From Handheld to Unconstrained Object Detection: a Weakly-supervised On-line Learning Approach
2022 31st IEEE International Conference on Robot and Human Interactive Communication (RO-MAN)(2022)
摘要
Deep Learning (DL) based methods for object detection achieve remarkable performance at the cost of computationally expensive training and extensive data labeling. Robots embodiment can be exploited to mitigate this burden by acquiring automatically annotated training data via a natural interaction with a human showing the object of interest, hand-held. However, learning solely from this data may introduce biases (the so-called domain shift), and prevents adaptation to novel tasks. While Weakly-supervised Learning offers a well-established set of techniques to cope with these problems in general-purpose Computer Vision, its adoption in challenging robotic domains is still at a preliminary stage. In this work, we target the scenario of a robot trained in a teacher-learner setting to detect handheld objects. The aim is to improve detection performance in different settings by letting the robot explore the environment with a limited human labeling budget. We compare several techniques for WSL in detection pipelines to reduce model re-training costs without compromising accuracy, proposing solutions which target the considered robotic scenario. We show that the robot can improve adaptation to novel domains, either by interacting with a human teacher (Active Learning) or with an autonomous supervision (Semi-supervised Learning). We integrate our strategies into an on-line detection method, achieving efficient model update capabilities with few labels. We experimentally benchmark our method on challenging robotic object detection tasks under domain shift
1
.
更多查看译文
关键词
unconstrained object detection,handheld,weakly-supervised,on-line
AI 理解论文
溯源树
样例
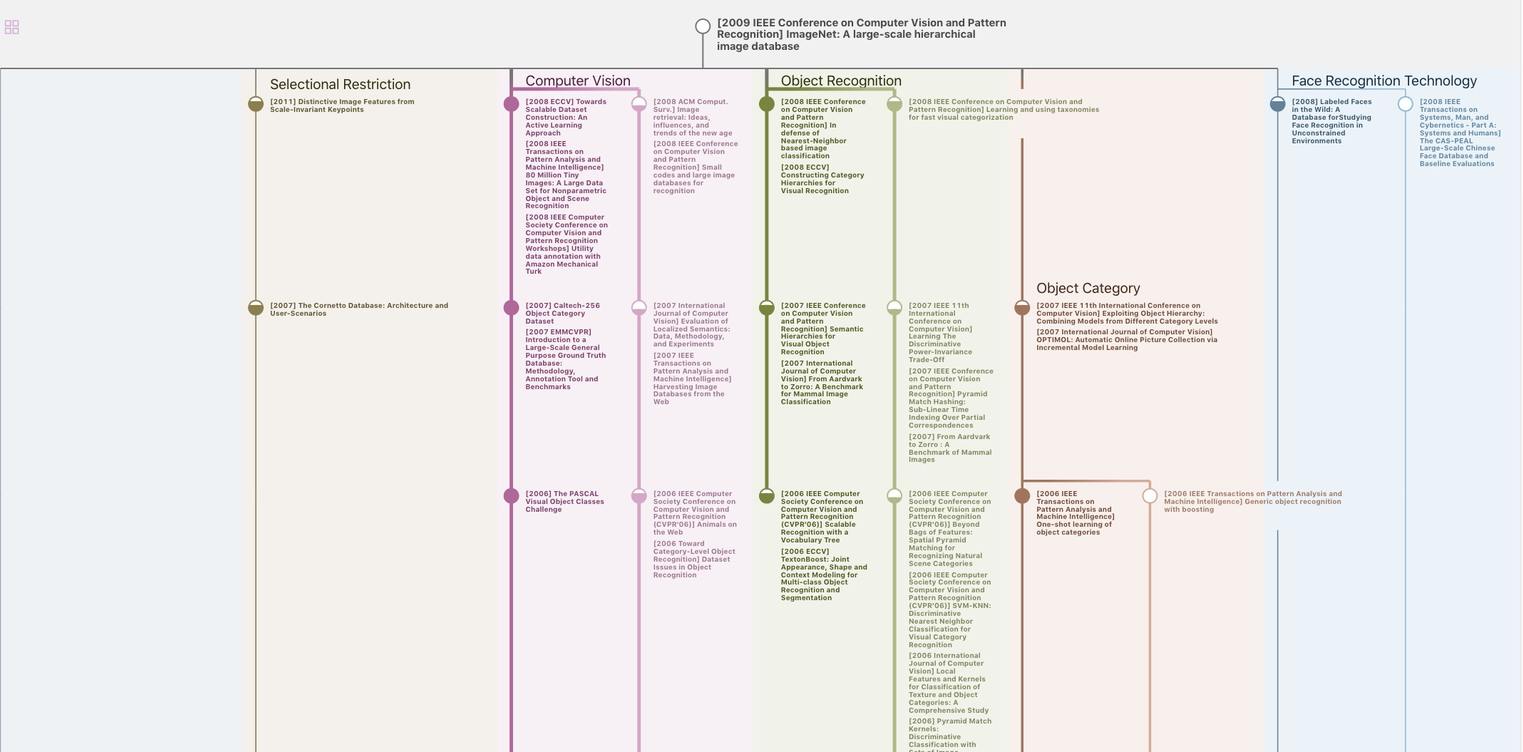
生成溯源树,研究论文发展脉络
Chat Paper
正在生成论文摘要