Autonomic scalability control for cloud workloads with Bayesian network.
International Journal of Cloud Computing(2022)
摘要
Cloud computing facilitates access to infrastructure, platform, and software to clients over the internet, on-demand. Clients run applications on cloud which are supported by virtual machines (VMs) running on top of physical machines (PM). Workload traffic coming to the cloud varies over time. To meet these changing workload demands the VMs must be scaled up and scaled down automatically to ensure that the service level agreement (SLA) parameters are not violated and client's quality of service (QoS) is maintained. This auto-scaling can be realised with a machine learning (ML) technique - Bayesian network (BN). In this article we propose a framework for autonomic scalability of cloud resources. The framework takes cloud data centre management dataset, selects SLA parameters of choice, discretises data of selected features, learns structural dependencies between these features and draws a BN, learns parameters, validates model, and after that makes decisions to either scale up or scale down on the basis of predictive and diagnostic capabilities of the BN model. The results achieved after evaluation are logical and consistent as prediction of workload demands and diagnosis of under utilisation of cloud resources is done with sufficient accuracy.
更多查看译文
关键词
autonomic scalability control,cloud workloads,bayesian network
AI 理解论文
溯源树
样例
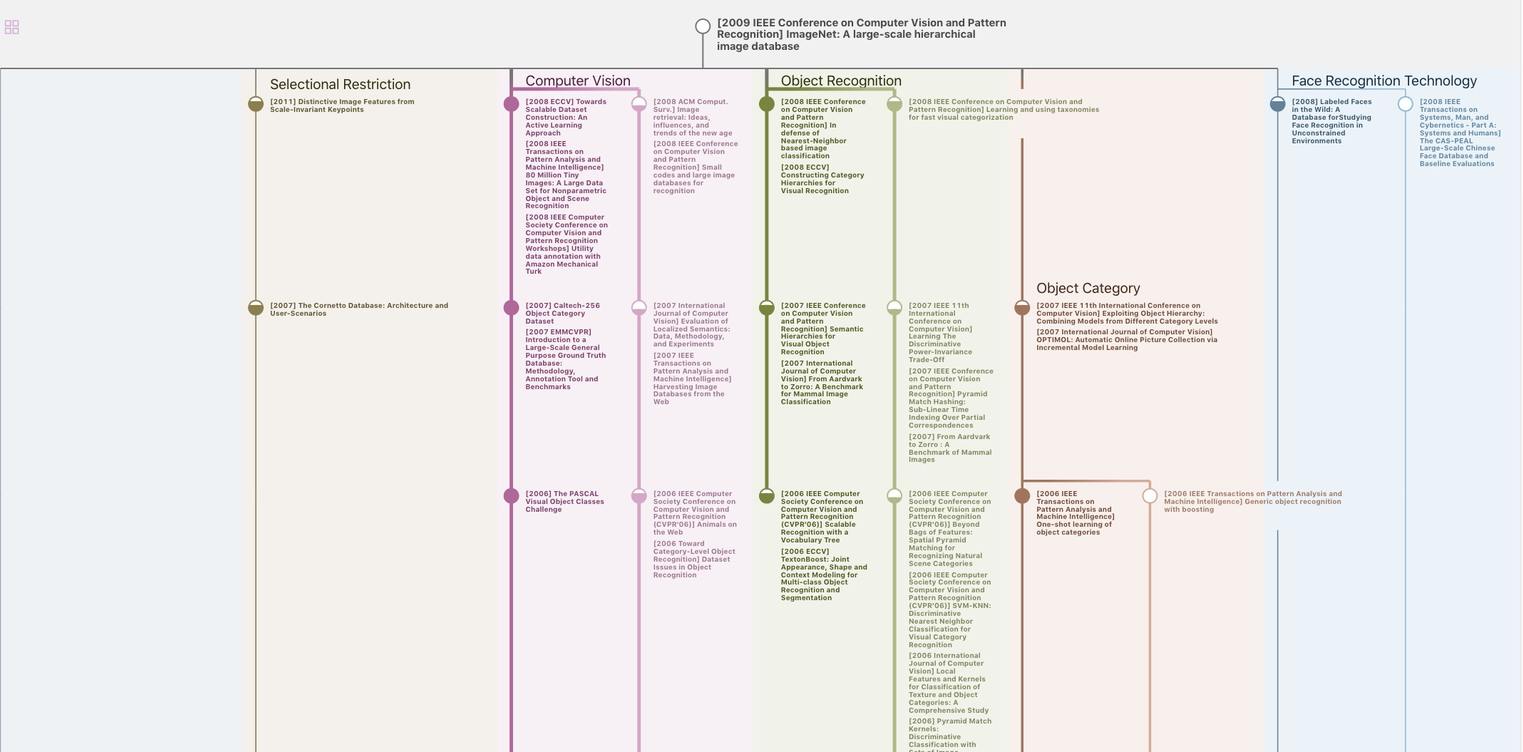
生成溯源树,研究论文发展脉络
Chat Paper
正在生成论文摘要