Cluster-Based Inference for Memory-Based Cognition
bioRxiv(2022)
摘要
This paper proposes a model of hippocampal-based category learning using a cluster-based inference framework which produces two systems (i) an extendable cluster-based memory module (CMM) that provides representations of learning episodes with strong pattern separation, and supports online decision making during that learning, (ii) cluster-based task modules (CTMs) which consolidate clusters learnt by CMM to support higher-accuracy decision making in the mid-term. Learning in CMMs optimises the joint probability of stimuli and category labels, whereas learning in CTMs optimises the probability of categories given stimuli. The latter system learns from the former via a process of “cluster consolidation”. We apply the model to data from a behavioral learning task and find that, as well as improving categorisation performance, cluster consolidation decreases recognition scores for old cues but increases them for novel cues. This model-based perspective explains forgetting and false memory effects as serving future categorisation performance. The paper also expresses a view that models of memorybased cognition should provide human-level performance on complex categorisation tasks, and do so with minimal labelled data. In working toward this goal we therefore compared the accuracy of CMM- and CTM-based decision making to standard Softmax Regression approaches on benchmark machine learning datasets. This produced mixed results. We found some significant improvements of CMM over Softmax Regression and of CTM over CMM. Overall, our framework reconciles complementary learning systems theory with more recent findings from cognitive neuroscience of generative replay and hippocampal-based generalisation.
### Competing Interest Statement
The authors have declared no competing interest.
更多查看译文
关键词
cognition,inference,cluster-based,memory-based
AI 理解论文
溯源树
样例
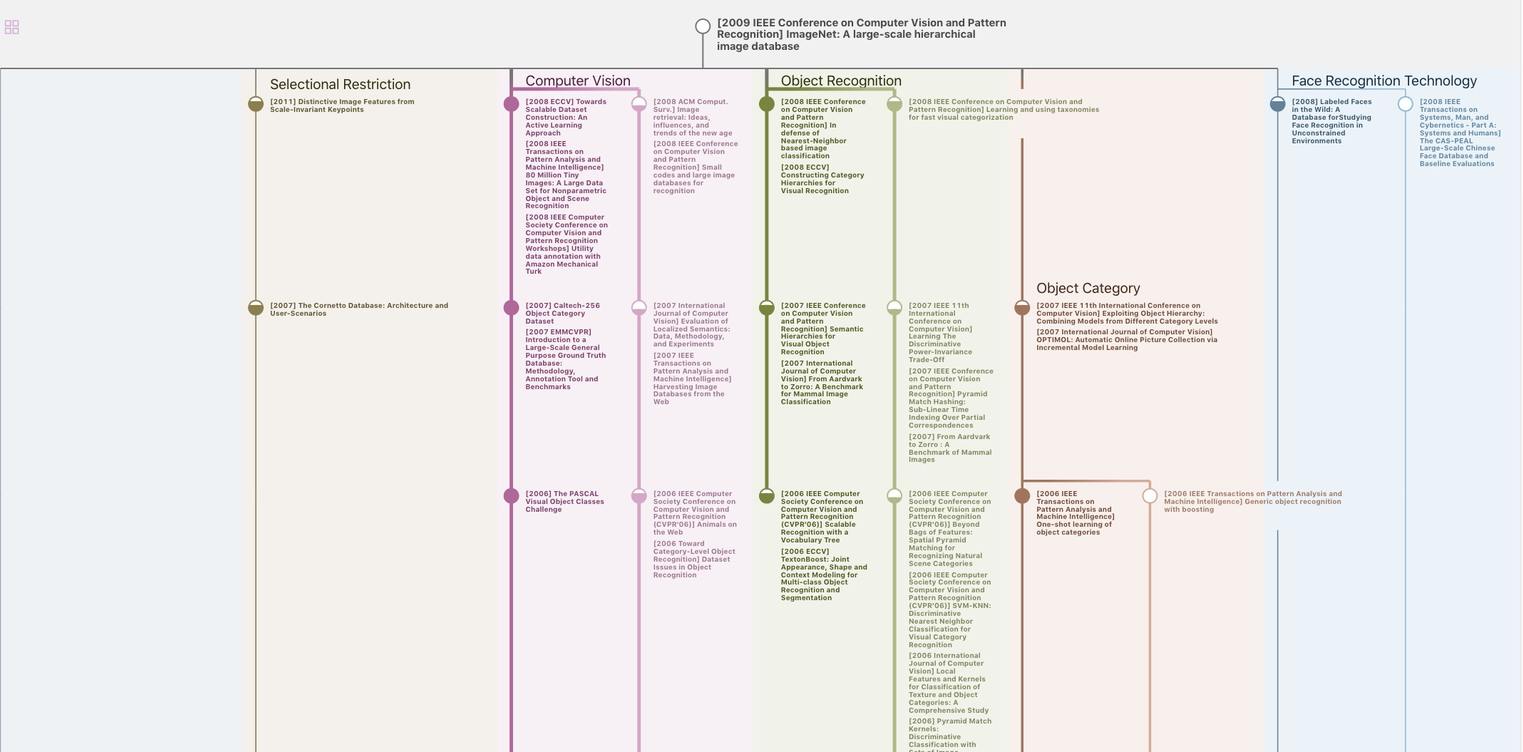
生成溯源树,研究论文发展脉络
Chat Paper
正在生成论文摘要