A Meta-Learning-Assisted Training Framework for Physical Layer Modeling in Optical Networks
Journal of Lightwave Technology(2022)
Abstract
An accurate quality of transmission (QoT) estimation can help to reduce the
design margin
of optical network planning. The physical layer impairments (PLI) modeling can be the basis of QoT estimation and support upper layer applications. Due to the complex structure of the physical layer and imperfect knowledge of network parameters, improving PLI modeling performance is hindered by the parameter uncertainty. The parameter uncertainty can be alleviated by online adaptation using data from the real system. However, obtaining the accurate value of each PLI is difficult since multiple impairments co-exist in the optical system. Therefore, updating PLI models with online adaptation is difficult, which requires PLI models with higher robustness to parameter uncertainty and more efficient adaptation ability with limited data from real systems. In this paper, we propose a meta-learning-assisted training framework for machine-learning-based physical layer models. This framework can improve the model robustness to agnostic uncertain parameters during offline training and enables the model to efficiently adapt to the real system with fewer data. Use cases of dealing with parameter uncertainty in fiber nonlinearity modeling and QoT estimation are performed to prove the concept through simulations, which demonstrates the robustness improvement and
few-shot learning
ability.
MoreTranslated text
Key words
Fiber nonlinearity modeling,meta learning,online learning,physical layer modeling
AI Read Science
Must-Reading Tree
Example
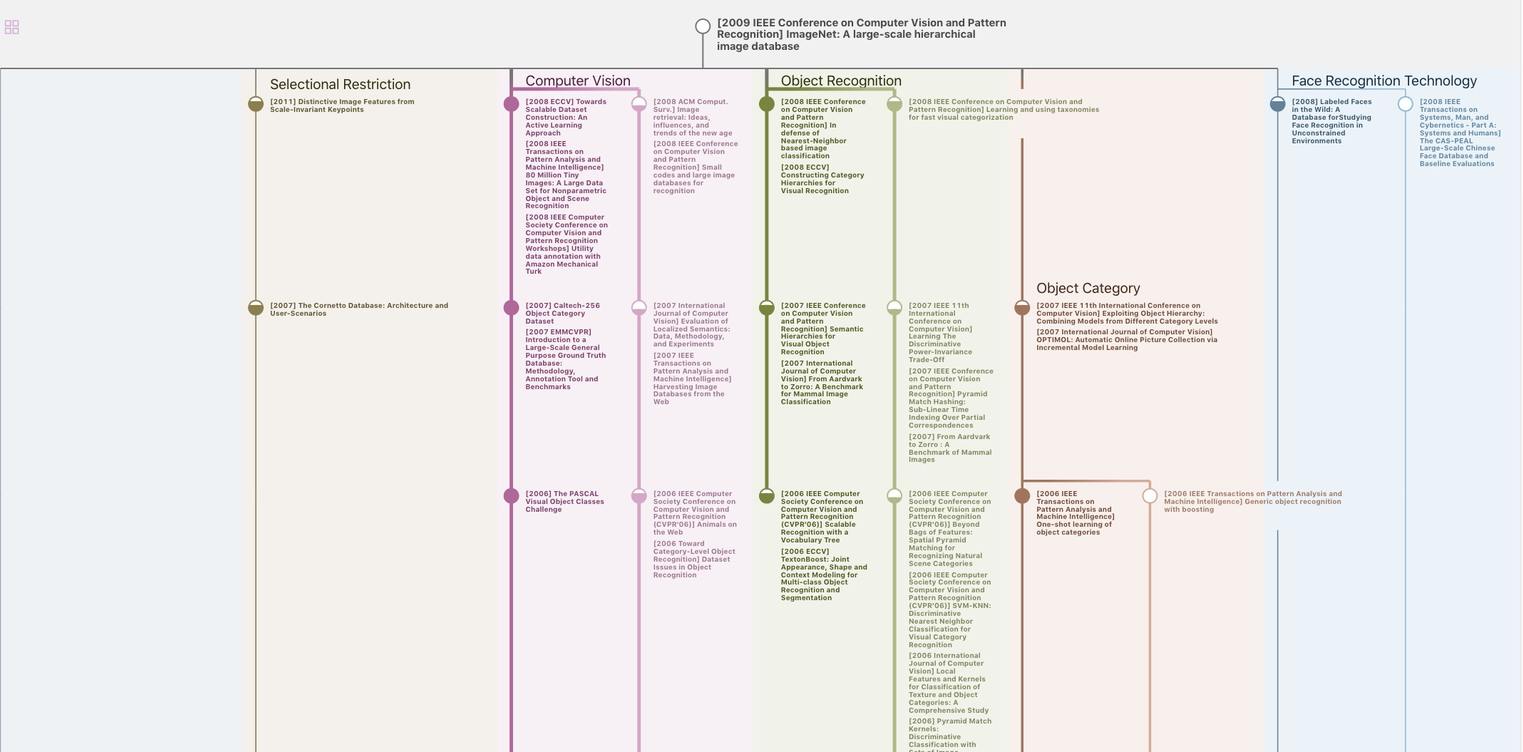
Generate MRT to find the research sequence of this paper
Chat Paper
Summary is being generated by the instructions you defined