Predicting remaining execution time of business process instances via auto-encoded transition system
INTELLIGENT DATA ANALYSIS(2022)
Abstract
As an important task in business process management, remaining time prediction for business process instances has attracted extensive attentions. However, most of the traditional remaining time prediction approaches only take into account formal process models and cannot handle large-scale event logs in an effective manner. Although machine learning and deep learning have been recently applied to the remaining time prediction task, these approaches cannot incorporate domain knowledge naturally. To overcome these weaknesses of existing studies, we propose a remaining execution time prediction approach based on a novel auto-encoded transition system, which can enhance the complementarity of process modeling and deep learning techniques. Through auto-encoding the event-level and state-level features, the proposed approach can represent process instances in a comprehensive and compact form. Furthermore, a transfer learning strategy is proposed to train the remaining time prediction model so as to avoid overfitting and improve the accuracy of prediction. We conduct extensive experiments on four real-world datasets to verify the effectiveness of the proposed approach. The results show its superiority over several state-of-the-art approaches.
MoreTranslated text
Key words
Business process management,event log,transition system,auto-encoder,remaining execution time
AI Read Science
Must-Reading Tree
Example
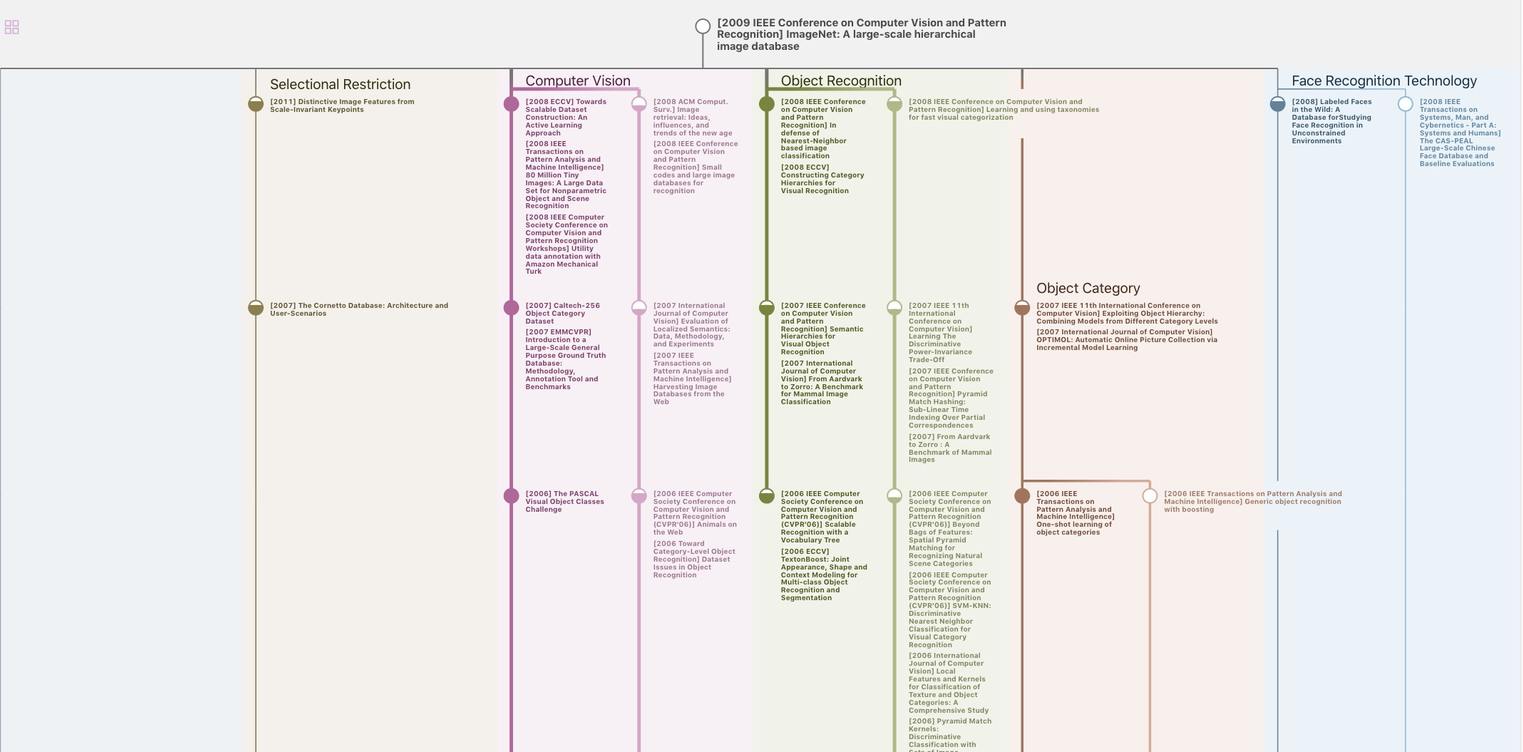
Generate MRT to find the research sequence of this paper
Chat Paper
Summary is being generated by the instructions you defined