MMatch: Semi-Supervised Discriminative Representation Learning for Multi-View Classification
IEEE Transactions on Circuits and Systems for Video Technology(2022)
摘要
Semi-supervised multi-view learning has been an important research topic due to its capability to exploit complementary information from unlabeled multi-view data. This work proposes MMatch, a new semi-supervised discriminative representation learning method for multi-view classification. Unlike existing multi-view representation learning methods that seldom consider the negative impact caused by particular views with unclear classification structures (weak discriminative views). MMatch jointly learns view-specific representations and class probabilities of training data. The representations concatenated to integrate multiple views’ information to form a global representation. Moreover, MMatch performs the smoothness constraint on the class probabilities of the global representation to improve pseudo labels, whereas the pseudo labels regularize the structure of view-specific representations. A discriminative global representation is mined with the training process, and the negative impact of weak discriminative views is overcome. Besides, MMatch learns consistent classification while preserving diverse information from multiple views. Experiments on several multi-view datasets demonstrate the effectiveness of MMatch.
更多查看译文
关键词
Semi-supervised learning,multi-view classification,discriminative representation,pseudo-labeling
AI 理解论文
溯源树
样例
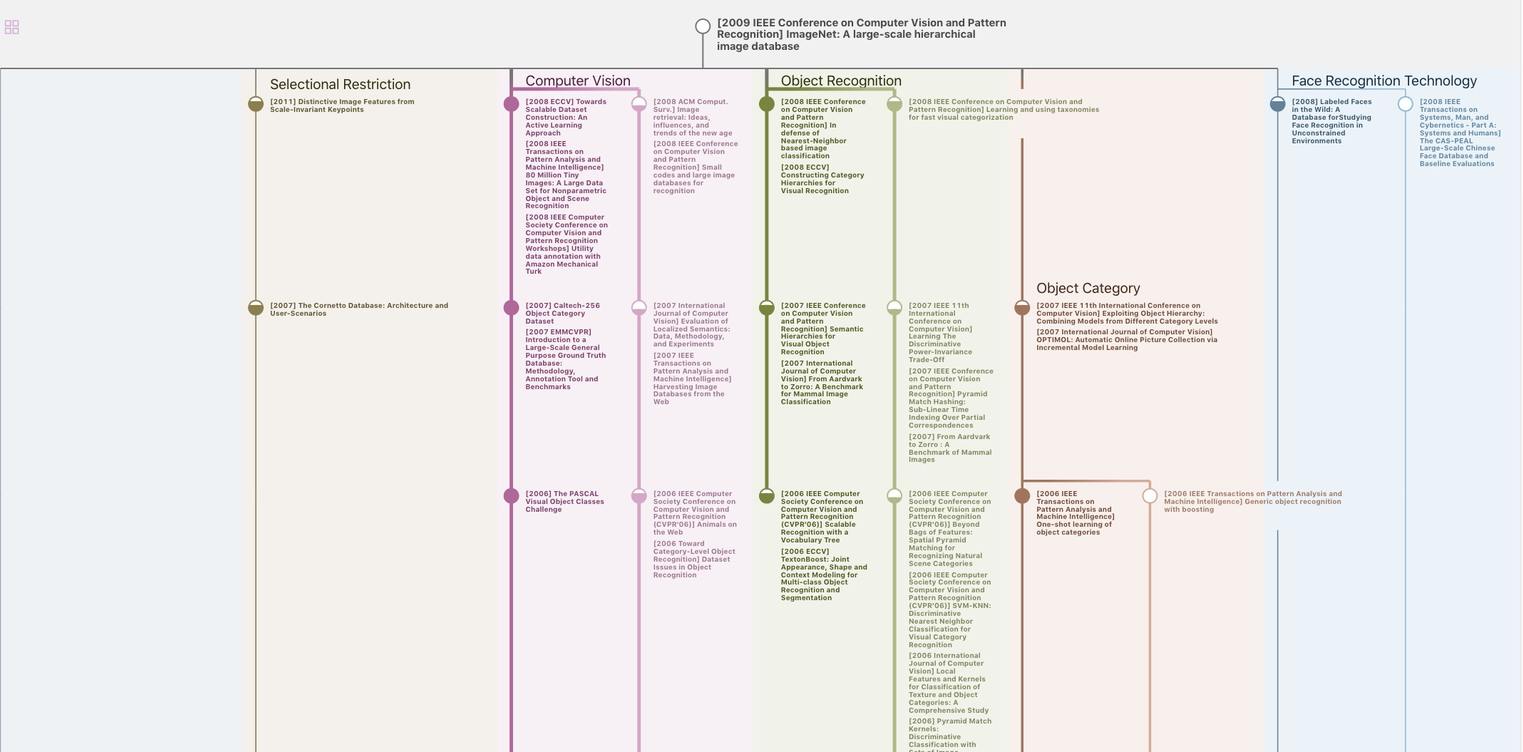
生成溯源树,研究论文发展脉络
Chat Paper
正在生成论文摘要