A Neural Network Approach for Real-Time High-Dimensional Optimal Control
semanticscholar(2021)
摘要
We propose a neural network approach for solving high-dimensional optimal control problems arising in real-time applications. Our approach yields controls in a feedback form, where the policy function is given by a neural network (NN). Specifically, we fuse the HamiltonJacobi-Bellman (HJB) and Pontryagin Maximum Principle (PMP) approaches by parameterizing the value function with an NN. We can therefore synthesize controls in realtime without having to solve an optimization problem. Once the policy function is trained, generating a control at a given space-time location takes milliseconds; in contrast, efficient nonlinear programming methods typically perform the same task in seconds. We train the NN offline using the objective function of the control problem and penalty terms that enforce the HJB equations. Therefore, our training algorithm does not involve data generated by another algorithm. By training on a distribution of initial states, we ensure the controls’ optimality on a large portion of the state-space. Our grid-free approach scales efficiently to dimensions where grids become impractical or infeasible. We demonstrate the effectiveness of our approach on several multi-agent collision-avoidance problems in up to 150 dimensions. Furthermore, we empirically observe that the number of parameters in our approach scales linearly with the dimension of the control problem, thereby mitigating the curse of dimensionality.
更多查看译文
AI 理解论文
溯源树
样例
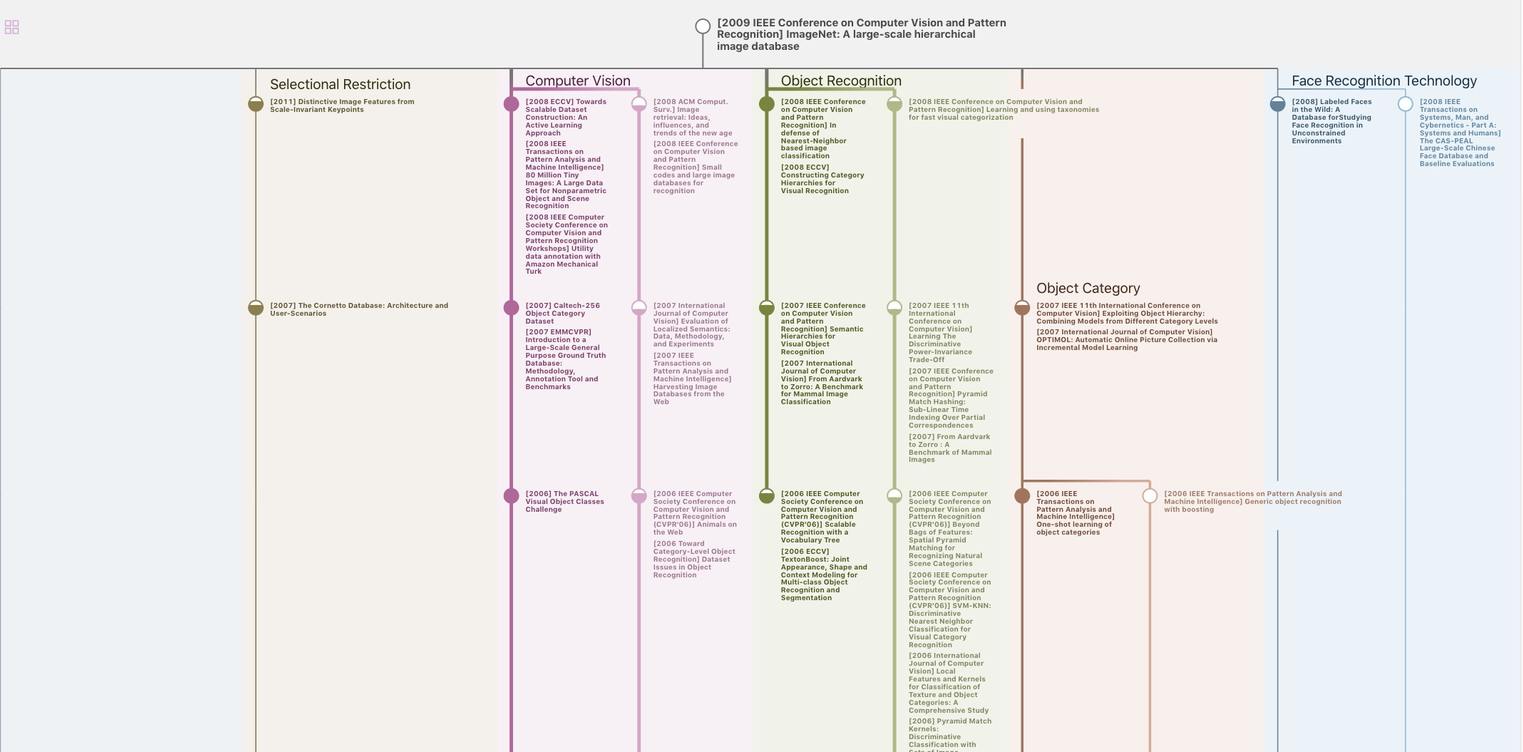
生成溯源树,研究论文发展脉络
Chat Paper
正在生成论文摘要