Towards Equitable Patient Subgroup Performance by Gene-Expression-Based Diagnostic Classifiers of Acute Infection
medRxiv(2022)
摘要
Host-response gene expression measurements may carry confounding associations with patient demographic characteristics that can induce bias in downstream classifiers. Assessment of deployed machine learning systems in other domains has revealed the presence of such biases and exposed the potential of these systems to cause harm. Such an assessment of a gene-expression-based classifier has not been carried out and collation of requisite patient subgroup data has not been undertaken. Here, we present data resources and an auditing framework for patient subgroup analysis of diagnostic classifiers of acute infection. Our dataset comprises demographic characteristics of nearly 6500 patients across 49 studies. We leverage these data to detect differences across patient subgroups in terms of gene-expression-based host response and performance with both our candidate pre-market diagnostic classifier and a standard-of-care biomarker of acute infection. We find evidence of variable representation with respect to patient covariates in our multi-cohort datasets as well as differences in host-response marker expression across patient subgroups. We also detect differences in performance of multiple host-response-based diagnostics for acute infection. This analysis marks an important first step in our ongoing efforts to characterize and mitigate potential bias in machine learning-based host-response diagnostics, highlighting the importance of accounting for such bias in developing diagnostic tests that generalize well across diverse patient populations.
更多查看译文
关键词
equitable patient subgroup performance,diagnostic classifiers,acute,infection,gene-expression-based gene-expression-based
AI 理解论文
溯源树
样例
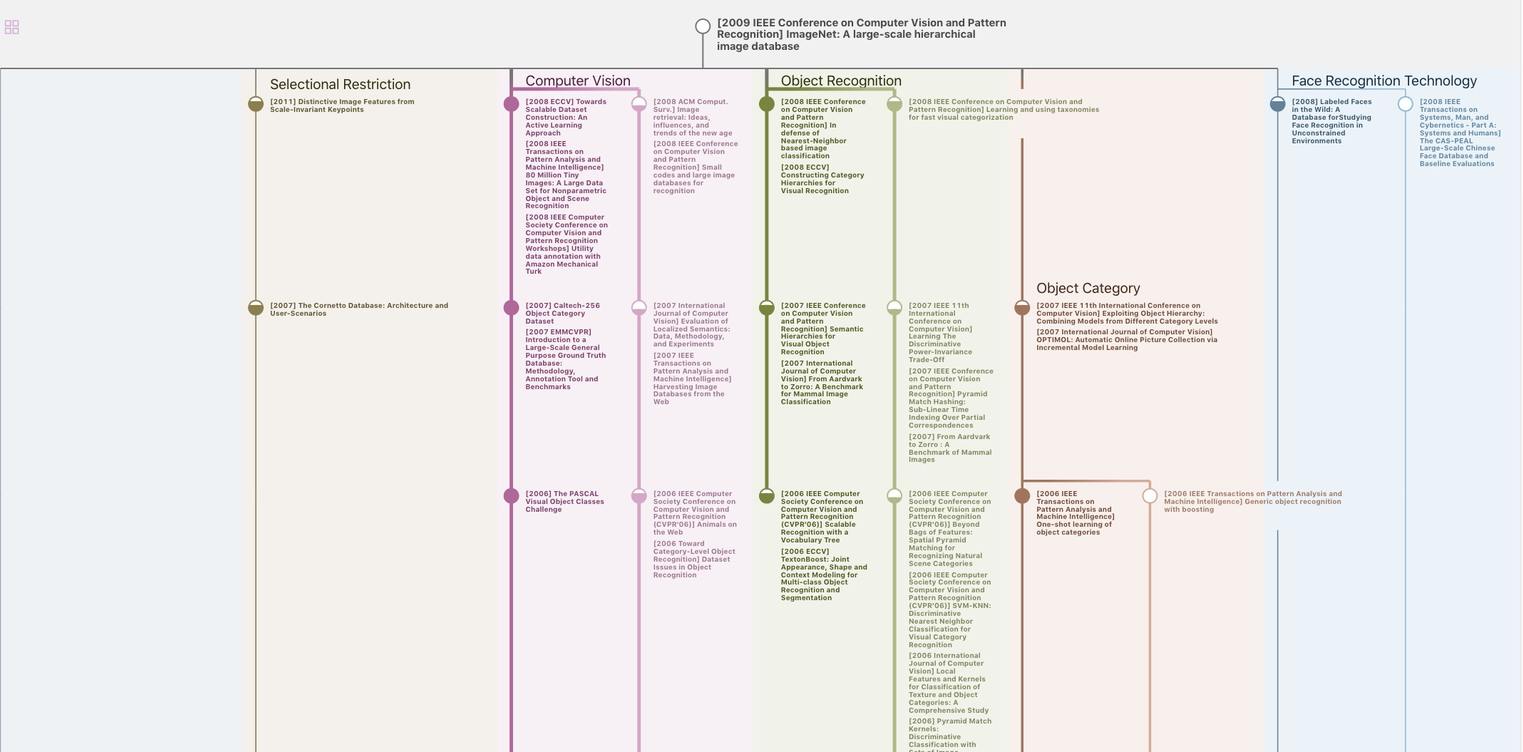
生成溯源树,研究论文发展脉络
Chat Paper
正在生成论文摘要