Believe The HiPe: Hierarchical Perturbation for Fast, Robust, and Model-Agnostic Saliency Mapping
semanticscholar(2021)
摘要
Understanding the predictions made by Artificial Intelligence (AI) systems is becoming more and more important as deep learning models are used for increasingly complex and high-stakes tasks. Saliency mapping – a popular visual attribution method – is one important tool for this, but existing formula-tions are limited by either computational cost or architectural constraints. We therefore propose Hierarchical Perturbation, a very fast and completely model-agnostic method for interpreting model predictions with robust saliency maps. Using standard benchmarks and datasets, we show that our saliency maps are of competitive or superior quality to those generated by existing model-agnostic methods – and are over 20 × faster to compute.
更多查看译文
AI 理解论文
溯源树
样例
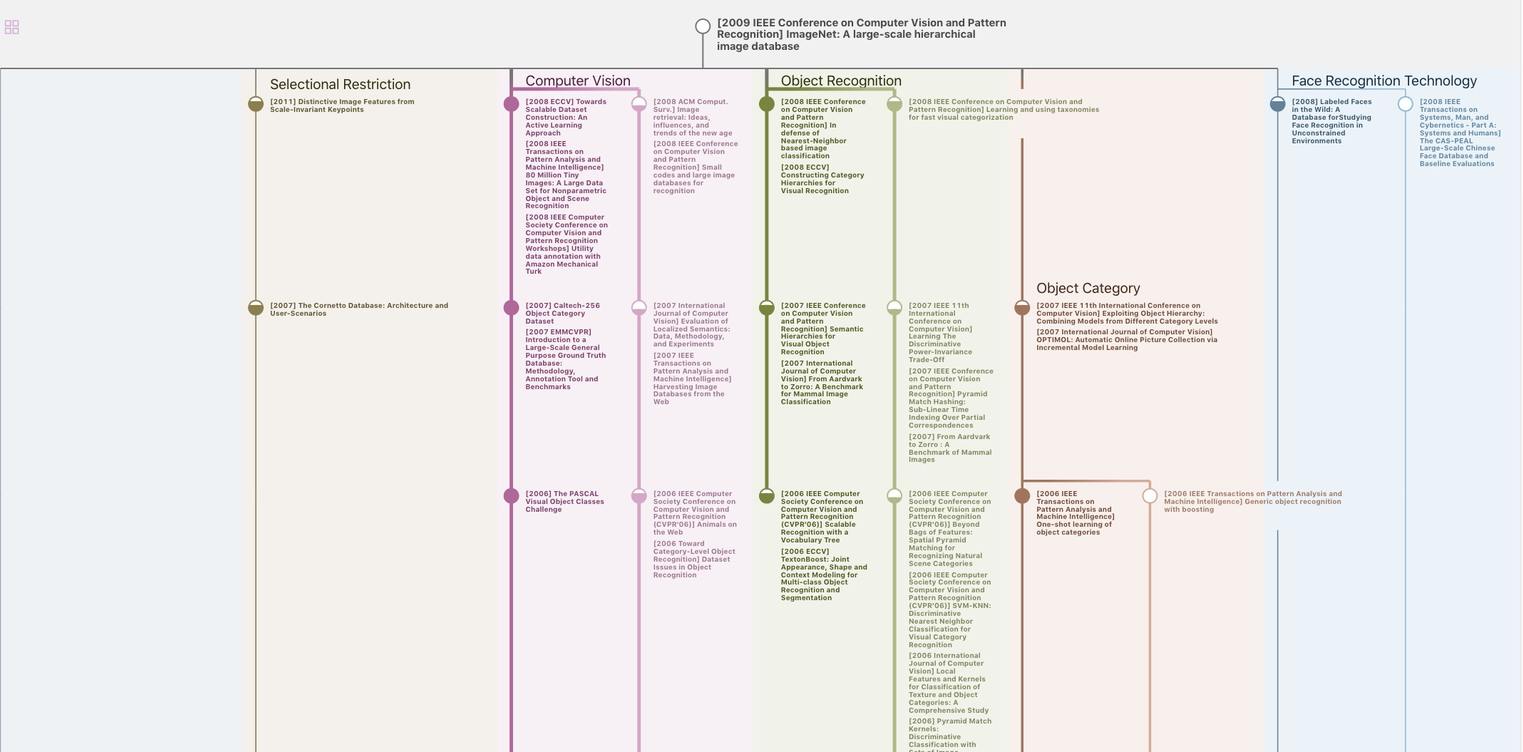
生成溯源树,研究论文发展脉络
Chat Paper
正在生成论文摘要