EDEN: Communication-Efficient and Robust Distributed Mean Estimation for Federated Learning.
International Conference on Machine Learning(2022)
摘要
Distributed Mean Estimation (DME) is a central building block in federated learning, where clients send local gradients to a parameter server for averaging and updating the model. Due to communication constraints, clients often use lossy compression techniques to compress the gradients, resulting in estimation inaccuracies. DME is more challenging when clients have diverse network conditions, such as constrained communication budgets and packet losses. In such settings, DME techniques often incur a significant increase in the estimation error leading to degraded learning performance. In this work, we propose a robust DME technique named EDEN that naturally handles heterogeneous communication budgets and packet losses. We derive appealing theoretical guarantees for EDEN and evaluate it empirically. Our results demonstrate that EDEN consistently improves over state-of-the-art DME techniques.
更多查看译文
关键词
federated learning,mean estimation,communication-efficient
AI 理解论文
溯源树
样例
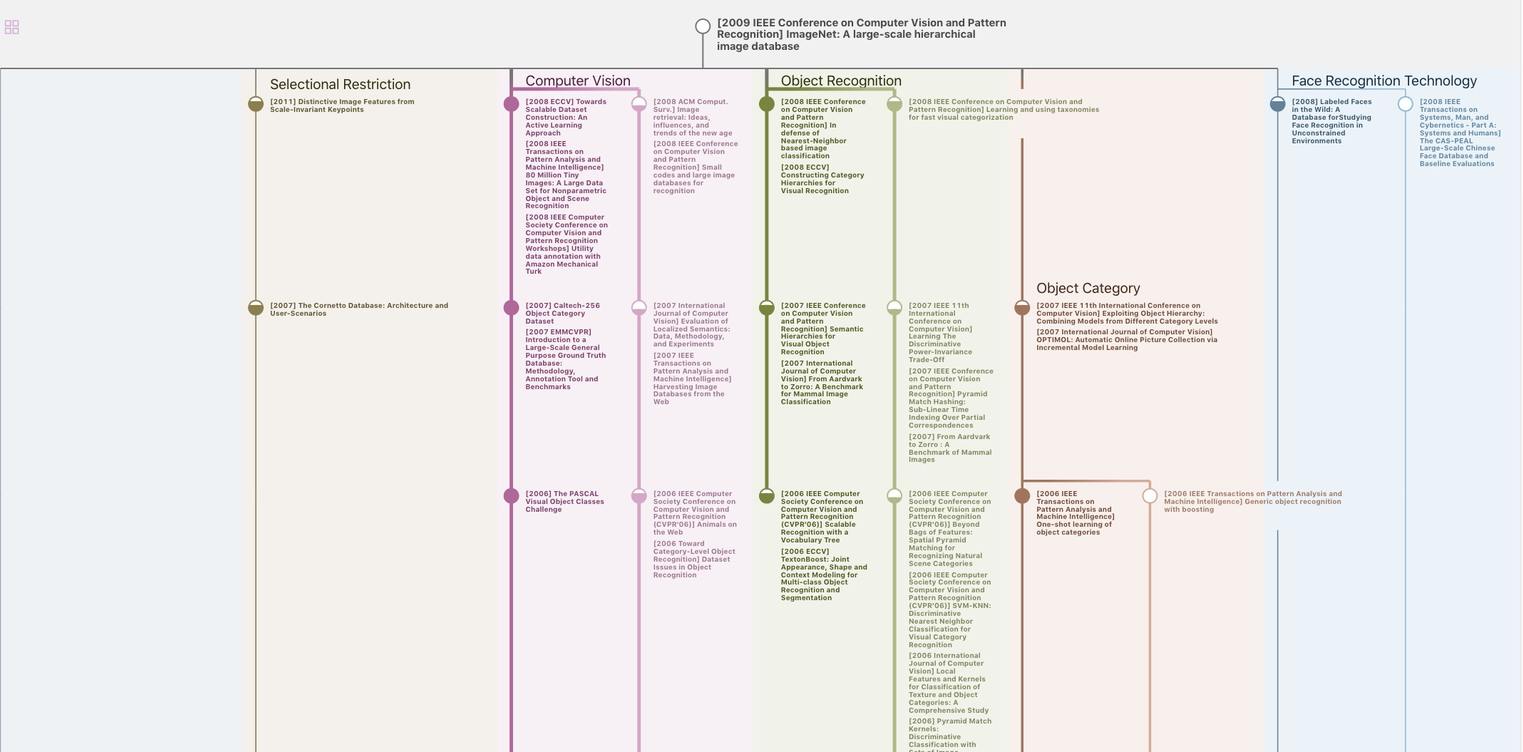
生成溯源树,研究论文发展脉络
Chat Paper
正在生成论文摘要