Memory-Oriented Unpaired Learning for Single Remote Sensing Image Dehazing
IEEE GEOSCIENCE AND REMOTE SENSING LETTERS(2022)
摘要
Remote sensing image dehazing (RSID) is an extremely challenging problem due to the irregular and nonuniform distribution of haze. The existing RSID methods achieve excellent performance using deep learning; however, relying on paired synthetic data is limited to their generality in various haze distribution. In this letter, we present a memory-oriented generative adversarial network (MO-GAN), which tries to capture the desired hazy features in an unpaired learning manner toward single RSID. For better extracting the haze-relevant features, a novel multistage attentive-recurrent memory module is designed to guide an autoencoder neural network, which can record the various appearances of haze distribution at different stages. To well differentiate fake images from real ones, a dual region discriminator is constructed to handle spatially varying haze densities in global and local regions. Extensive experiments demonstrate that our designed MO-GAN outperforms the recent comparing approaches on the various frequently used datasets, especially in real world nonuniform haze conditions. The source code is released in
https://github.com/cxtalk/MO-GAN
.
更多查看译文
关键词
Feature extraction,Generative adversarial networks,Training,Memory modules,Sensors,Image reconstruction,Task analysis,Attention mechanism,generative adversarial networks (GANs),haze removal,memory network,remote sensing (RS) image,unpaired learning
AI 理解论文
溯源树
样例
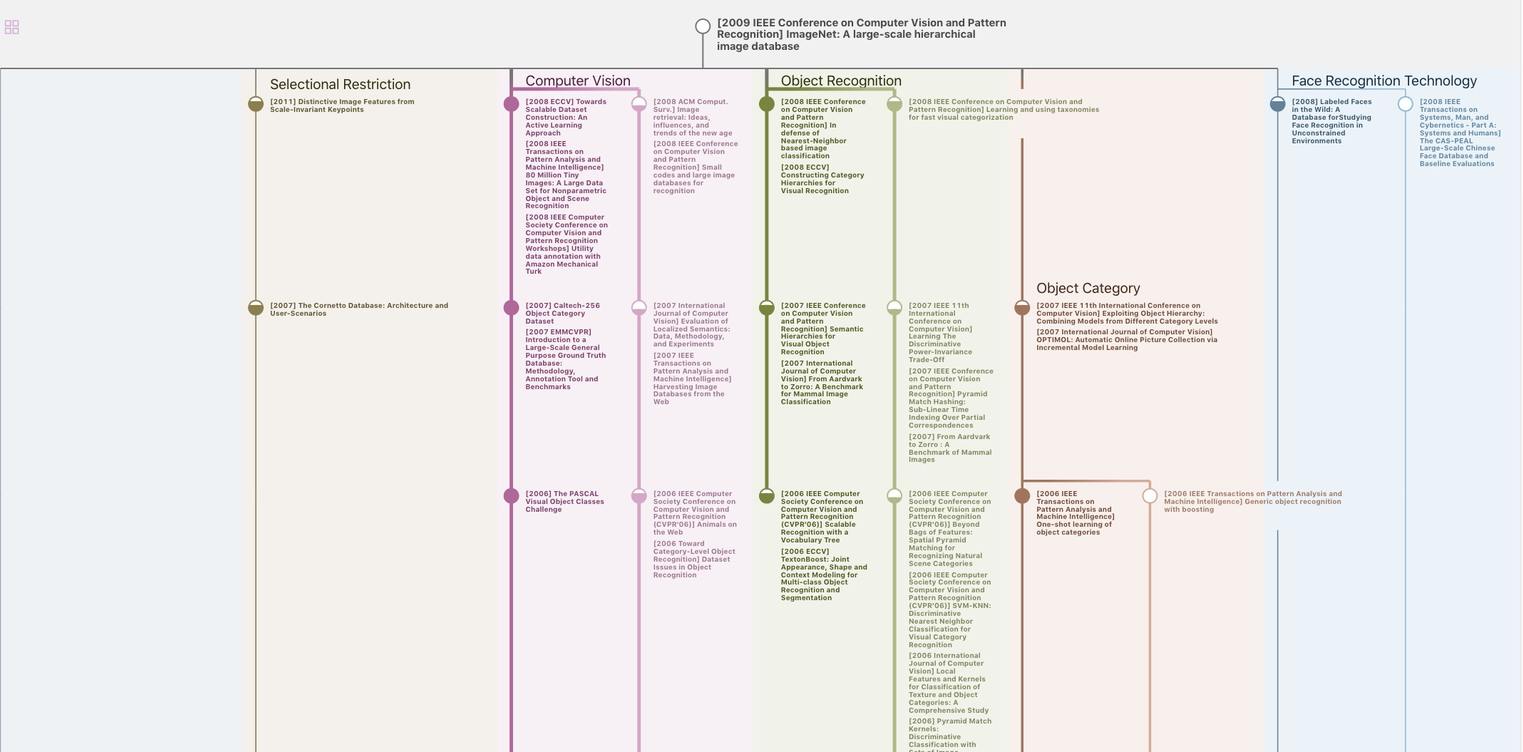
生成溯源树,研究论文发展脉络
Chat Paper
正在生成论文摘要