Learning Discrete Neural Reaction Class to Improve Retrosynthesis Prediction
semanticscholar(2021)
Abstract
Computer-aided retrosynthesis accelerate and innovate the process of molecule and material design, allowing the discovery of new pathways and automating part of the overall development process for drugs and materials. Current machinelearning methods applied to retrosynthesis are limited by their lack of control when generating single-step reactions as they rely on sampling or beam search algorithm. In this work, we apply vector quantized representation learning [1] to learn reaction classes along with retrosynthetic predictions. We represent each reaction class with a vector allowing us to condition the retrosynthetic prediction. We show that learning reaction classes increases control as well as generating more diverse predictions than a baseline model. Our results are a significant step forward in the development of multistep retrosynthesis prediction.
MoreTranslated text
AI Read Science
Must-Reading Tree
Example
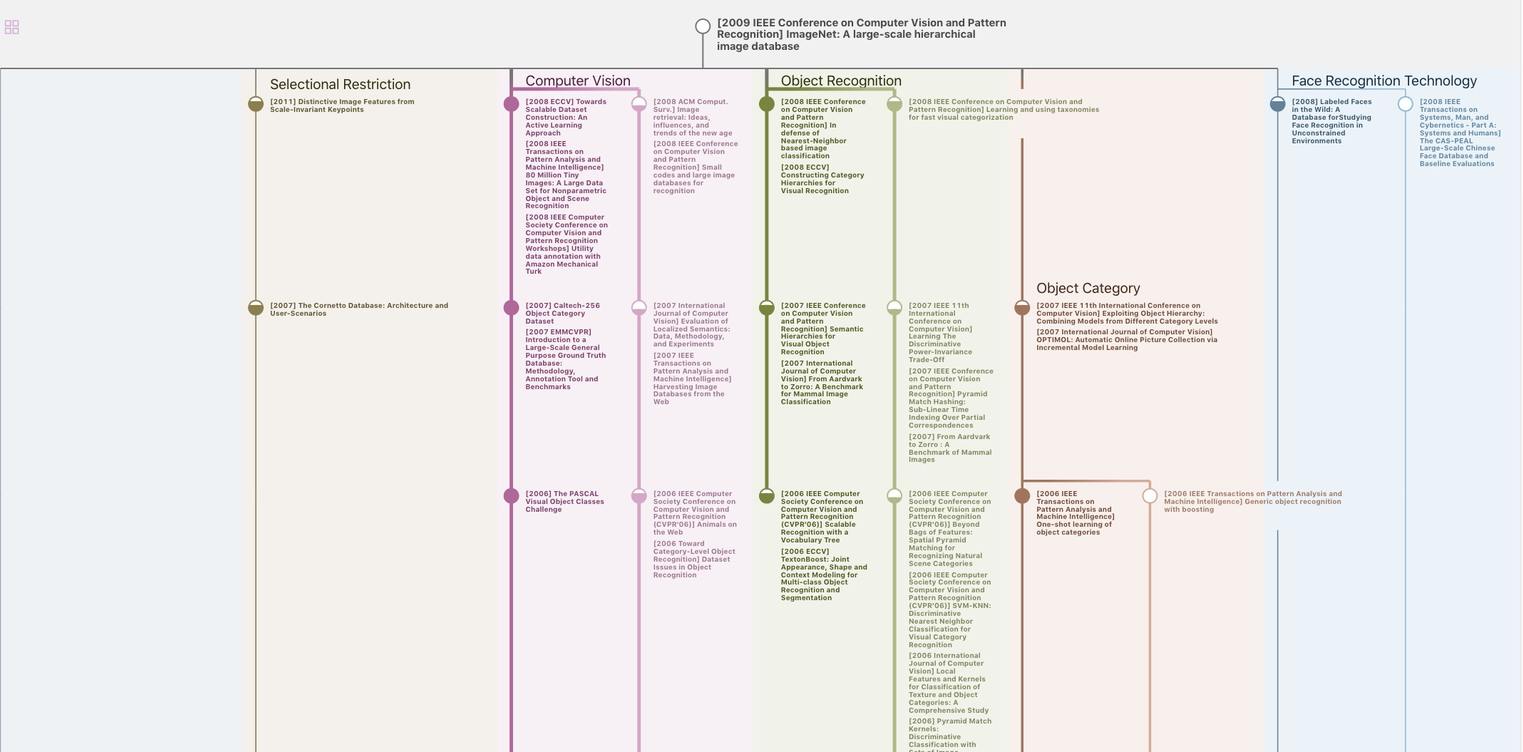
Generate MRT to find the research sequence of this paper
Chat Paper
Summary is being generated by the instructions you defined