Meta-learning richer priors for VAEs
semanticscholar(2022)
摘要
Variational auto-encoders have proven to capture complicated data distributions and useful latent representations, while advances in meta-learning have made it possible to extract prior knowledge from data. We incorporate these two approaches and propose a novel flexible prior, namely the Pseudo-inputs prior, to obtain a richer latent space. We train VAEs using the Model-Agnostic Meta-Learning (MAML) algorithm and show that it achieves comparable reconstruction performance with standard training. However, we show that this MAML-VAE model learns richer latent representations, which we evaluate in terms of unsupervised few-shot classification as a downstream task. Moreover, we show that our proposed Pseudo-inputs prior outperforms baseline priors, including the VampPrior, in both models, while also encouraging high-level representations through its pseudo-inputs.
更多查看译文
AI 理解论文
溯源树
样例
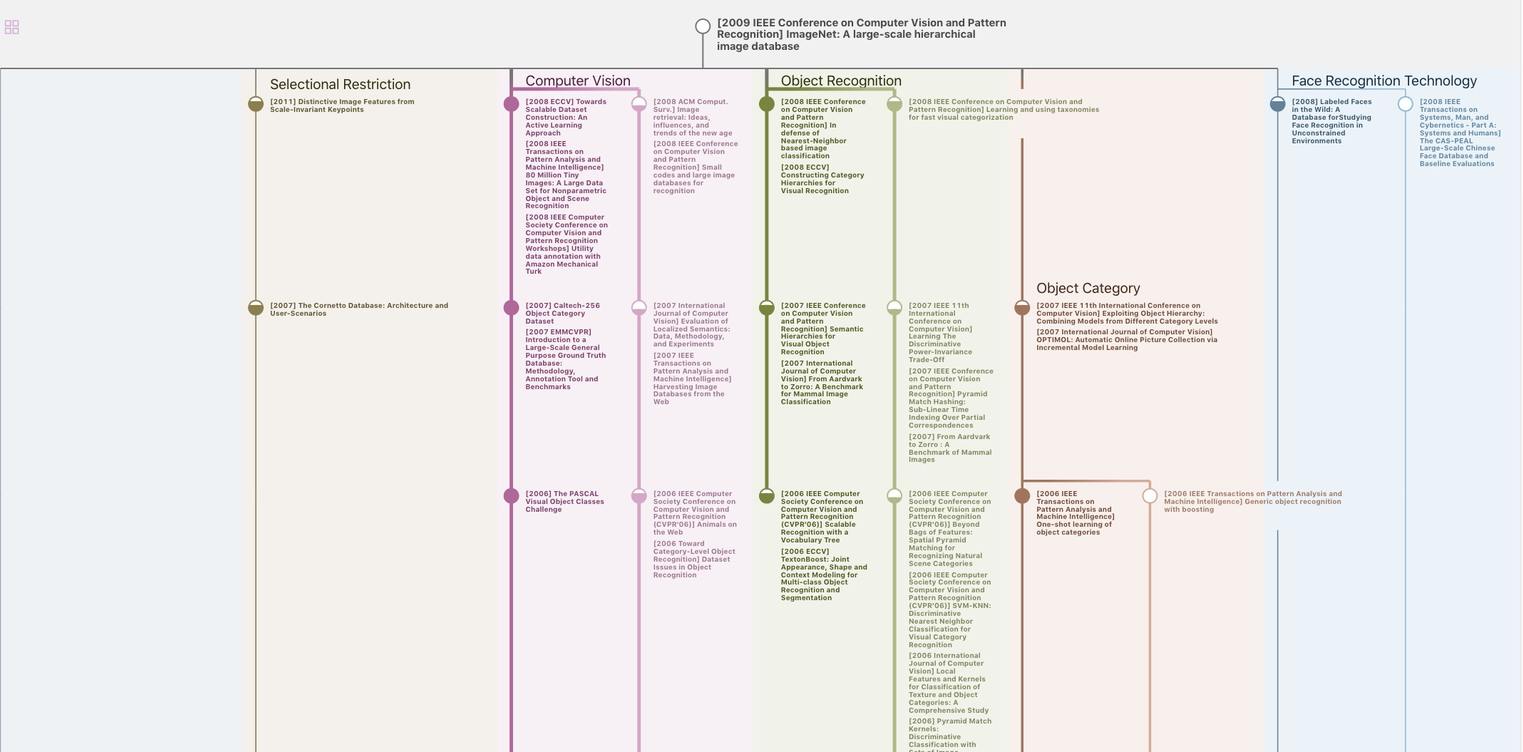
生成溯源树,研究论文发展脉络
Chat Paper
正在生成论文摘要