Assessing the Sensitivity of Vegetation Cover to Climate Change in the Yarlung Zangbo River Basin Using Machine Learning Algorithms
REMOTE SENSING(2022)
Abstract
Vegetation is a key indicator of the health of most terrestrial ecosystems and different types of vegetation exhibit different sensitivity to climate change. The Yarlung Zangbo River Basin (YZRB) is one of the highest basins in the world and has a wide variety of vegetation types because of its complex topographic and climatic conditions. In this paper, the sensitivity to climate change for different vegetation types, as reflected by the Normalized Difference Vegetation Index (NDVI), was assessed in the YZRB. Three machine learning models, including multiple linear regression, support vector machine, and random forest, were adopted to simulate the response of each vegetation type to climatic variables. We selected random forest, which showed the highest performance in both the calibration and validation periods, to assess the sensitivity of the NDVI to temperature and precipitation changes on an annual and monthly scale using hypothetical climatic scenarios. The results indicated there were positive responses of the NDVI to temperature and precipitation changes, and the NDVI was more sensitive to temperature than to precipitation on an annual scale. The NDVI was predicted to increase by 1.60-4.68% when the temperature increased by 1.5 degrees C, while it only changed by 0.06-0.24% when the precipitation increased by 10% in the YZRB. Monthly, the vegetation was more sensitive to temperature changes in spring and summer. Spatially, the vegetation was more sensitive to temperature increases in the upper and middle reaches, where the existing temperatures were cooler. The time-lag effects of climate were also analyzed in detail. For both temperature and precipitation, Needleleaf Forest and Broadleaf Forest had longer time lags than those of other vegetation types. These findings are useful for understanding the eco-hydrological processes of the Tibetan Plateau.
MoreTranslated text
Key words
vegetation response,attribution analysis,machine learning,NDVI,the Yarlung Zangbo River Basin
AI Read Science
Must-Reading Tree
Example
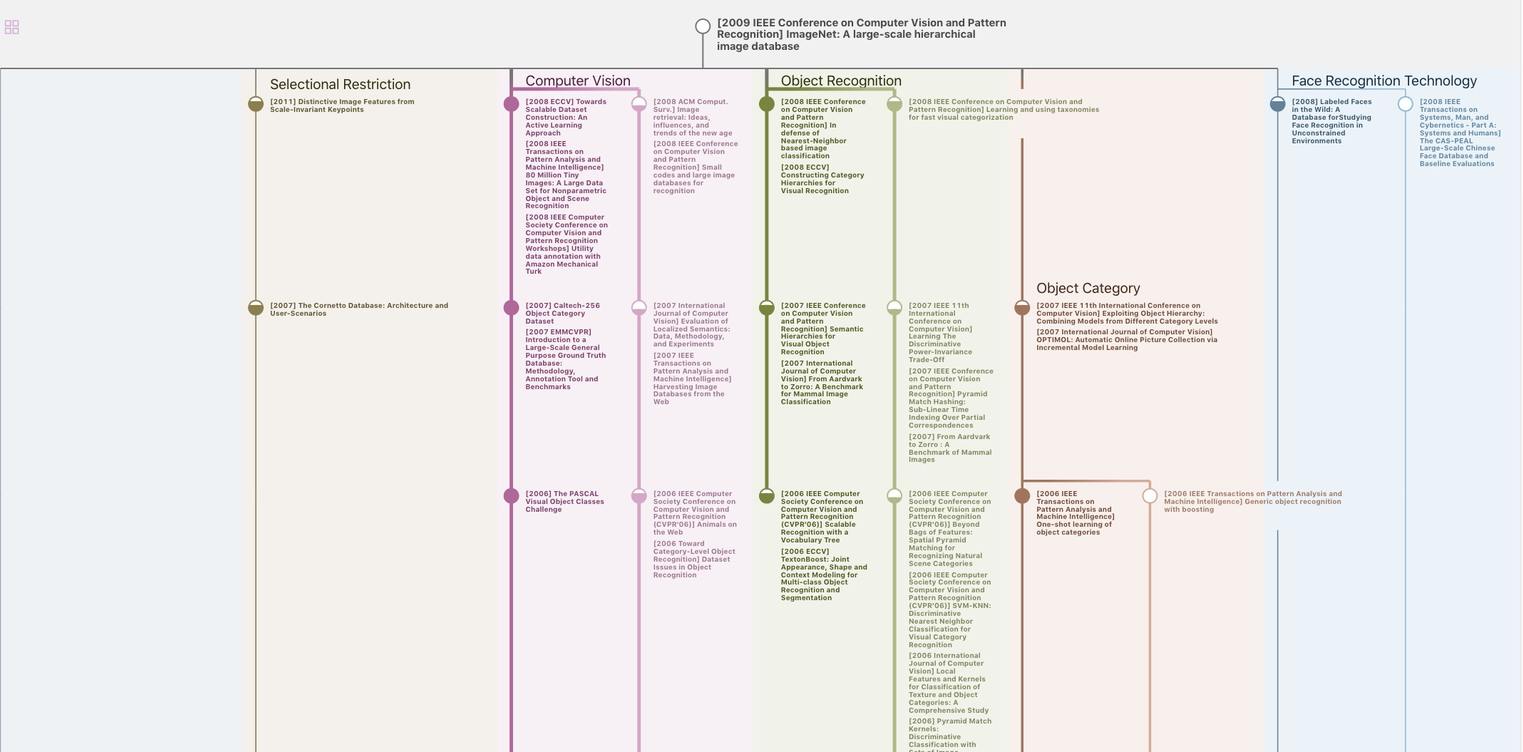
Generate MRT to find the research sequence of this paper
Chat Paper
Summary is being generated by the instructions you defined