COVID-19 Prognostic Modeling Using CT Radiomic Features and Machine Learning Algorithms: Analysis of a Multi-Institutional Dataset of 14,339 Patients
medRxiv(2021)
摘要
Objective: In this large multi-institutional study, we aimed to analyze the prognostic power of computed tomography (CT)-based radiomics models in COVID-19 patients. Methods: CT images of 14,339 COVID-19 patients with overall survival outcome were collected from 19 medical centers. Whole lung segmentations were performed automatically using a previously validated deep learning-based model, and regions of interest were further evaluated and modified by a human observer. All images were resampled to an isotropic voxel size, intensities were discretized into 64-binning size, and 105 radiomics features, including shape, intensity, and texture features were extracted from the lung mask. Radiomics features were normalized using Z-score normalization. High-correlated features using Pearson (R2>0.99) were eliminated. We applied the Synthetic Minority Oversampling Technique (SMOT) algorithm in only the training set for different models to overcome unbalance classes. We used 4 feature selection algorithms, namely Analysis of Variance (ANOVA), Kruskal-Wallis (KW), Recursive Feature Elimination (RFE), and Relief. For the classification task, we used seven classifiers, including Logistic Regression (LR), Least Absolute Shrinkage and Selection Operator (LASSO), Linear Discriminant Analysis (LDA), Random Forest (RF), AdaBoost (AB), Naive Bayes (NB), and Multilayer Perceptron (MLP). The models were built and evaluated using training and testing sets, respectively. Specifically, we evaluated the models using 10 different splitting and cross-validation strategies, including different types of test datasets (e.g. non-harmonized vs. ComBat-harmonized datasets). The sensitivity, specificity, and area under the receiver operating characteristic (ROC) curve (AUC) were reported for models evaluation. Results: In the test dataset (4301) consisting of CT and/or RT-PCR positive cases, AUC, sensitivity, and specificity of 0.83(sd:0.01) (CI95%: 0.81-0.85), 0.81, and 0.72, respectively, were obtained by ANOVA feature selector + RF classifier. In RT-PCR-only positive test sets (3644), similar results were achieved, and there was no statistically significant difference. In ComBat harmonized dataset, Relief feature selector + RF classifier resulted in highest performance of AUC, reaching 0.83 (sd:0.01) (CI95%: 0.81-0.85), with sensitivity and specificity of 0.77 and 0.74, respectively. At the same time, ComBat harmonization did not depict statistically significant improvement relevant to non-harmonized dataset. In leave-one-center-out, the combination of ANOVA feature selector and LR classifier resulted in the highest performance of AUC (0.80 (sd:0.084)) with sensitivity and specificity of 0.77 (sd:0.11) and 0.76 (sd: 0.075), respectively. Conclusion: Lung CT radiomics features can be used towards robust prognostic modeling of COVID-19 in large heterogeneous datasets gathered from multiple centers. As such, CT radiomics-based model has significant potential for use in prospective clinical settings towards improved management of COVID-19 patients.
更多查看译文
关键词
ct radiomic features,machine learning algorithms,patients,multi-institutional
AI 理解论文
溯源树
样例
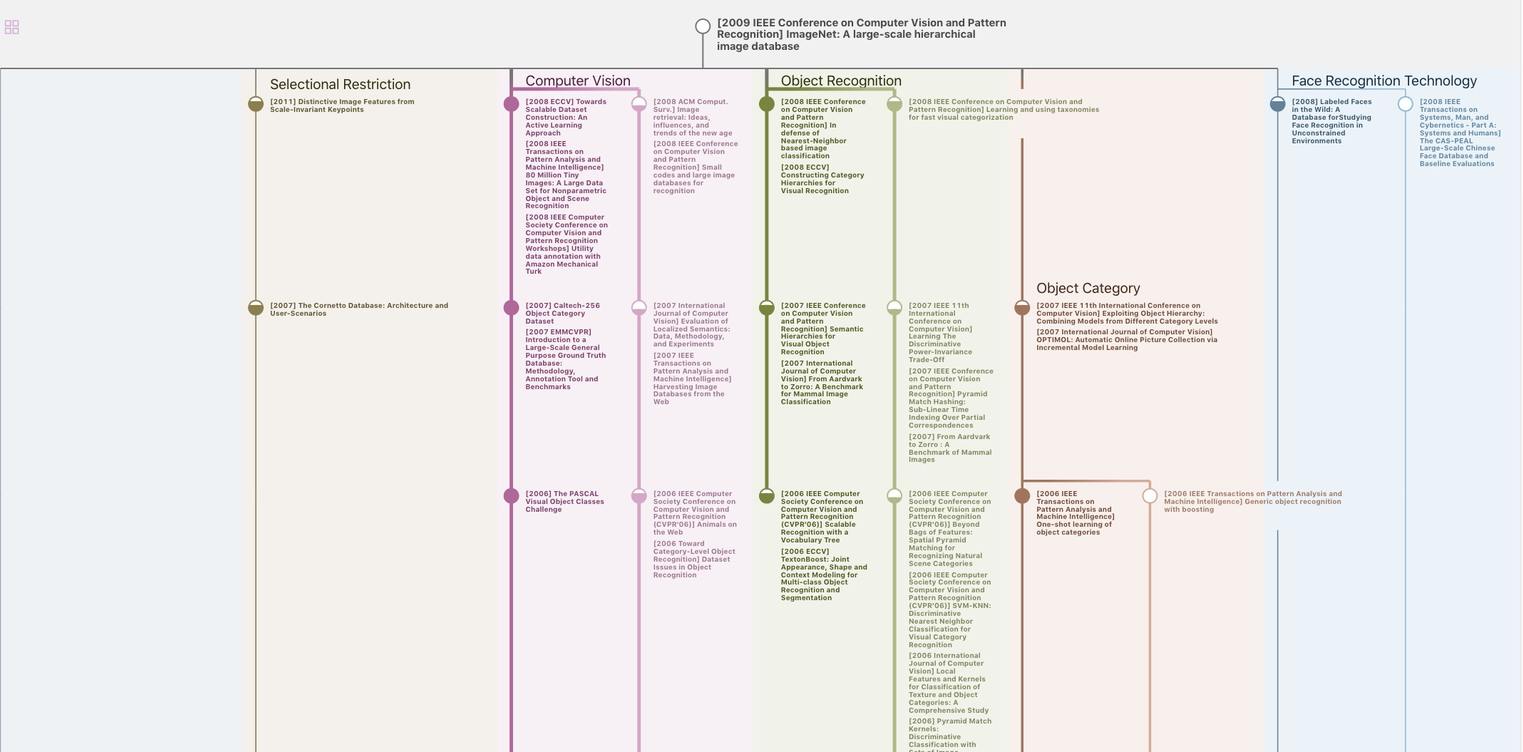
生成溯源树,研究论文发展脉络
Chat Paper
正在生成论文摘要