Identification of MOSFET Working State Based on the Stress Wave and Deep Learning
IEEE TRANSACTIONS ON INSTRUMENTATION AND MEASUREMENT(2022)
摘要
Nondestructive testing methods are gradually used to monitor the state of power devices, including acoustic emission (AE) testing. However, the application of AE in power devices is still in the research stage and relies on manual analysis. In this article, the stress wave signal of the device working under different electrical parameters is combined with deep learning (DL) for the first time to judge the working state of the device. Time-domain signals, frequency-domain amplitudes, and envelope of the stress wave and their corresponding label are used for the training of the artificial neural network (ANN), 1-D convolutional neural network (1DCNN), long short-term memory (LSTM), and recurrent neural network (RNN). The training effect of 1DCNN combined with time-domain series is the best, and the accuracy rate can reach up to 100%. Compared with other classic 1DCNN models, the proposed 1DCNN method does not require complex feature extraction and model calculations, which accurately identifies the working state of the device and also improves its speed.
更多查看译文
关键词
Stress,Acoustic emission,Monitoring,Probes,Time-domain analysis,MOSFET,Low-pass filters,1-D convolutional neural network (1DCNN),acoustic emission (AE),deep learning (DL),identification,MOSFET
AI 理解论文
溯源树
样例
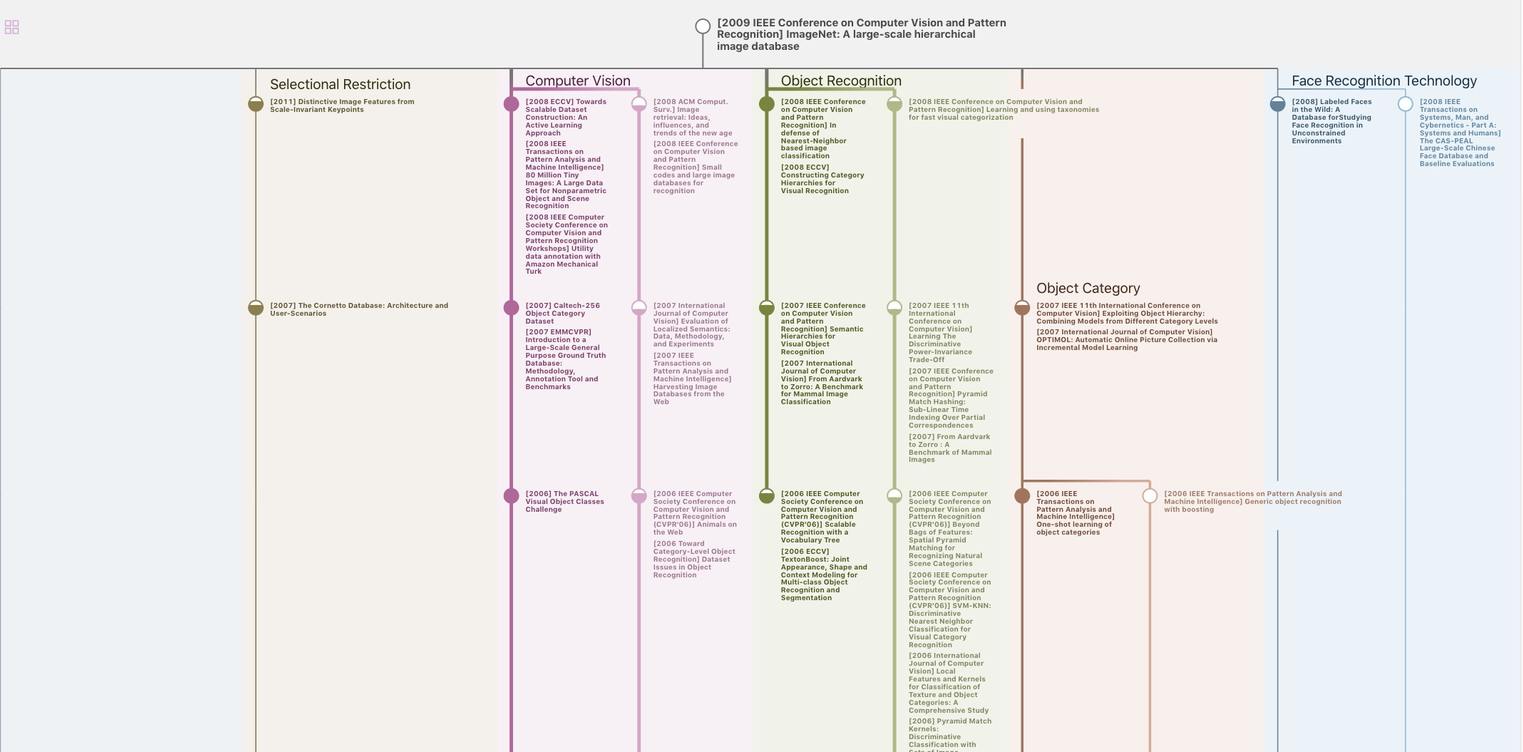
生成溯源树,研究论文发展脉络
Chat Paper
正在生成论文摘要