Deep Learning Based Detection for Communications Systems With Radar Interference
IEEE Transactions on Vehicular Technology(2022)
摘要
Due to the increasing demand for spectrum resources, the co-existence of communications and radar systems has been proposed that allows radar and communications systems to operate in the same frequency band. On the other hand, deep learning has shown great potential in revolutionizing communications systems. In this work, we investigate the use of deep learning in communications systems subject to interference from radar systems. Specifically, we consider a single-carrier communications system. Linear frequency-modulated (LFM) and frequency-modulated continuous-wave (FMCW) are considered for radar. Several important system parameters, including the level of noise and interference, the radar interference coverage, the symbol duration, feature extraction methods and the number of hidden layers are investigated for the performance of the detector. Fully connected deep neural network (FCDNN) and long short-term memory (LSTM) detectors are implemented, where principle component analysis (PCA) is applied to preprocess the observed signals for the FCDNN detector. Numerical results show that the learning-based detector achieves comparable performance in the radar-communication system to the traditional detector but without interference cancellation. Preprocessing the received signals with PCA can improve the performance of FCDNN when interference is strong. Also, LSTM shows more robust performance than FCDNN when the channel has time-related distortion.
更多查看译文
关键词
Communications,deep learning,radar interference,signal detection
AI 理解论文
溯源树
样例
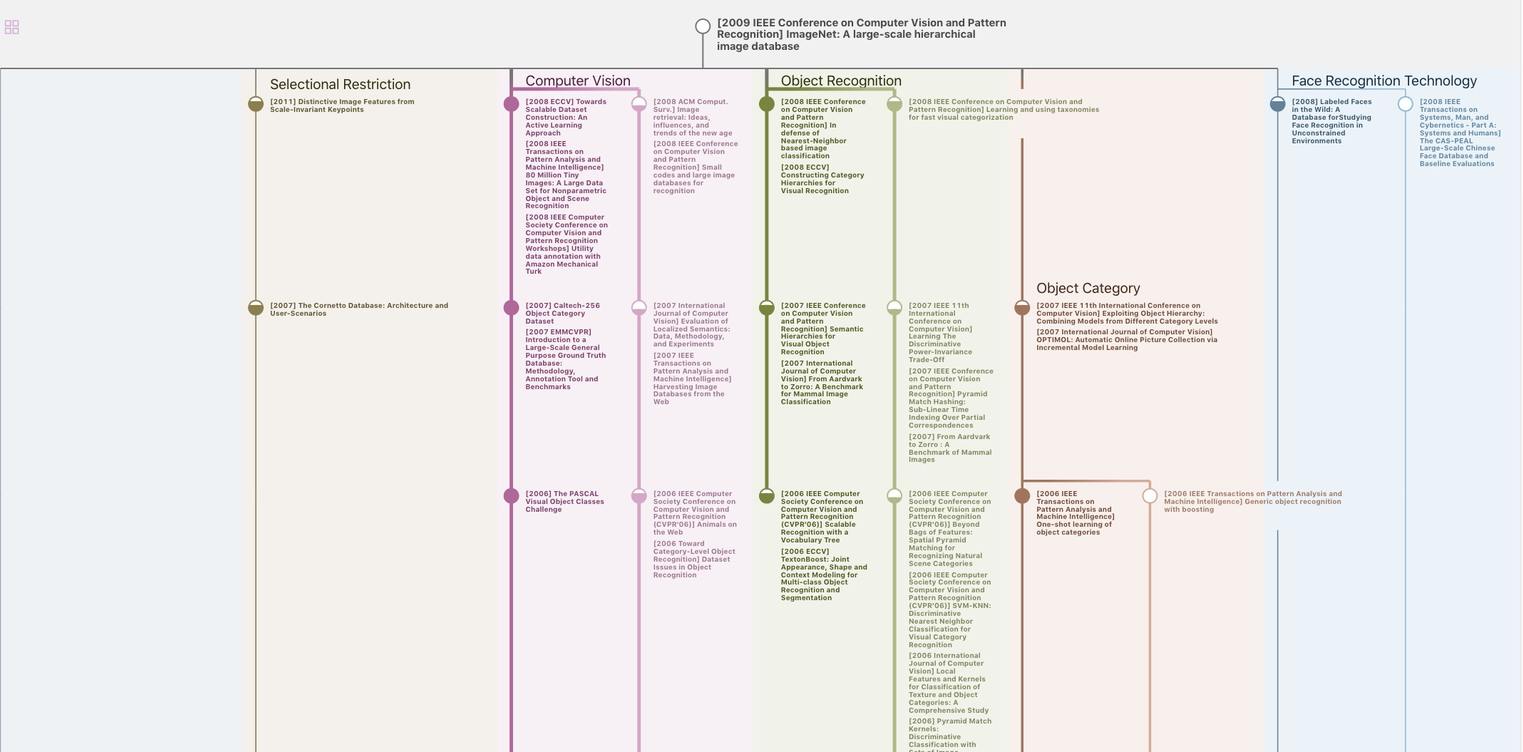
生成溯源树,研究论文发展脉络
Chat Paper
正在生成论文摘要