Full-Cycle Energy Consumption Benchmark for Low-Carbon Computer Vision
CoRR(2021)
Abstract
The energy consumption of deep learning models is increasing at a breathtaking rate, which raises concerns due to potential negative effects on carbon neutrality in the context of global warming and climate change. With the progress of efficient deep learning techniques, e.g., model compression, researchers can obtain efficient models with fewer parameters and smaller latency. However, most of the existing efficient deep learning methods do not explicitly consider energy consumption as a key performance indicator. Furthermore, existing methods mostly focus on the inference costs of the resulting efficient models, but neglect the notable energy consumption throughout the entire life cycle of the algorithm. In this paper, we present the first large-scale energy consumption benchmark for efficient computer vision models, where a new metric is proposed to explicitly evaluate the full-cycle energy consumption under different model usage intensity. The benchmark can provide insights for low carbon emission when selecting efficient deep learning algorithms in different model usage scenarios.
MoreTranslated text
Key words
energy,vision,full-cycle,low-carbon
AI Read Science
Must-Reading Tree
Example
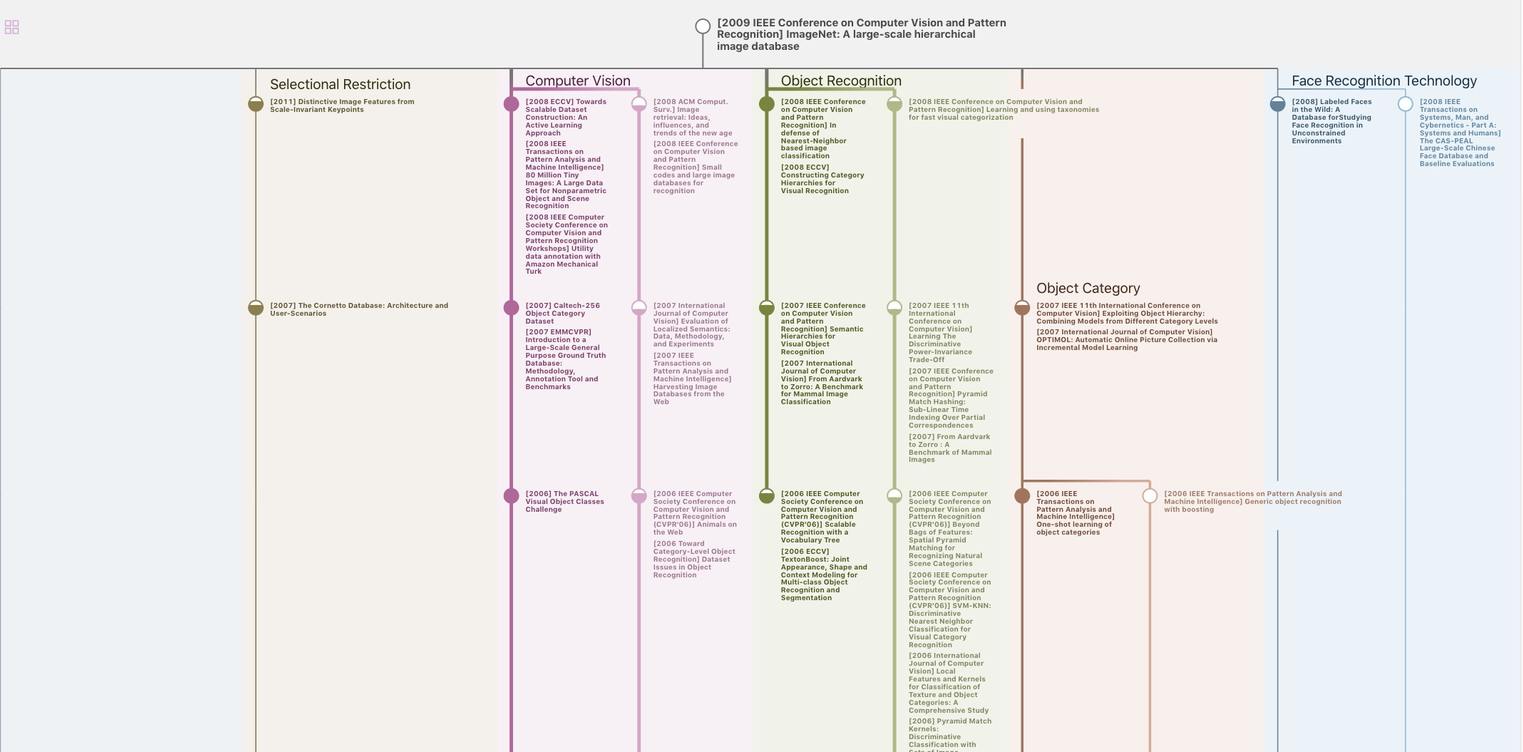
Generate MRT to find the research sequence of this paper
Chat Paper
Summary is being generated by the instructions you defined