Scheduling for Massive MIMO With Hybrid Precoding Using Contextual Multi-Armed Bandits
IEEE Transactions on Vehicular Technology(2022)
摘要
In this work we study different scheduling problems in the downlink of a Frequency Division Duplex multiuser wireless system that employs a hybrid precoding antenna architecture for massive Multiple Input Multiple Output. In this context, we propose a scheduling framework using Reinforcement Learning (RL) tools, namely Contextual Multi-Armed Bandits (CMAB), that can dynamically adapt themselves to solve three scheduling problems, which are: i) Maximum Throughput (MT); ii) Maximum Throughput with Fairness Guarantees (MTFG), and; iii) Maximum Throughput with QoS Guarantees (MTQG), which are well-known relevant problems. Before performing scheduling itself, we exploit statistical Channel State Information (CSI) to create clusters of spatially compatible User Equipmentss (UEss). This structure, combined with the usage of Zero-Forcing precoding, allows us to reduce the scheduler complexity by considering each cluster as an independent virtual RL scheduling agent. Next, we apply a new learning-based scheduler aiming to optimize the desired system performance metric. Moreover, only scheduled UEss need to feed back instantaneous equivalent CSI, which also reduces the signaling overhead of the proposal. The superiority of the proposed framework is demonstrated through numerical simulations in comparison with reference solutions.
更多查看译文
关键词
Multi-Armed Bandits,Reinforcement Learning,Scheduling,Massive MIMO
AI 理解论文
溯源树
样例
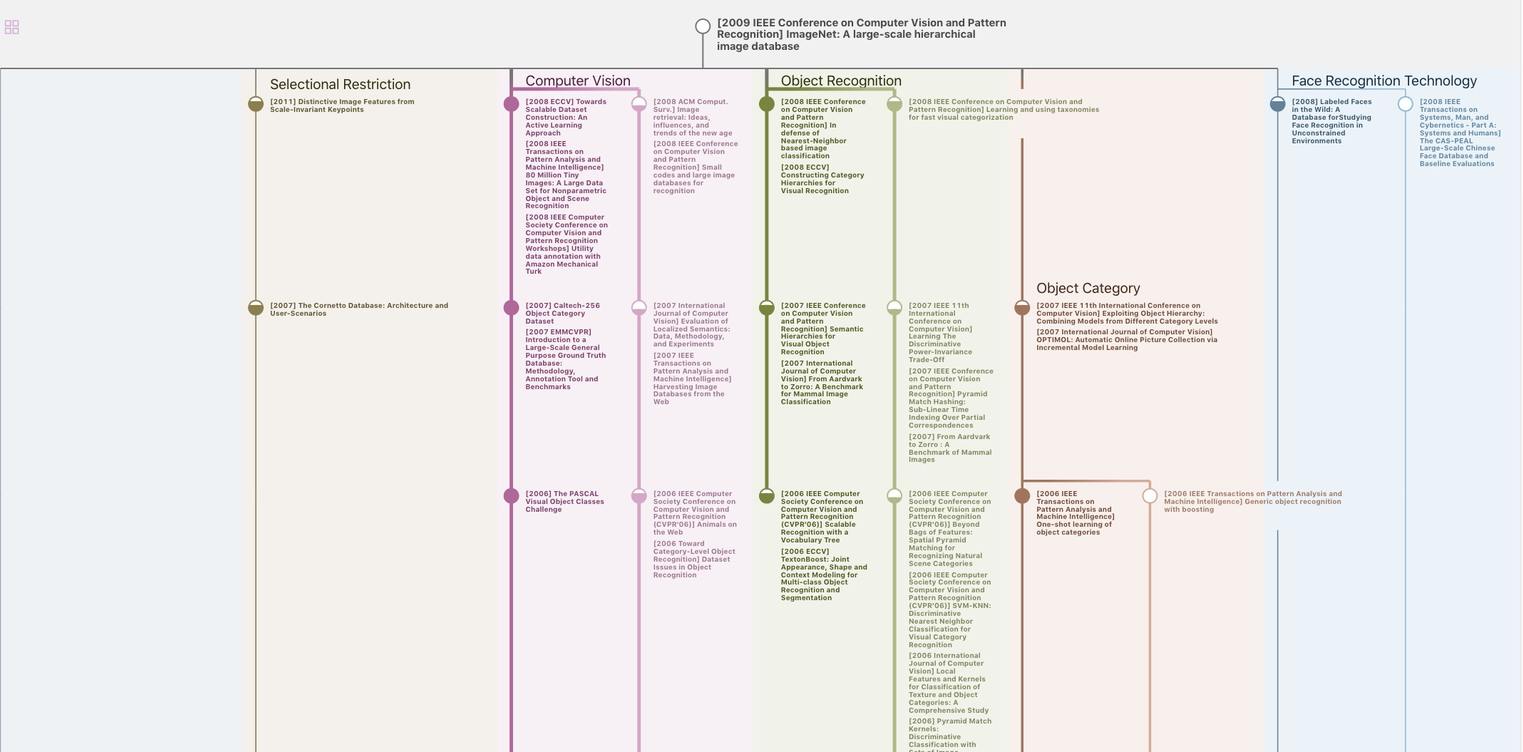
生成溯源树,研究论文发展脉络
Chat Paper
正在生成论文摘要