3D Deep Heterogeneous Manifold Network for Behavior Recognition
SECURITY AND COMMUNICATION NETWORKS(2022)
摘要
With the broadening of application scenarios for Internet of Things, intelligent behavior recognition task has attracted more and more attention. Since human behavior is nonrigid motion with strong spatiotemporal topological association, modeling it directly with traditional Euclidean space-based methods may destroy its underlying nonlinearity. Based on the advantages of Riemannian manifold in describing 3D motion, we propose an end-to-end 3D behavior manifold feature learning framework composed of deep heterogeneous networks. This heterogeneous architecture aims to leverage the graph construction to guide manifold backbone network to mine more discriminative nonlinear spatiotemporal features. Therefore, we first model the nonlinear spatiotemporal co-occurrence of 3D behavior in the high-dimensional Riemannian manifold space. Secondly, we implement a non-Euclidean heterogeneous architecture on the Riemannian manifold so that the backbone network can learn deep spatiotemporal features while preserving the manifold topology. Finally, an end-to-end deep graph similarity-guided learning optimization mechanism is introduced to enable the overall model to fully utilize the complex similarity relationship between manifold features. We have verified our 3D deep heterogeneous manifold network on popular skeleton behavior datasets and achieved competitive results.
更多查看译文
关键词
heterogeneous,recognition,3d,behavior
AI 理解论文
溯源树
样例
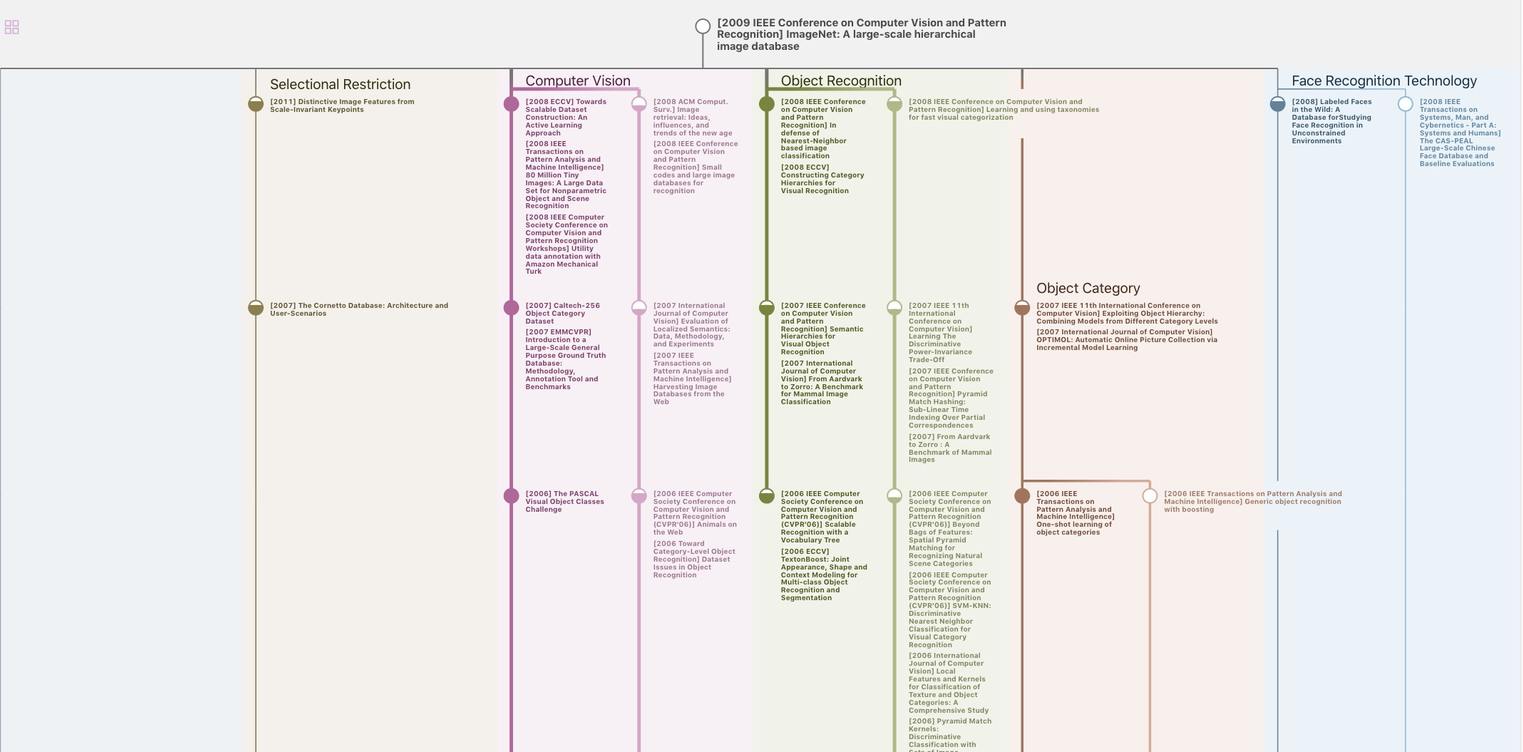
生成溯源树,研究论文发展脉络
Chat Paper
正在生成论文摘要