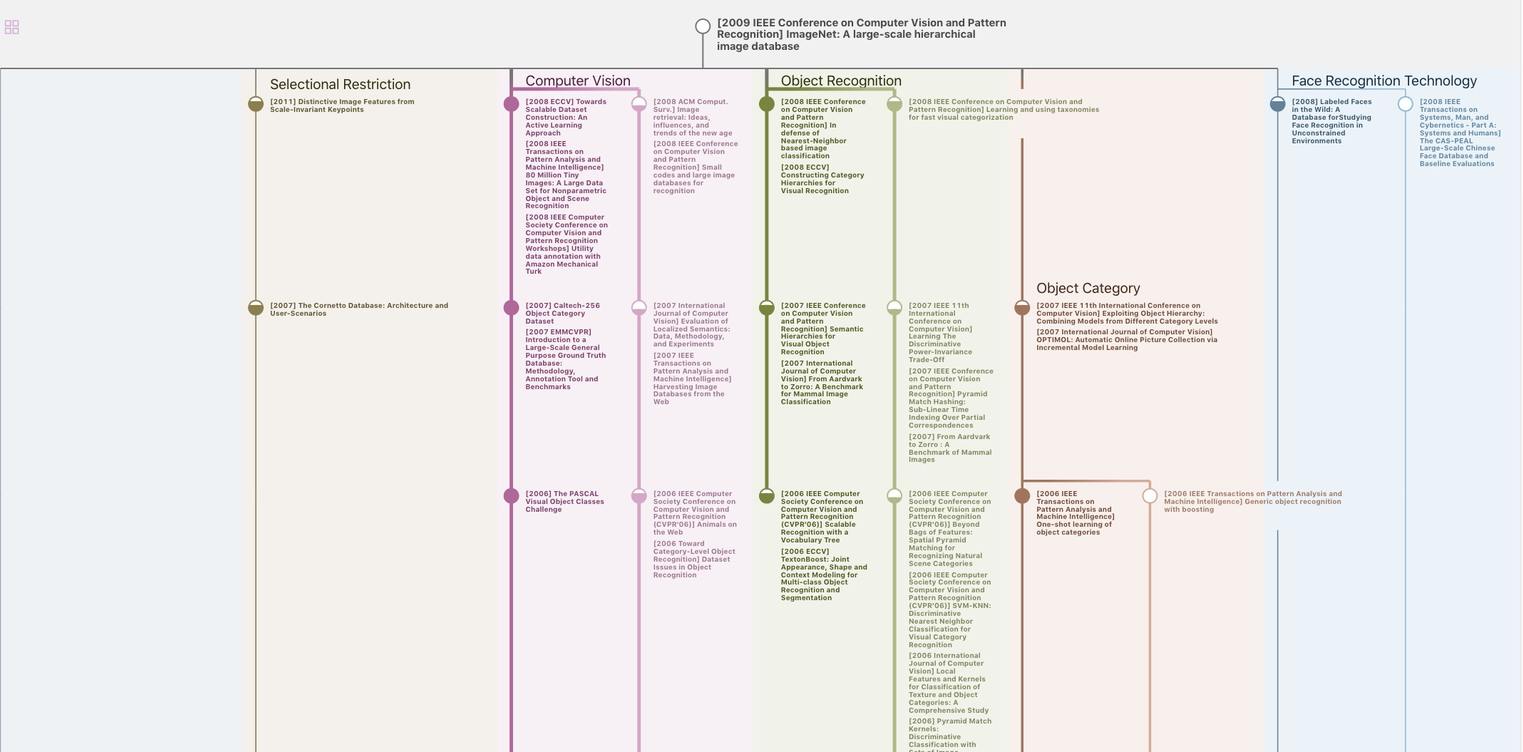
生成溯源树,研究论文发展脉络
Chat Paper
正在生成论文摘要