Deep Learning-Based Abdominal Muscle Segmentation on CT Images of Surgical Patient Populations
MEDICAL IMAGING 2022: BIOMEDICAL APPLICATIONS IN MOLECULAR, STRUCTURAL, AND FUNCTIONAL IMAGING(2022)
摘要
Computed tomography (CT) is commonly used for the characterization and tracking of abdominal muscle mass in surgical patients for both pre-surgical outcome predictions and post-surgical monitoring of response to therapy. In order to accurately track changes of abdominal muscle mass, radiologists must manually segment CT slices of patients, a time-consuming task with potential for variability. In this work, we combined a fully convolutional neural network (CNN) with high levels of preprocessing to improve segmentation quality. We utilized a CNN based approach to remove patients' arms and fat from each slice and then applied a series of registrations with a diverse set of abdominal muscle segmentations to identify a best fit mask. Using this best fit mask, we were able to remove many parts of the abdominal cavity, such as the liver, kidneys, and intestines. This preprocessing was able to achieve a mean Dice similarity coefficient (DSC) of 0.53 on our validation set and 0.50 on our test set by only using traditional computer vision techniques and no artificial intelligence. The preprocessed images were then fed into a similar CNN previously presented in a hybrid computer vision-artificial intelligence approach and was able to achieve a mean DSC of 0.94 on testing data. The preprocessing and deep learning-based method is able to accurately segment and quantify abdominal muscle mass on CT images.
更多查看译文
关键词
Abdominal imaging,machine learning,surgical patient outcomes,CT,convolutional neural network
AI 理解论文
溯源树
样例
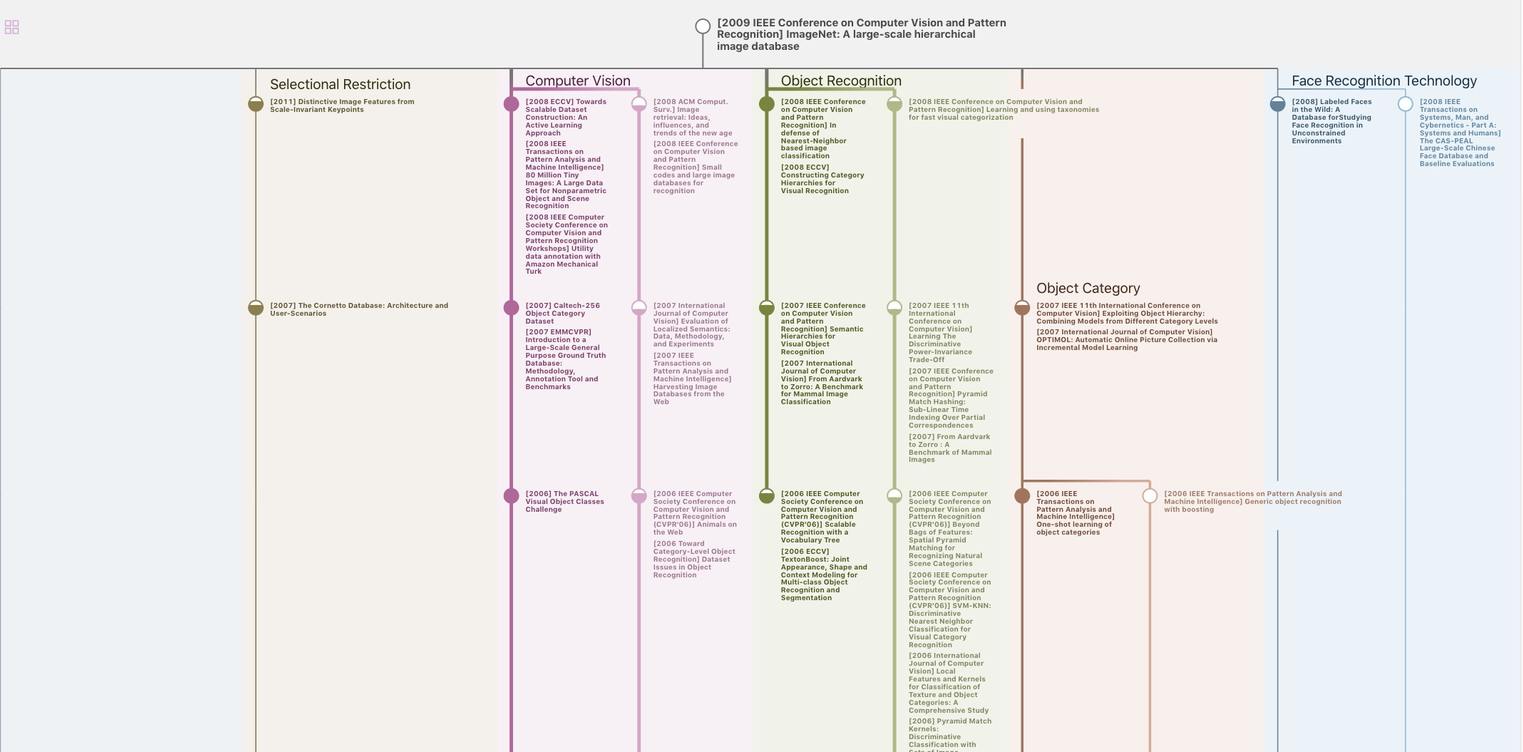
生成溯源树,研究论文发展脉络
Chat Paper
正在生成论文摘要