Improving adaptability to new environments and removing catastrophic forgetting in Reinforcement Learning by using an eco-system of agents
ArXiv(2022)
摘要
Adapting a Reinforcement Learning (RL) agent to an unseen environment is a difficult task due to typ-ical over-fitting on the training environment. RL agents are often capable of solving environments very close to the trained environment, but when environments become substantially different, their performance quickly drops. When agents are retrained on new environments, a second issue arises: there is a risk of catastrophic forgetting, where the performance on previously seen environments is seriously hampered. This paper proposes a novel approach that exploits an ecosystem of agents to address both concerns. Hereby, the (limited) adaptive power of individual agents is harvested to build a highly adaptive ecosystem. This allows to trans-fer part of the workload from learning to inference. An evaluation of the approach on two distinct dis-tributions of environments shows that our approach outperforms state-of-the-art techniques in terms of adaptability/generalization as well as avoids catastrophic forgetting.
更多查看译文
AI 理解论文
溯源树
样例
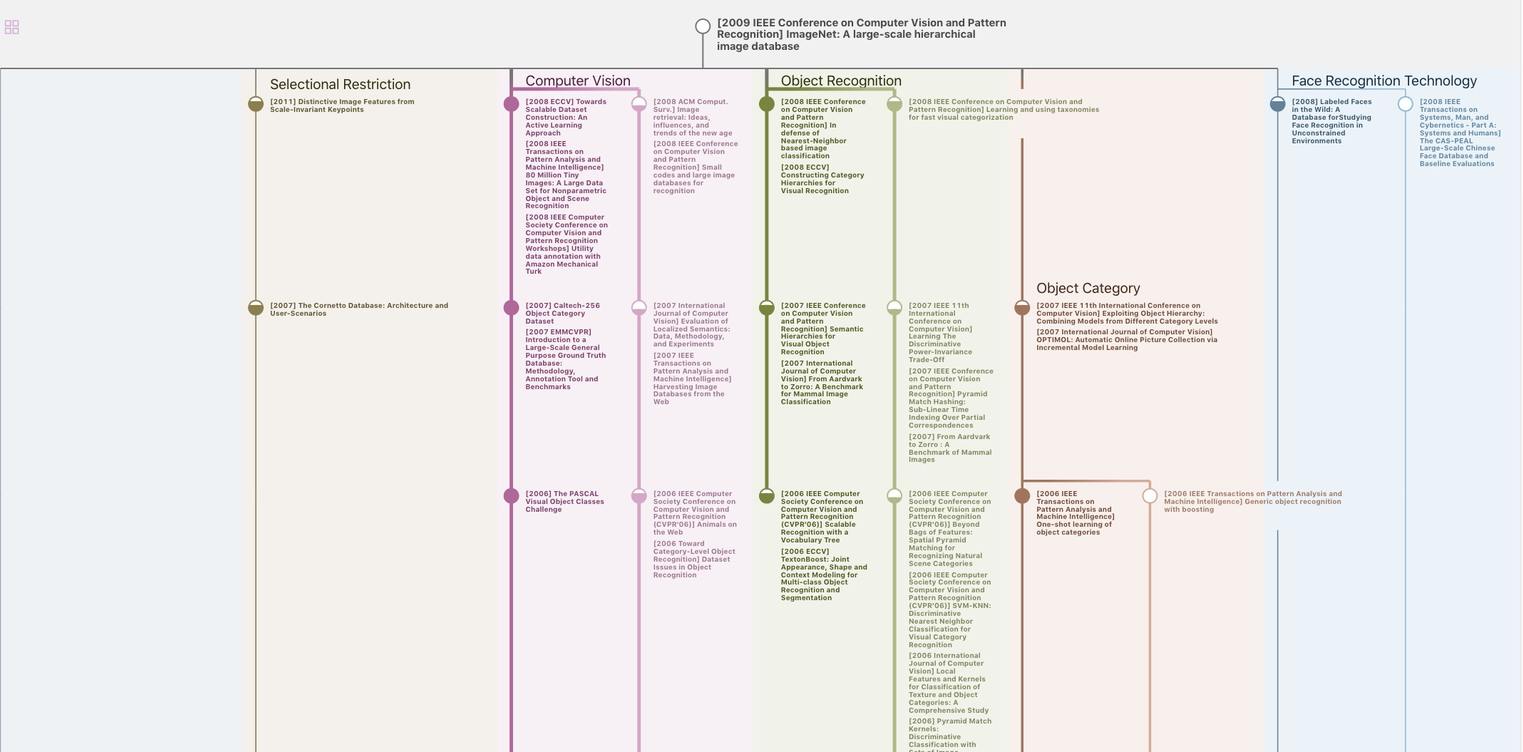
生成溯源树,研究论文发展脉络
Chat Paper
正在生成论文摘要