MCMC Should Mix: Learning Energy-Based Model with Neural Transport Latent Space MCMC
International Conference on Learning Representations (ICLR)(2022)
Abstract
Learning energy-based model (EBM) requires MCMC sampling of the learned model as an inner loop of the learning algorithm. However, MCMC sampling of EBMs in high-dimensional data space is generally not mixing, because the energy function, which is usually parametrized by a deep network, is highly multi-modal in the data space. This is a serious handicap for both theory and practice of EBMs. In this paper, we propose to learn an EBM with a flow-based model (or in general a latent variable model) serving as a backbone, so that the EBM is a correction or an exponential tilting of the flow-based model. We show that the model has a particularly simple form in the space of the latent variables of the backbone model, and MCMC sampling of the EBM in the latent space mixes well and traverses modes in the data space. This enables proper sampling and learning of EBMs.
MoreTranslated text
Key words
neural transport
AI Read Science
Must-Reading Tree
Example
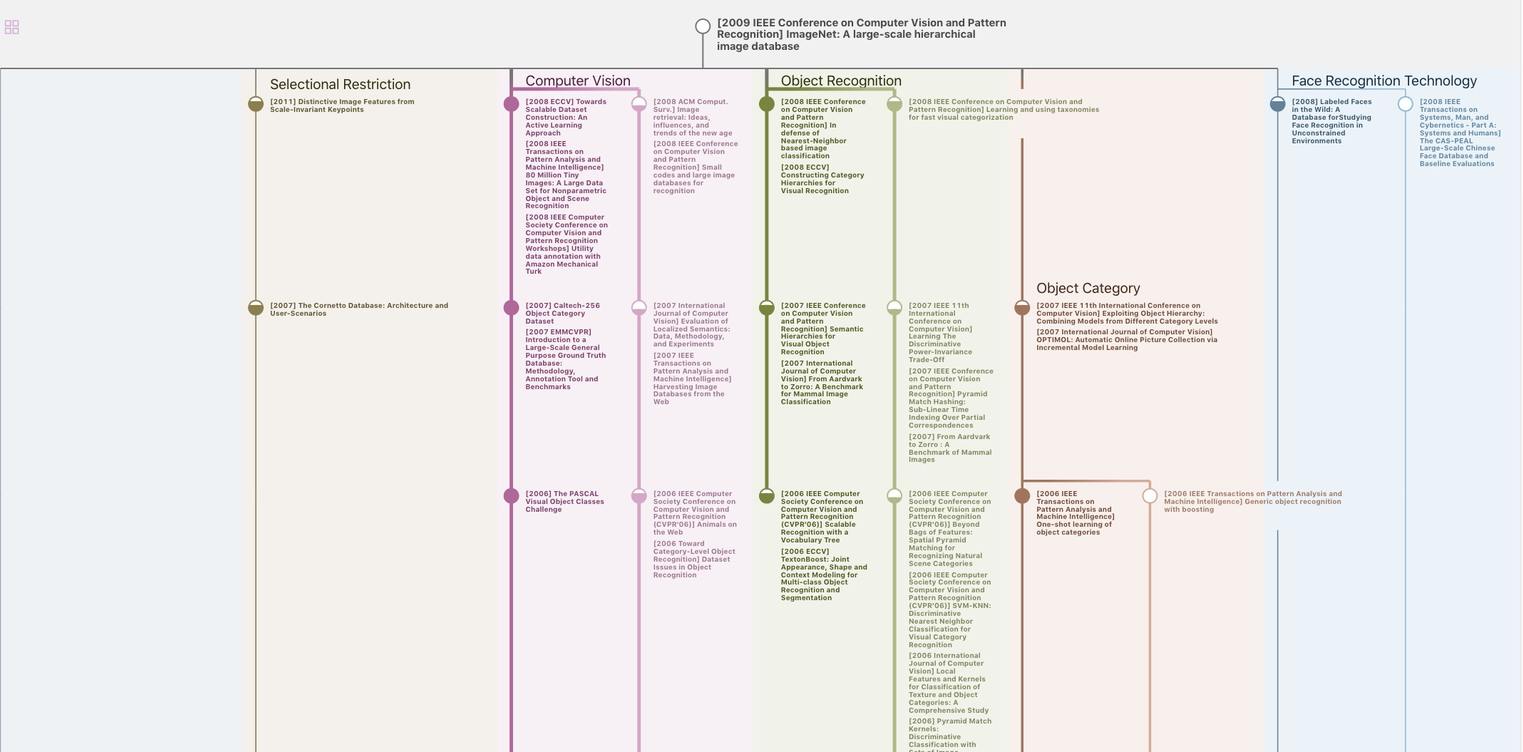
Generate MRT to find the research sequence of this paper
Chat Paper
Summary is being generated by the instructions you defined