Copd_a_301466 1477..1496
semanticscholar(2021)
Abstract
Department of Mechanical Engineering, University of Iowa, Iowa City, IA, USA; IIHR-Hydroscience & Engineering, University of Iowa, Iowa City, IA, USA; Department of Biomedical Engineering, University of Iowa, Iowa City, IA, USA; Department of Internal Medicine, School of Medicine, University of Kansas, Kansas City, KS, USA; Department of Radiology, Perelman School of Medicine, University of Pennsylvania, Philadelphia, PA, USA; School of Mechanical Engineering, Kyungpook National University, Daegu, Republic of Korea; Department of Internal Medicine, University of Iowa, Iowa City, IA, USA; Department of Radiology, University of Iowa, Iowa City, IA, USA; Department of Radiology, College of Medicine, Seoul National University, Seoul, Republic of Korea; Mailman School of Public Health, Columbia University, New York, NY, USA; Department of Medicine, The University of Arizona, Tucson, AZ, USA; Department of Physiology, UCLA, Los Angeles, CA, USA; Department of Biostatistics, University of North Carolina, Chapel Hill, NC, USA; Department of Internal Medicine, University of Michigan, Ann Arbor, MI, USA; School of Medicine, Johns Hopkins, Baltimore, MD, USA; School of Medicine, University of Utah, Salt Lake City, UT, USA; Department of Radiology, University of Michigan, Ann Arbor, MI, USA; Department of Medicine, UCLA, Los Angeles, CA, USA; Weill Cornell Medicine, Cornell University, New York, NY, USA; School of Medicine, University of North Carolina, Chapel Hill, NC, USA; Department of Internal Medicine, University of Nebraska College of Medicine, Omaha, NE, USA; Department of Medicine, College of Physicians and Surgeons, Columbia University, New York, NY, USA; Department of Medicine, McGill University Health Centre Research Institute, Montreal, Canada; Department of Medicine, University of California at San Francisco, San Francisco, CA, USA Purpose: Quantitative computed tomography (qCT) imaging-based cluster analysis identified clinically meaningful COPD former-smoker subgroups (clusters) based on cross-sectional data. We aimed to identify progression clusters for former smokers using longitudinal data. Patients and Methods: We selected 472 former smokers from SPIROMICS with a baseline visit and a one-year follow-up visit. A total of 150 qCT imaging-based variables, comprising 75 variables at baseline and their corresponding progression rates, were derived from the respective inspiration and expiration scans of the two visits. The COPD progression clusters identified were then associated with subject demography, clinical variables and biomarkers. Results: COPD severities at baseline increased with increasing cluster number. Cluster 1 patients were an obese subgroup with rapid progression of functional small airway disease percentage (fSAD%) and emphysema percentage (Emph%). Cluster 2 exhibited a decrease of fSAD% and Emph%, an increase of tissue fraction at total lung capacity and airway narrowing over one year. Cluster 3 showed rapid expansion of Emph% and an attenuation of fSAD%. Cluster 4 demonstrated severe emphysema and fSAD and significant structural alterations at baseline with rapid progression of fSAD% over one year. Subjects with different progression patterns in the same cross-sectional cluster were identified by longitudinal clustering. Conclusion: qCT imaging-based metrics at two visits for former smokers allow for the derivation of four statistically stable clusters associated with unique progression patterns and clinical characteristics. Use of baseline variables and their progression rates enables identification of longitudinal clusters, resulting in a refinement of cross-sectional clusters.
MoreTranslated text
AI Read Science
Must-Reading Tree
Example
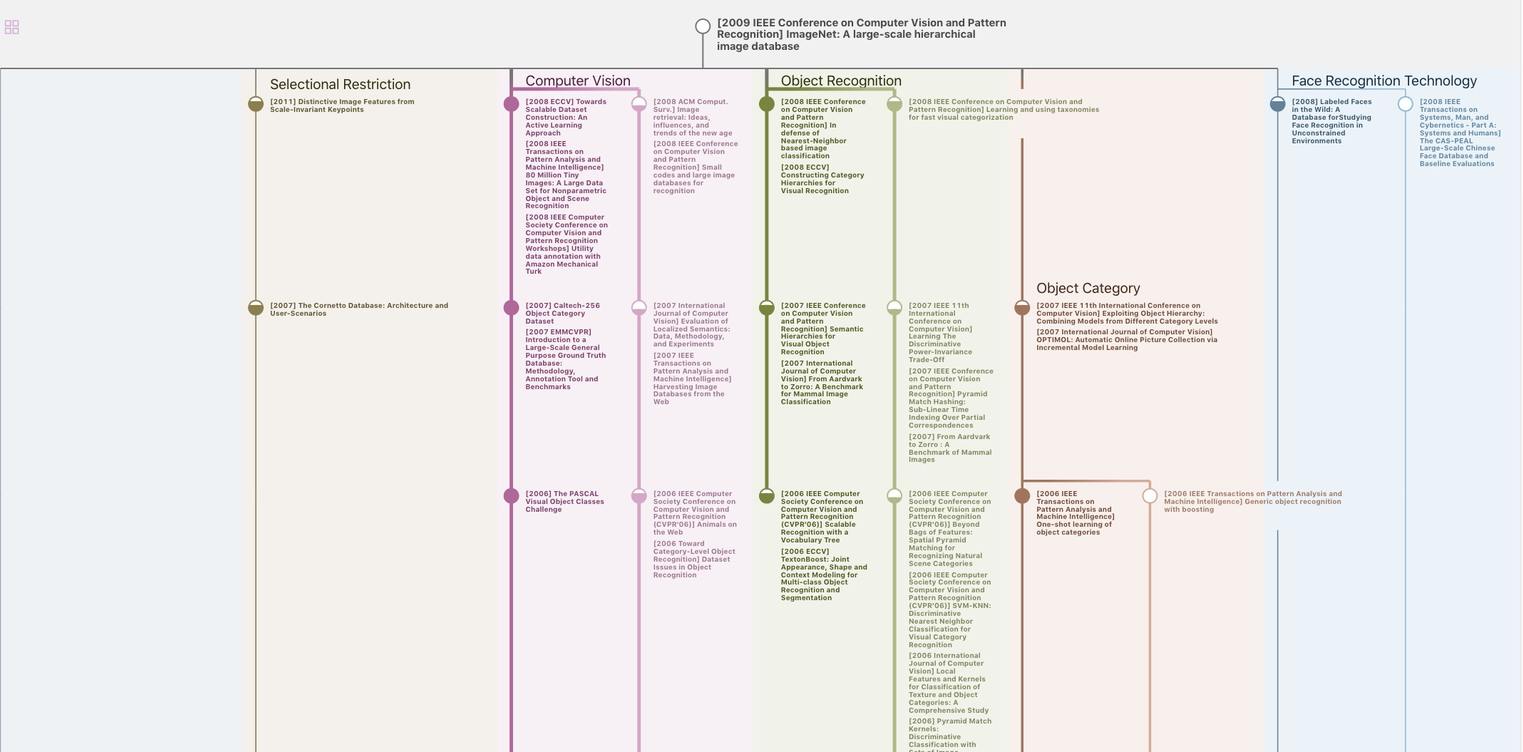
Generate MRT to find the research sequence of this paper
Chat Paper
Summary is being generated by the instructions you defined