Constraints on Theories of Open-World Learning
semanticscholar(2022)
摘要
In this paper, I examine challenges that arise in developing theories of open-world learning. After defining the problem, I review some theories from the history of chemistry, biology, geology, and AI, along with the importance of inductive bias to constrain the learning process. Classic cognitive architectures offer one source for such guidance, but their generality provides little aid on this front. Instead, I propose that more constrained architectures for embodied agents have greater potential, as they make commitments about the form of domain knowledge used to describe environments, as well as the processes that operate over them. In addition, I hypothesize that autonomous agents must include motivational structures that drive behavior and that changes to the environment can lead to their revision as well. I argue that a full account of open-world learning should make commitments about the structures and processes that underlie these capabilities. Autonomous Agency in Open Worlds Advances in sensors, effectors, memories, and processors have led to autonomous agents that are far more capable and common than those from only a decade ago. These take on many different forms, from self-driving cars and delivery drones to military robots and planetary rovers. The development of such systems typically relies on collection and processing of very large training sets to create accurate pattern recognizers and efficient controllers. This approach is viable for some applications and certain contexts, but it depends on two related assumptions: the environment will not change in important ways; and the agent’s expertise will remain accurate and appropriate. Unfortunately, these postulates will not hold in many real-world settings. We would like autonomous agents that are robust to such shifts. For example, consider an unmanned aerial drone on an exploratory mission in the Amazon rainforest. The system’s expertise remains accurate and its behavior is acceptable until an airborne spider’s web tangles one of its rotor blades, a large predatory bird attacks it from above, a strong updraft pulls it off course, a dense fog bank degrades its visibility, or high humidity causes intermittent shorts in its camera controller. A truly flexible autonomous agent would reCopyright c © 2022, Association for the Advancement of Artificial Intelligence (www.aaai.org). All rights reserved. alize, in each case, that its expertise was outdated and adapt rapidly enough to still achieve the mission goals. Scenarios of this sort raise the challenge of open-world learning, a class of problems introduced by DARPA’s SAILON program (Senator, 2019). We can state this problem as: • Given: An agent architecture that operates in some class of tasks and environments; • Given: Expertise that supports acceptable performance for these tasks and environments; • Given: Limited experience after sudden, unannounced changes to the environment degrade performance; • Find: When the environmental change occurs and what revised expertise will give acceptable performance. This formulation applies to many agents, environments, and tasks, regardless of whether their initial expertise is handcrafted or learned from experience. The problem of open-world learning addresses the very heart of what we mean by the term ‘autonomous agent’. We say that an entity is an ‘agent’ if it carries out actions that affect its environment over time. However, in this light, teleoperated robots and remote-controlled drones would count as agents. We say that an agent is ‘autonomous’ if it operates independently and without supervision, but this can hold to different degrees. Thermostats are autonomous but only in a very narrow context. Robot vacuum cleaners have a broader range of behaviors but have been programmed by humans. Humans fall at the spectrum’s extreme end, because they can not only adapt their model of the world but, in some cases, alter their own motivations and value systems. Some readers may question why open-world learning poses a challenge, in that modern techniques for machine learning have been widely advertised as the solution to nearly any problem. However, remember that environmental shifts can be sudden and unannounced and that the agent must detect them and repair its expertise rapidly. The most widely adopted methods for classification learning, despite their success in some settings, rely on batch processing and require many labeled training cases, neither of which are sufficient here. Reinforcement learning, a popular approach to sequential action selection, typically requires many runs on a simulator, which will not be available for unfamiliar physical environments. In summary, mainstream approaches to machine learning are ill suited to such scenarios. In the pages that follow, I discuss a number of issues related to theories of open-world learning. First, I review some familiar examples from the history of science, including artificial intelligence, and their lessons