Exploration of Sub-Optimal Constellation Maps for Coherent Fiber-Optic Communication links by Auto-Encoder Type Machine Learning
2022 International Electrical Engineering Congress (iEECON)(2022)
Abstract
Autoencoder (AE) was applied to the exploration of sub-optimal constellation maps and decision boundaries in a coherent optical fiber communication link. Single-wavelength point-to-point single-mode fiber coherent optical communication link that considers wavelength dispersion and self-phase modulation was incorporated in the middle of AE and sub-optimal constellation maps / decision boundaries were automatically acquired by learning AE under various conditions. The transmitting side of AE automatically configured an encoder, so called AE modulation (AEM), from one-hot-vectors to IQ constellations considering noise, wavelength dispersion, and self-phase modulation (SPM) given during transmission, and the receiving side automatically configured a decoder having linear decision boundaries from the received IQ constellations to one-hot vectors. Setting an appropriate signal-to-noise power ratio (SNR) during AE learning resulted in constellations and decision boundaries stable for wide range of operating SNR. The obtained BER characteristic for 16AEM was approaching to the one of the compensated 16 quadrature amplitude modulation (16QAM).
MoreTranslated text
Key words
machine learning,sub-optimal,fiber-optic,auto-encoder
AI Read Science
Must-Reading Tree
Example
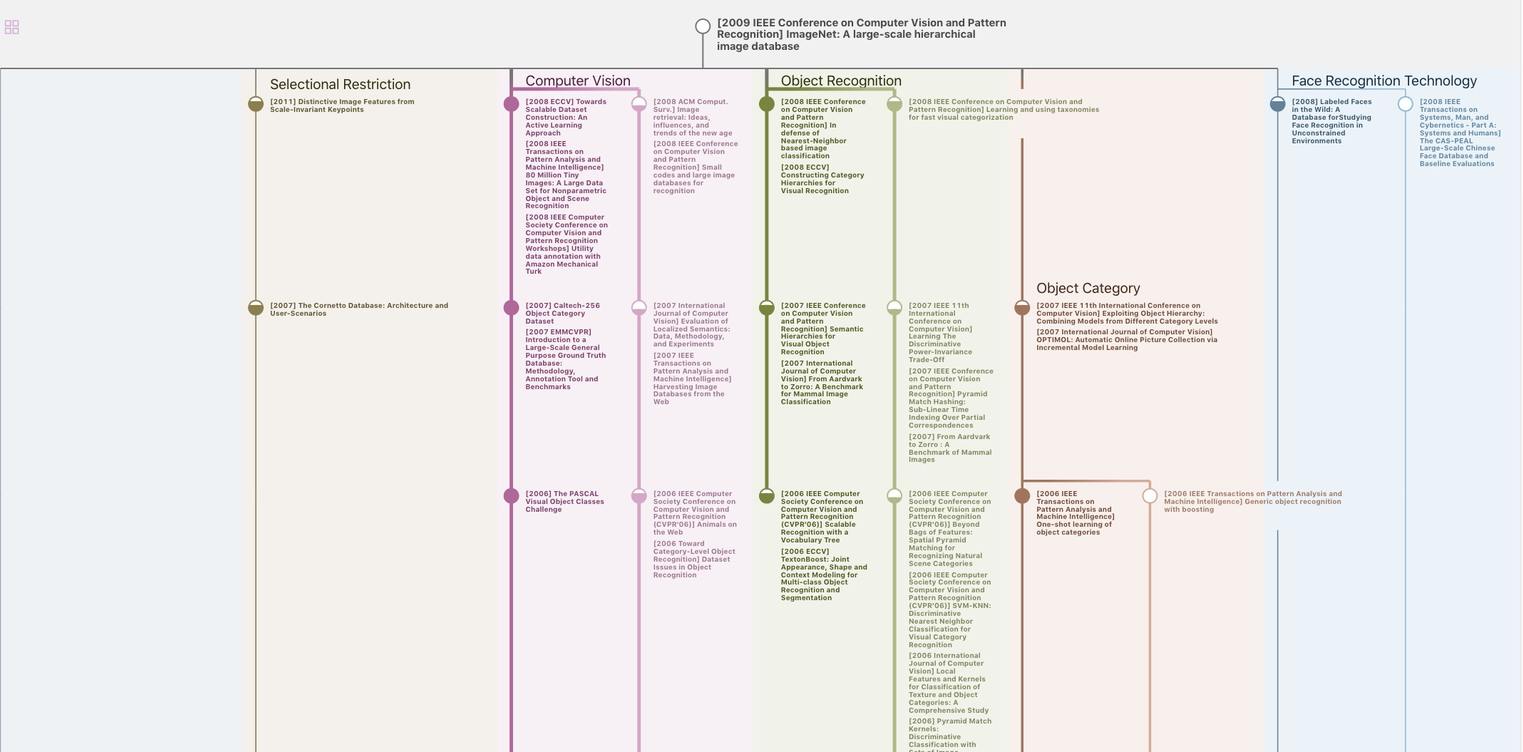
Generate MRT to find the research sequence of this paper
Chat Paper
Summary is being generated by the instructions you defined