Analysis on improving the application of machine learning in product development
The Journal of Supercomputing(2022)
摘要
One of the main competitive advantages of an enterprise is its continuous development of new products. The higher the level of product innovation, the more the enterprise is ahead of its competitors. However, products that are more innovative have a higher chance of failure. Traditionally, enterprises use questionnaires, focus groups and customer interviews to understand the expectations and requirements of their customers for specific products; however, if the customers do not fully understand their own needs, they cannot express them fully. Therefore, in order to understand the functional requirements that are expected from their products, some enterprises go directly to the place where their customers are and observe how they use them. Nevertheless, the success rate for new product development is low. To solve this problem, this study uses the machine learning algorithm for the planning and prediction of product R&D. Firstly, the prediction model of a product’s success rate is established by using the random tree algorithm, the bagging algorithm and the SMO algorithm. The model is then used for the planning and prediction of new products. The results show that the model accuracy of the random tree algorithm is 100%, that of the bagging algorithm is 83.33%, and that of the SMO algorithm is 91.67%. The prediction results of the three algorithms are integrated to judge the market’s acceptance of the planned new products. Finally, the new products are improved, and after the improvements have been made, the three algorithms are used to make predictions. As a result, the acceptance of new products by customers has increased significantly. According to this study, machine learning can improve the success rate of new products in the marketplace.
更多查看译文
关键词
Machine learning,Product development,Random tree algorithm,Bagging algorithm,SMO algorithm
AI 理解论文
溯源树
样例
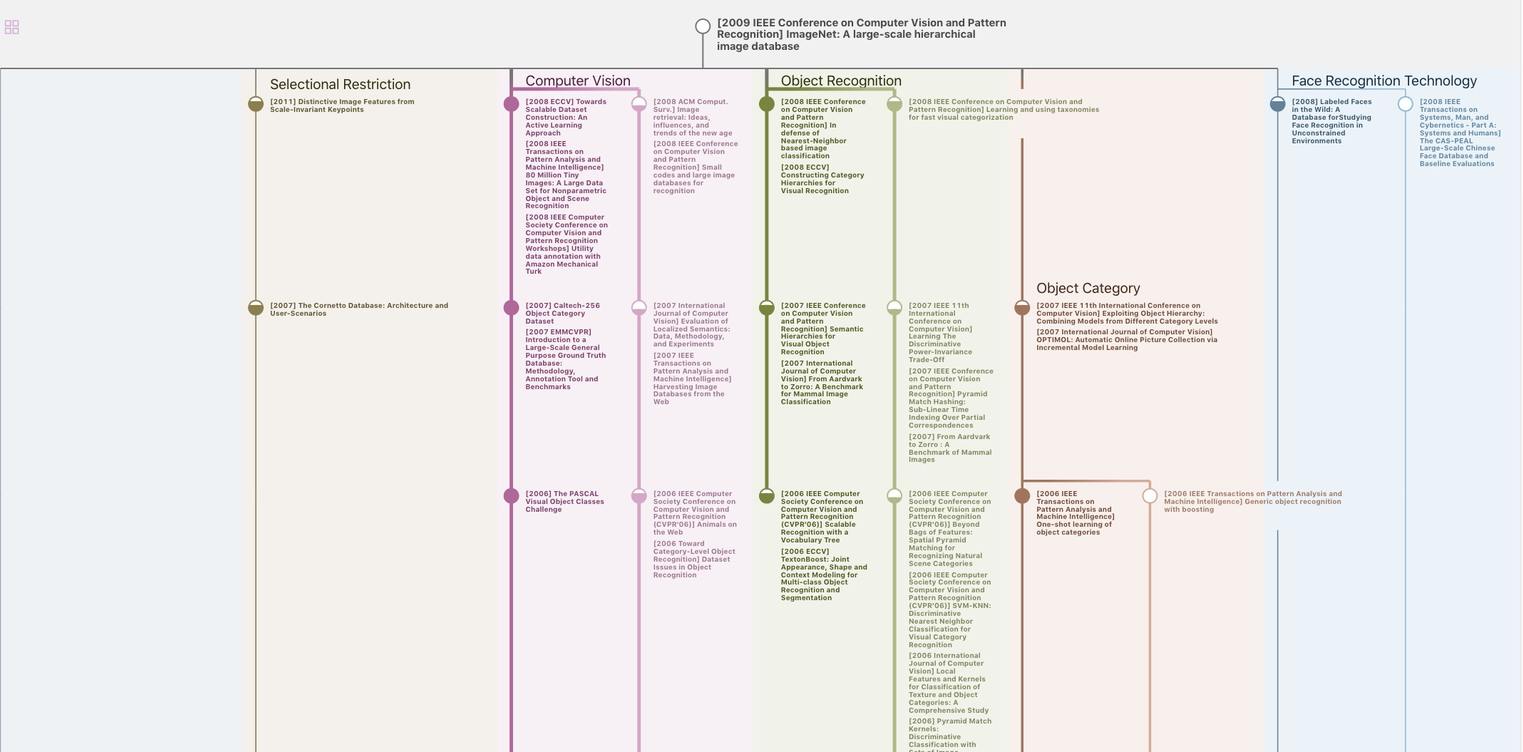
生成溯源树,研究论文发展脉络
Chat Paper
正在生成论文摘要