Quantitative Uncertainty Assessment of Lumen Detection in Intravascular Ultrasound Images Using Deep Ensembles Deep Ensembles を用いた血管内超音波画像の血管内腔検出に おける不確実性の定量評価
semanticscholar(2021)
摘要
Intravascular ultrasound (IVUS) and the fractional flow reserve (FFR) tests are necessary examinations that assess detailed structures and hemodynamics of the pathological coronary artery and provide useful information to determine the necessity of the invasive percutaneous coronary intervention (PCI) treatment. Since the FFR test requires inserting pressure sensors into the stenosed artery and administrating drugs to induce cardiac stress, alternative methods that can estimate FFR index without those cumbersome techniques have been studied. One of which is using IVUS images to extract changes in diameter around the pathological artery and estimating the FFR index from those geometrical features. The major problem of the method is that physicians must manually trace the arterial wall from consequent images as it is demanding and sometimes impossible procedure because the boundary is often unclear or invisible in IVUS images. As a solution, Deep Learning models to automatically trace the vessel wall has been reported. However, the Deep Learning techniques have a problem called overconfidence, where the estimated probability in classification problems is biased towards 0 and 1, and the estimated probability value does not represent its uncertainty. Therefore, the reliability of each extracted boundary has not been considered. This study aimed to develop a machine-learning based method for both detecting the lumen of the coronary artery and quantifying its uncertainty using Deep Ensembles algorithm.
更多查看译文
AI 理解论文
溯源树
样例
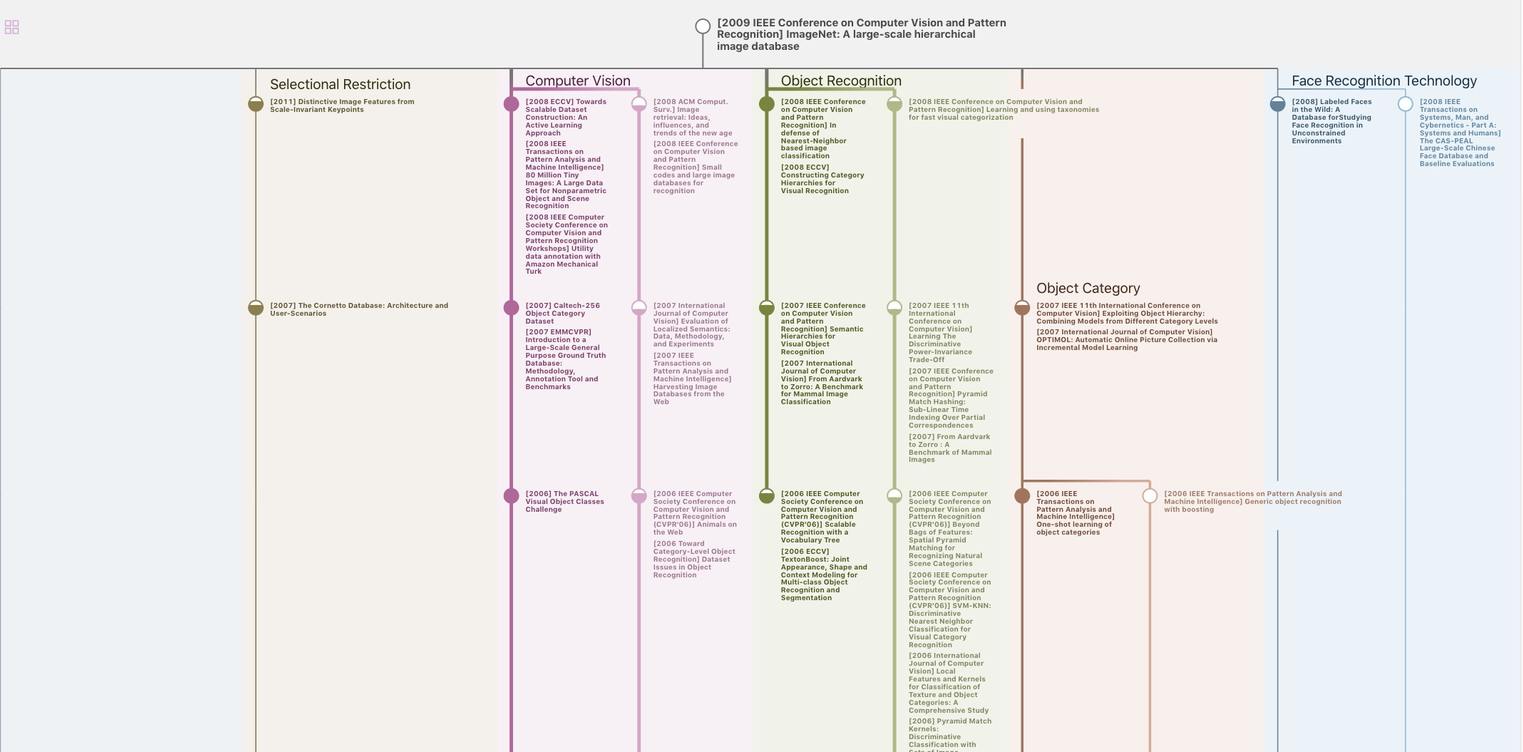
生成溯源树,研究论文发展脉络
Chat Paper
正在生成论文摘要