A Multi-Stage Automatic Evaluation System for Sight-Singing
IEEE Transactions on Multimedia(2023)
摘要
Sight-singing exercises are a fundamental part of music education. In this paper, we present an objective and complete automatic evaluation system for sight-singing, which has two critical stages: note transcription and note alignment. In the first stage, we use an onset detector based on the convolutional recurrent neural network (CRNN) for note segmentation and the pitch extractor described in (Kim et al. 2018) for note labeling. In the second stage, an alignment algorithm based on relative pitch modeling is proposed. Due to the lack of datasets for sight-singing note alignment and the overall system evaluation, we construct the sight-singing vocal dataset (SSVD). Each module of the system and the entire system are tested on this dataset. The onset detector achieves an F-measure of 90.61%, and the stages of note transcription and note alignment achieve an F-measure of 88.42% and 94.79%, respectively. In addition, we propose an objective criterion for the sight-singing evaluation system. Based on this criterion, our automatic sight-singing system achieves an F-measure of 77.95% on the SSVD dataset.
更多查看译文
关键词
Music,Spectrogram,Feature extraction,Hidden Markov models,Detectors,Convolutional neural networks,Deep learning,Automatic sight-singing system,note alignment,sight-singing transcription,systematic evaluation measure
AI 理解论文
溯源树
样例
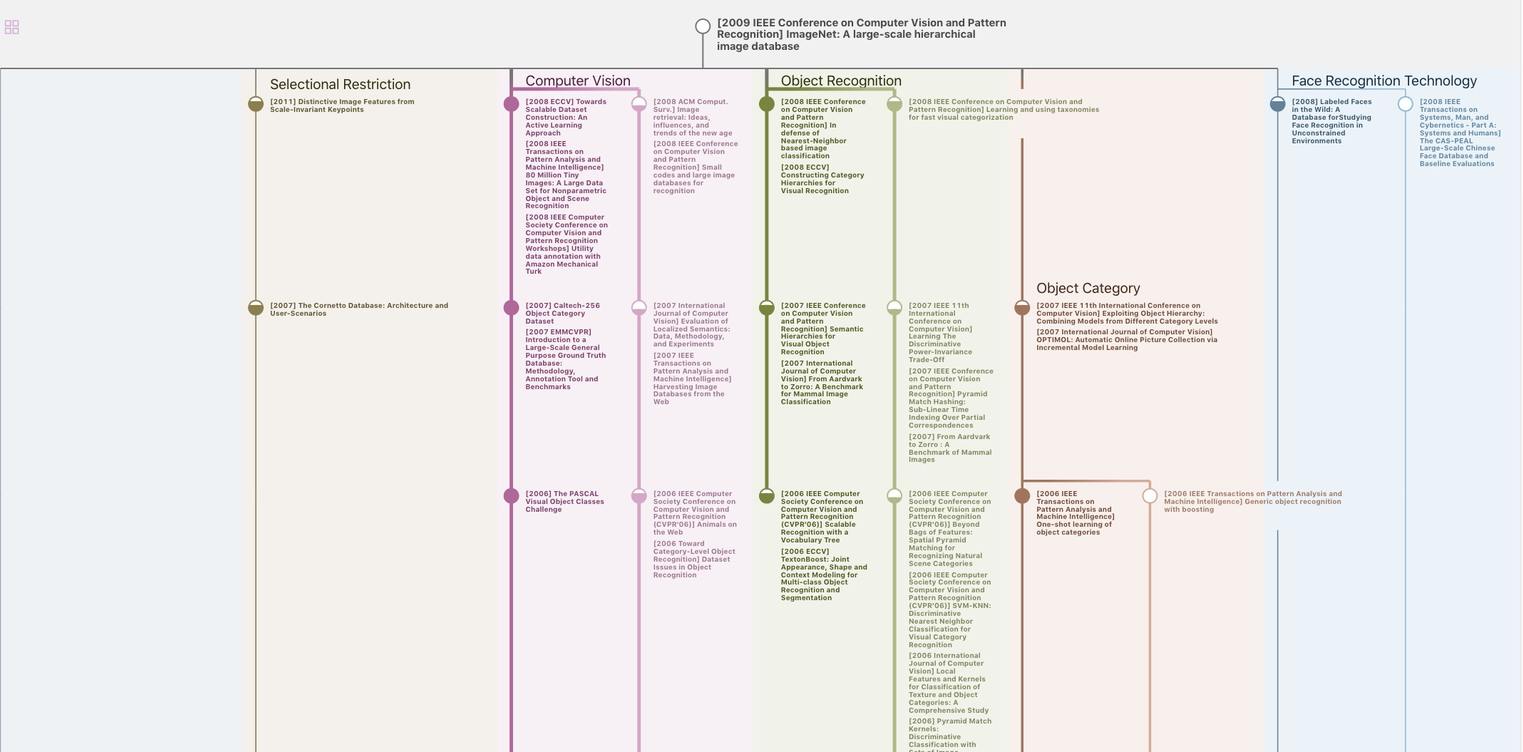
生成溯源树,研究论文发展脉络
Chat Paper
正在生成论文摘要