Knowledge-enhanced graph convolutional network for recommendation
Multimedia Tools and Applications(2022)
摘要
Recommendation systems based on collaborative filtering (CF) are often accompanied by cold start and sparsity issues, which can be alleviated by using user information, item attributes and optimizing algorithms fully and reasonably. Empirically, the side information, such as item attributes, is not isolated but connected to each other in the form of the knowledge graph (KG). In this article, we put forward Knowledge-Enhanced Graph Convolutional Network (KE-GCN), a well-designed end-to-end architecture for recommendation, mining associated attributes of inter-item on the KG. To capture relatedness of inter-item effectively, we improve the attention mechanism to better probe into the correlation of the item’s neighbors, and design a new aggregator to enhance feature aggregation. We conducted experiments on three public benchmarks, and empirical results of them show that KE-GCN is markedly superior to state-of-the-art methods.
更多查看译文
关键词
Knowledge-enhanced recommendation, Graph convolutional network, Aggregator, Attention mechanism
AI 理解论文
溯源树
样例
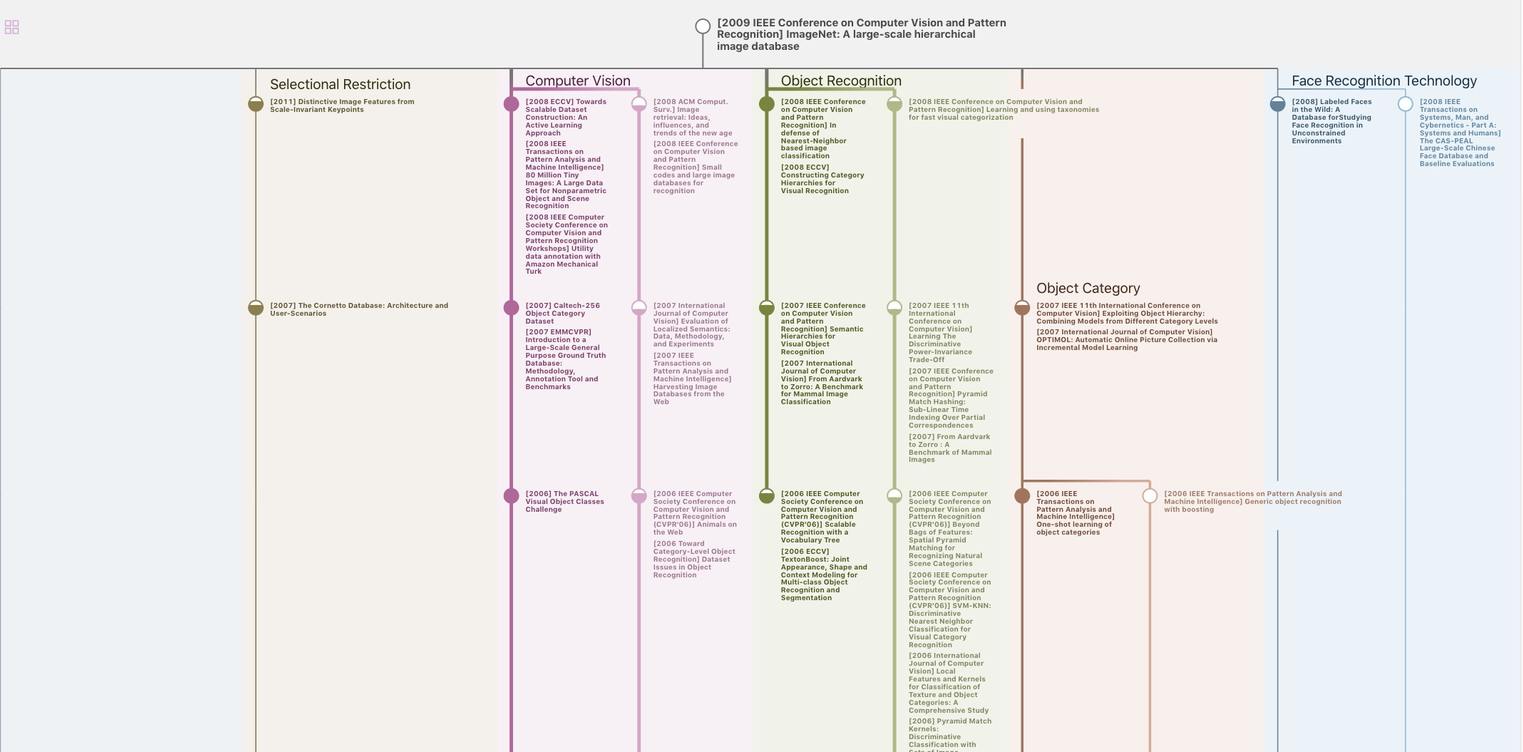
生成溯源树,研究论文发展脉络
Chat Paper
正在生成论文摘要