Sparse Neural Generative Inference Based Pose Estimation
semanticscholar(2021)
Abstract
Current state-of-the-art pose estimation techniques often rely on end-to-end trained neural network architectures to infer a 6-DOF pose. These architectures, while effective, are computationally expensive and often require a large number of parameters. Instead, we propose formulating the pose estimation problem as a differentiable particle filter. This approach learns a per-particle latent embedding which infers its respective pose, object likelihood, and re-sampling objective iteratively. Our proposed method should decrease the tight coupling between input size and model architecture, and also improve the training and inference time scalability of the network. Furthermore, the proposed approach allows for reasoning across a distribution of inferred poses represented by the different particles.
MoreTranslated text
AI Read Science
Must-Reading Tree
Example
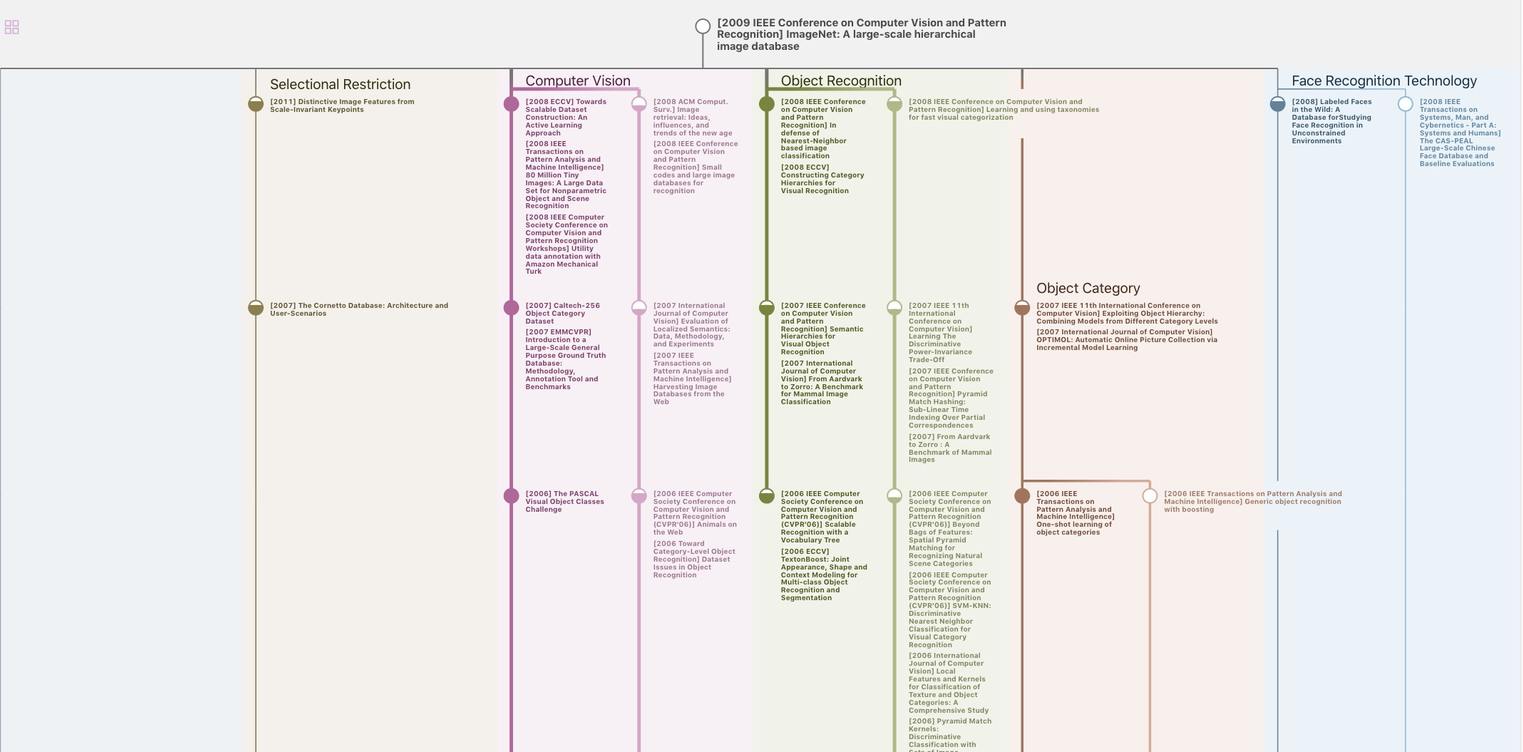
Generate MRT to find the research sequence of this paper
Chat Paper
Summary is being generated by the instructions you defined