Risk factors and prognostic nomogram for patients with second primary cancers after lung cancer using classical statistics and machine learning
CLINICAL AND EXPERIMENTAL MEDICINE(2022)
摘要
Previous studies have revealed an increased risk of secondary primary cancers (SPC) after lung cancer. The prognostic prediction models for SPC patients after lung cancer are particularly needed to guide screening. Therefore, we study retrospectively analyzed the Surveillance, Epidemiology, and End Results (SEER) database using classical statistics and machine learning to explore the risk factors and construct a novel overall survival (OS) prediction nomogram for patients with SPC after lung cancer. Data of patients with SPC after lung cancer, covering 2000 to 2016, were gathered from the SEER database. The incidence of SPC after lung cancer was calculated by Standardized incidence ratios (SIRS). Cox proportional hazards regression, machine learning (ML), Kaplan-Meier (KM) methods, and log-rank tests were conducted to identify the important prognostic factors for predicting OS. These significant prognostic factors were used for the development of an OS prediction nomogram. Totally, 10,487 SPC samples were randomly divided into training and validation cohorts (model construction and internal validation) from the SEER database. In the random forest (RF) and extreme gradient boosting (XGBoost) feature importance ranking models, age was the most important variable which was also reflected in the nomogram. And, the models that combined machine learning with cox proportional hazards had a better predictive performance than the model that only used cox proportional hazards (AUC = 0.762 in RF, AUC = 0.737 in XGBoost, AUC = 0.722 in COX). Calibration curves and decision curve analysis (DCA) curves also revealed that our nomogram has excellent clinical utility. The web-based dynamic nomogram calculator was accessible on https://httseer.shinyapps.io/DynNomapp/. The prognosis characteristics of SPC following lung cancer were systematically reviewed. The dynamic nomogram we constructed can provide survival predictions to assist clinicians in making individualized decisions.
更多查看译文
关键词
Second primary cancer,Lung cancer,Risk factor,Nomogram,Survival
AI 理解论文
溯源树
样例
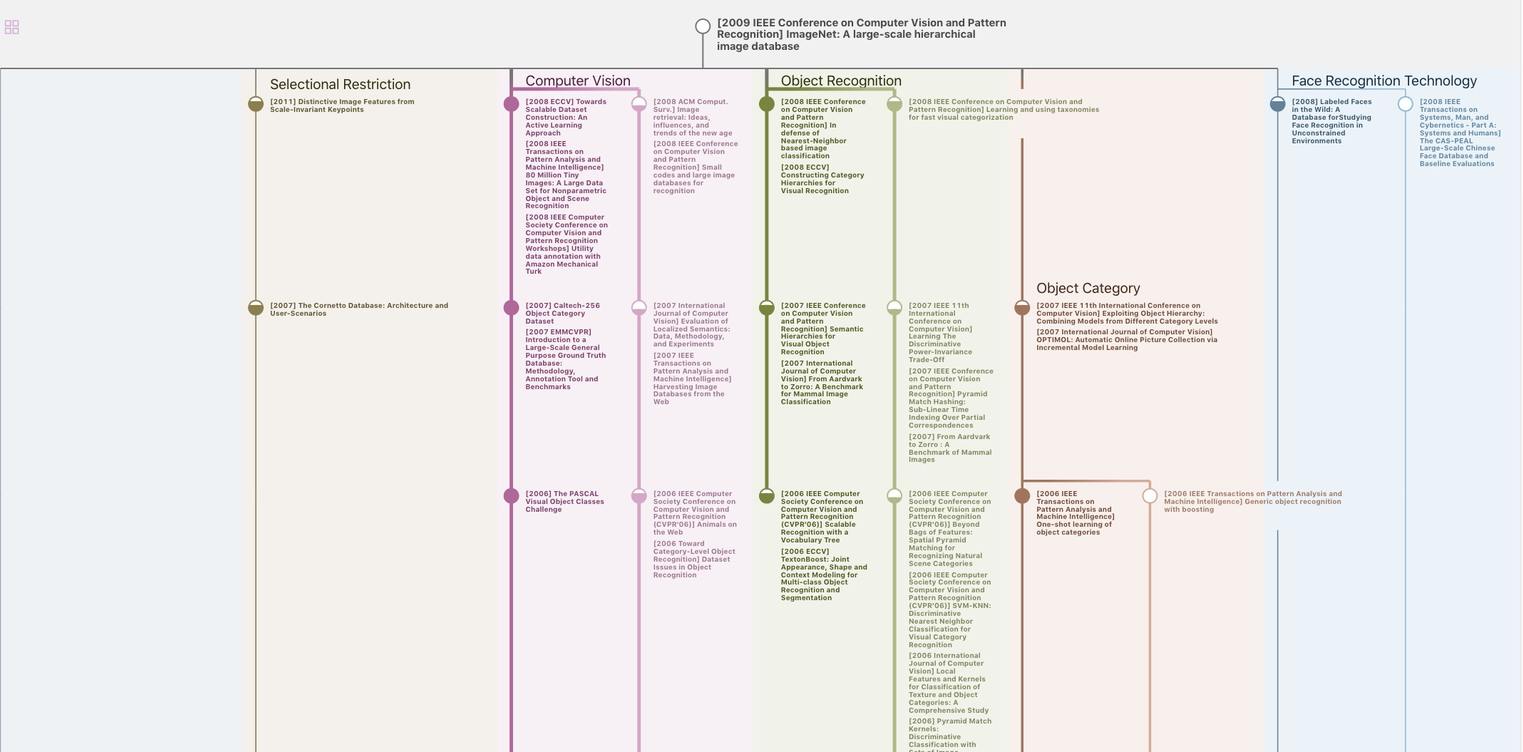
生成溯源树,研究论文发展脉络
Chat Paper
正在生成论文摘要