Deep Learning for the Analysis of Optical Maps of CVD-grown TMDs
semanticscholar(2021)
摘要
The study of atomically thin transitional metal dichalcogenides (TMDs) is a new and vibrant research field. Due to their advantageous electronic and optical properties, these materials show great promise for use in electronic and photonic devices. In particular, chemical vapor deposition (CVD) synthesis has a good potential to grow large and uniform monolayer islands, while still exhibiting sufficient crystallinity. However, synthesis does not typically produce homogenous distribution of island shapes and properties with respect to lateral position on the growth substrate. To tackle this problem, we propose a low-cost upgrade to existing optical microscope used to inspect synthesized samples. The upgrade consists of an x-y motorized stage based on the Arduino microcontroller and MARLIN, an open-source software for 3D printers, as well as the Raspberry Pi and camera for image acquisition. This allows us to acquire high magnification optical image maps and extract distribution maps of different features over the whole substrate area. Typically, in data analysis of 2D samples, processing is done on a few representative areas. However, in recent years, different machine learning algorithms are employed to map over the entire substrate area in setups similar to ours. However, to the best of our knowledge, all previous work is mainly focused on exfoliated samples of 2D materials in an effort to extract the number of layers and size of the exfoliated flakes. In this work, we use CVD grown crystals of different TMDs and employ neural networks for feature extraction. We employ a YOLO-like architecture to localize and assess the size of crystal islands, allowing us to get a lateral distribution of island density and island size for each grown sample. We also employ a modified UNET neural network with adversarial augmentation for segmentation of material from the substrate area to extract the distribution of material coverage. We anticipate our work will extend this principle to other mapping techniques such as AFM, SEM and Raman microscopy. Furthermore, we plan to extract maps of morphological information from individual islands. Using this information, we can correlate CVD parameters to different growth mechanisms and optimise the synthesis to be more homogeneous, while retaining good quality of the samples.
更多查看译文
AI 理解论文
溯源树
样例
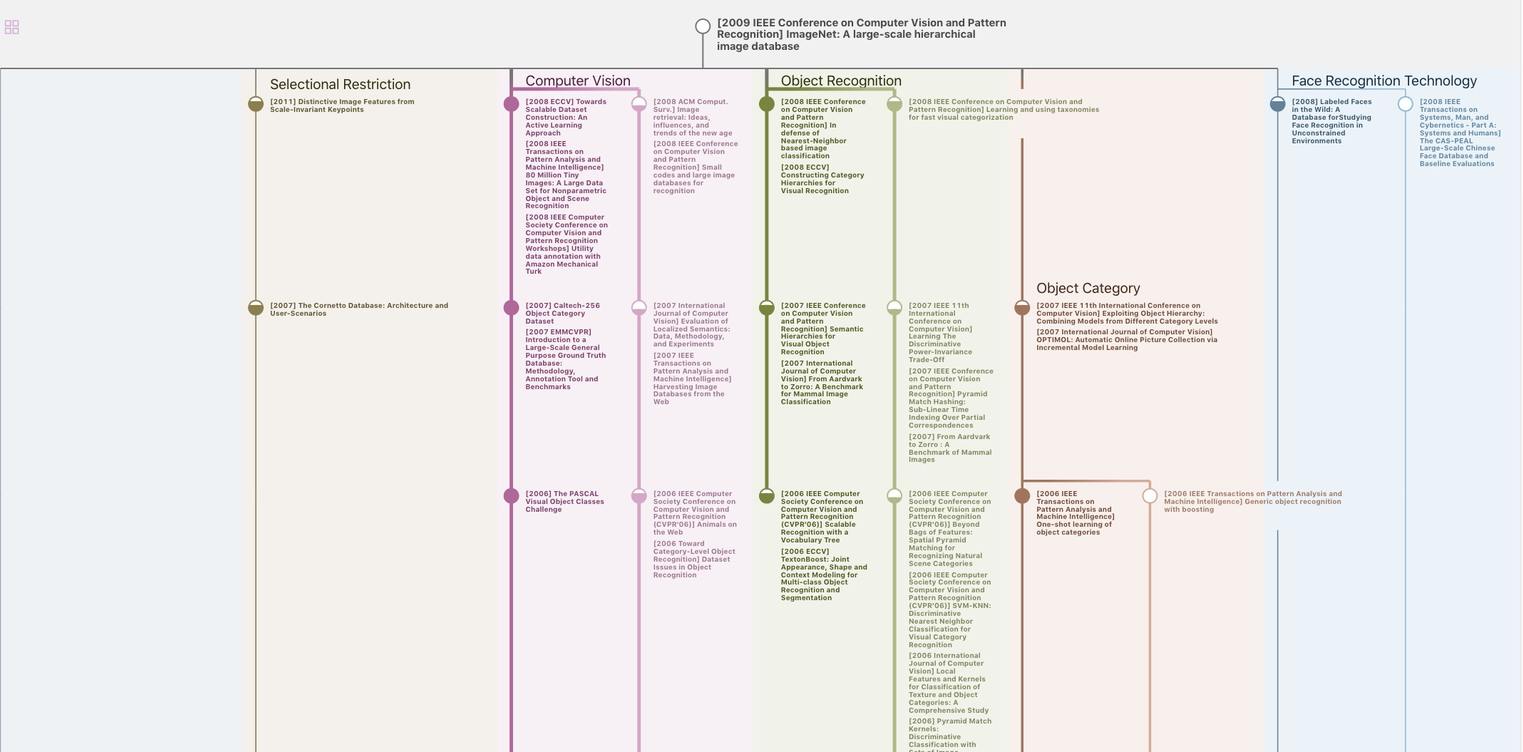
生成溯源树,研究论文发展脉络
Chat Paper
正在生成论文摘要