for accounts of learning. After noting the need for some form of inductive bias to make the search process tractable, I consider what cognitive architectures can offer to this end. Upon concluding that they offer only weak constraints, I turn to more specialized architectures for embodied agents that include theoretical commitments about knowledge of the physical environment. Finally, I argue that a complete theory of open-world learning must address not only how agents can alter their environmental models, but how they can alter their own motivations in response to such changes. Theories of Open-World Learning Given that we want the research community to develop theories of open-world learning, we should consider the form that such accounts might take. The history of science, including the early phases of artificial intelligence, offer compelling examples from which researchers can draw useful lessons. In this section, I review three classic theories from the disciplines of chemistry, biology, and geology, along with three others cases from the study of intelligent systems. In closing, I revisit some familiar ideas about the need for constraints in machine learning. Scientific theories aim to explain observed phenomena in terms of a set of interconnected claims or assumptions. Here are three well-known examples: • Dalton’s (1808) atomic theory posited that macroscopic objects are made from tiny molecules, each involving atoms of nondecomposable elements. Moreover, chemical reactions transform some types of molecules into other types by rearranging their constituent atoms. • Pasteur’s (1880) germ theory of disease proposed that many illnesses are caused by small organisms that invade the body and attack it. In addition, these germs spread from one host to another through the process of infection. • Hess’s (1962) theory of plate tectonics stated that interlocking plates make up the Earth’s surface, with mountains and deep sea trenches at their interfaces. These plates move very slowly under, over, and against each other to produce large-scale geological formations. There are many analogous examples from the history of science, but we can draw some tentative conclusions from this set about the character of theories. Despite their many differences in form and content, scientific theories nearly always include postulates that impose qualitative constraints on the domain under study. This holds even when the account also includes quantitative elements, which are often introduced after a field has agreed about their qualitative aspects. Moreover, theories posit both structures (e.g., entities and their relations) and processes that operate over and transform them. For instance, molecules and atoms are structures in the atomic theory, whereas chemical reactions are processes that affect them. Also, theories are abstract enough that they cannot be tested directly; this can only occur when one has added enough assumptions to produce operational models. For example, the atomic theory must be augmented by specific claims about the constituents of particular molecules, while germ theory requires associations between specific microorganisms and diseases. Finally, scientific theories regularly elaborate earlier ones with which they share assumptions, as the immune theory builds on the more basic germ theory. We should also consider examples from the early days of artificial intelligence, which illustrate many of the same characteristics. These include three classic theories: • Physical symbol systems (Newell & Simon, 1976), which posits that mental structures consist of symbols (persistent physical patterns) and symbol structures (organized sets of such symbols), which in turn can designate other entities or activities. This theory also proposes mental processes that create, modify, and interpret an evolving sequence of these symbol structures. • Production systems (Newell, 1966), which elaborates on the first theory by postulating memories that contain sets of modular elements encoded as symbol structures, including a rapidly changing working memory and a more stable long-term store with condition-action rules. Processing involves repeatedly matching rules against elements in working memory and using them to alter its contents, which in turn enables new matches. • Heuristic search (Newell & Simon, 1976), which also extends the first framework by declaring that problem solving relies on symbol structures to denote candidate solutions, generators of candidates, criteria for acceptance, and heuristics. This theory assumes processes for generating candidate solutions, testing them for acceptability, and using heuristics to guide choices. These examples clarify that theories of intelligent behavior specify both structures and processes that operate over them. They also have the same abstract, qualitative character as the cases from chemistry, biology, and geology. These observations are relevant for researchers wh
更多查看译文
AI 理解论文
溯源树
样例
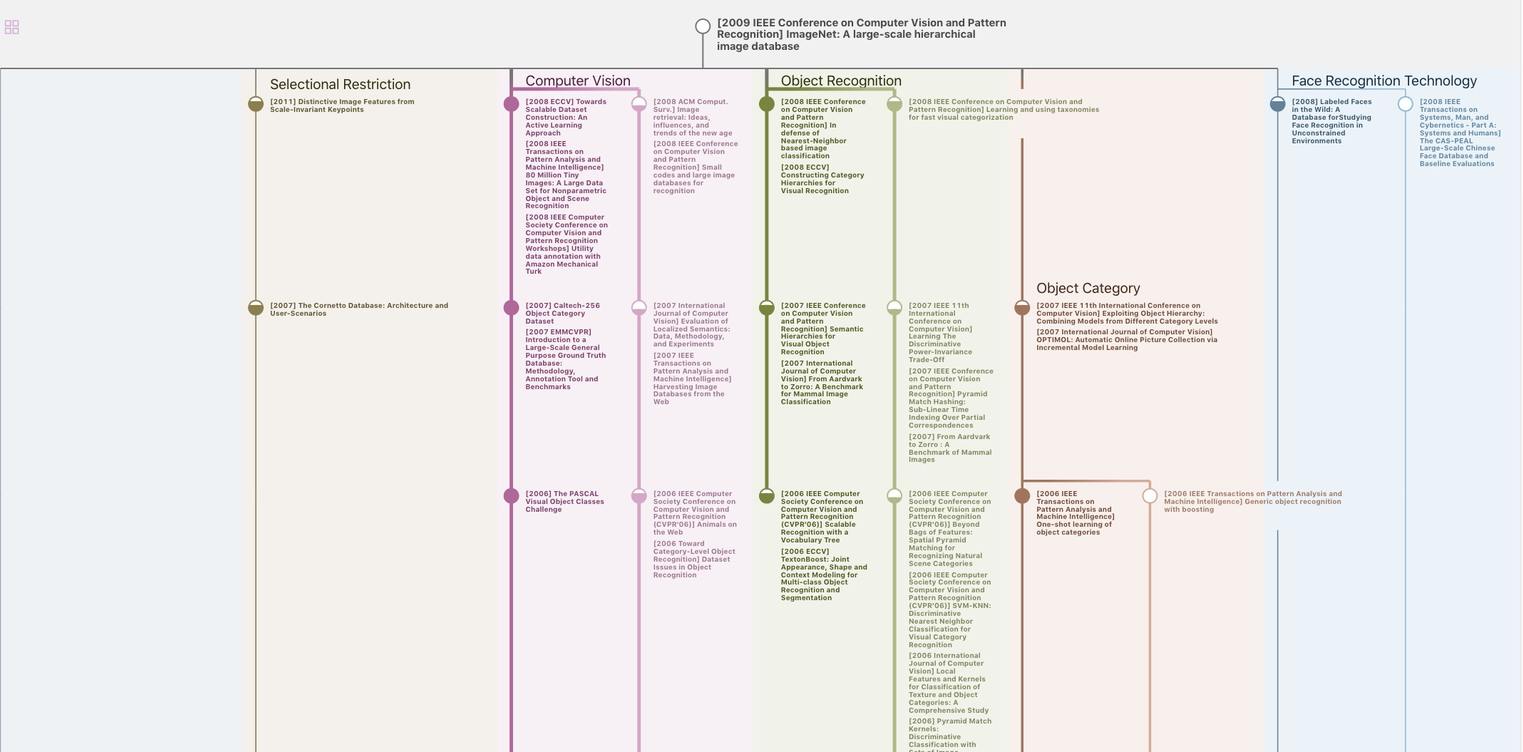
生成溯源树,研究论文发展脉络
Chat Paper
正在生成论文摘要