Security Analysis of Camera-LiDAR Fusion Against Black-Box Attacks on Autonomous Vehicles.
Usenix Security Symposium(2022)
摘要
To enable safe and reliable decision-making, autonomous vehicles (AVs) feed sensor data to perception algorithms to understand the environment. Sensor fusion with multi-frame tracking is becoming increasingly popular for detecting 3D objects. Thus, in this work, we perform an analysis of cameraLiDAR fusion, in the AV context, under LiDAR spoofing attacks. Recently, LiDAR-only perception was shown vulnerable to LiDAR spoofing attacks; however, we demonstrate these attacks are not capable of disrupting camera-LiDAR fusion. We then define a novel, context-aware attack: frustum attack, and show that out of 8 widely used perception algorithms – across 3 architectures of LiDAR-only and 3 architectures of camera-LiDAR fusion – all are significantly vulnerable to the frustum attack. In addition, we demonstrate that the frustum attack is stealthy to existing defenses against LiDAR spoofing as it preserves consistencies between camera and LiDAR semantics. Finally, we show that the frustum attack can be exercised consistently over time to form stealthy longitudinal attack sequences, compromising the tracking module and creating adverse outcomes on end-to-end AV control.
更多查看译文
关键词
security analysis,attacks,camera-lidar,black-box
AI 理解论文
溯源树
样例
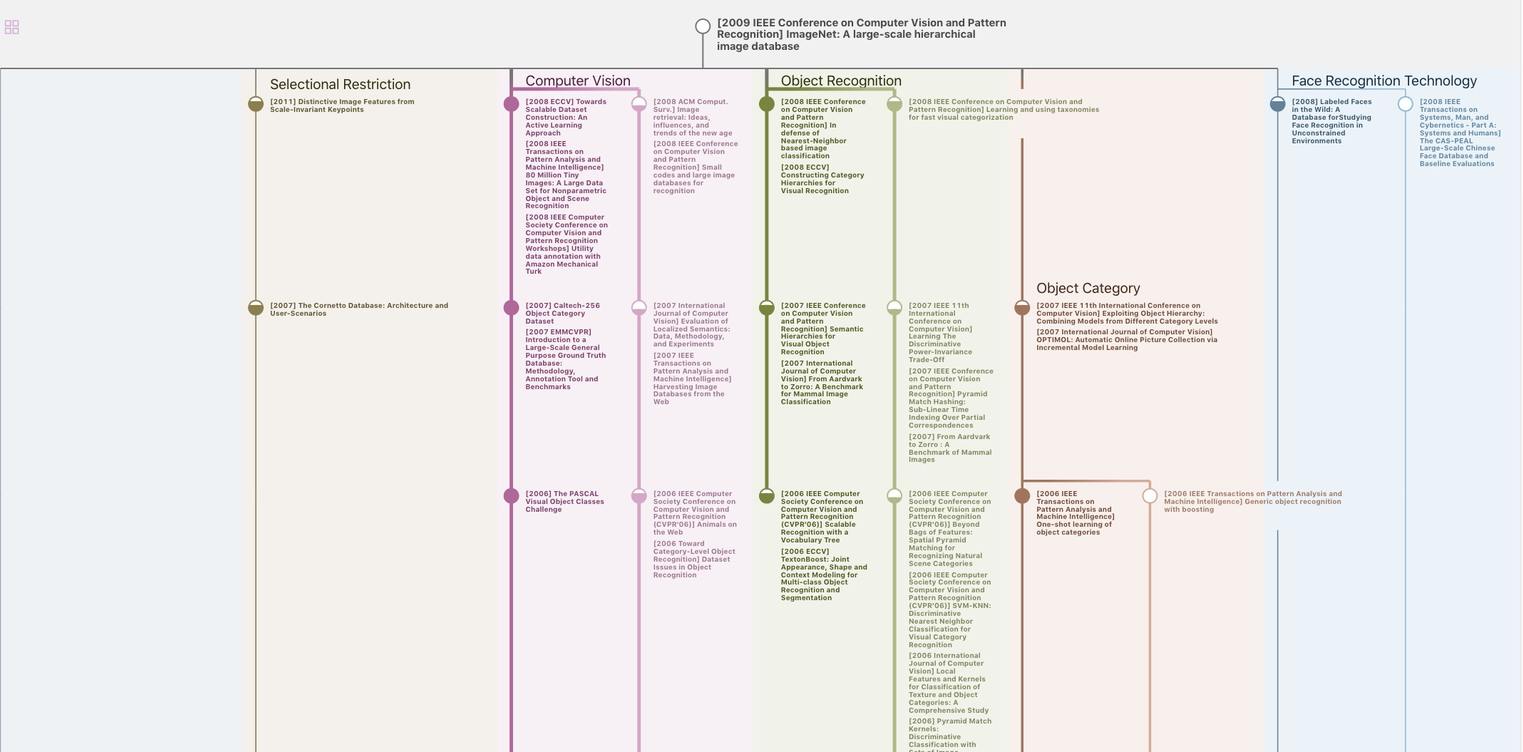
生成溯源树,研究论文发展脉络
Chat Paper
正在生成论文摘要