ELODI: Ensemble Logit Difference Inhibition for Positive-Congruent Training.
IEEE transactions on pattern analysis and machine intelligence(2024)
摘要
Negative flips are errors introduced in a classification system when a legacy model is updated. Existing methods to reduce the negative flip rate (NFR) either do so at the expense of overall accuracy by forcing a new model to imitate the old models, or use ensembles, which multiply inference cost prohibitively. We analyze the role of ensembles in reducing NFR and observe that they remove negative flips that are typically not close to the decision boundary, but often exhibit large deviations in the distance among their logits. Based on the observation, we present a method, called Ensemble Logit Difference Inhibition (ELODI), to train a classification system that achieves paragon performance in both error rate and NFR, at the inference cost of a single model. The method distills a homogeneous ensemble to a single student model which is used to update the classification system. ELODI also introduces a generalized distillation objective, Logit Difference Inhibition (LDI), which only penalizes the logit difference of a subset of classes with the highest logit values. On multiple image classification benchmarks, model updates with ELODI demonstrate superior accuracy retention and NFR reduction.
更多查看译文
关键词
Cross-model compatibility,ensemble learning,positive-congruent training
AI 理解论文
溯源树
样例
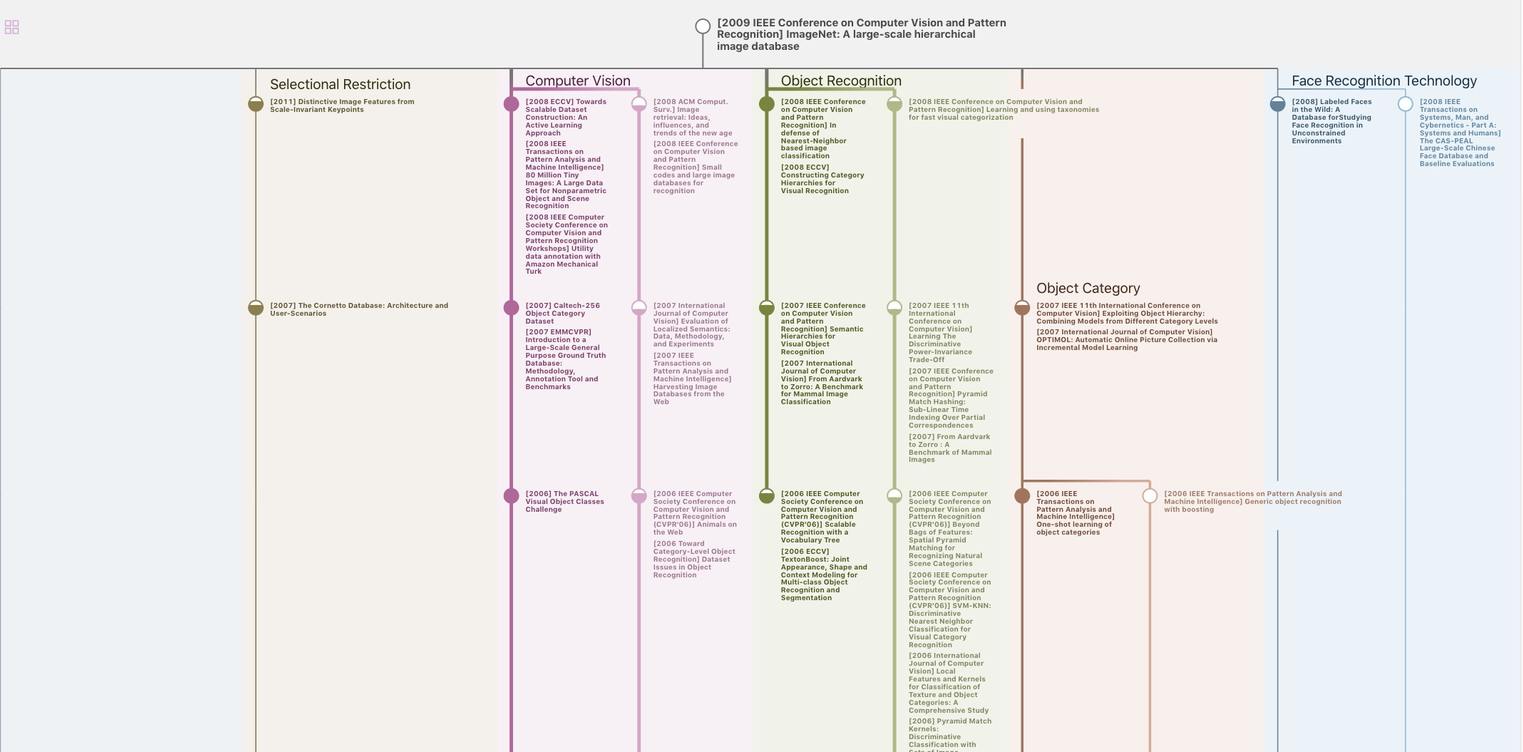
生成溯源树,研究论文发展脉络
Chat Paper
正在生成论文摘要