A self-consistent-field iteration for MAXBET with an application to multi-view feature extraction
ADVANCES IN COMPUTATIONAL MATHEMATICS(2022)
摘要
As an extension of the traditional principal component analysis, the multi-view canonical correlation analysis (MCCA) aims at reducing m high dimensional random variables s(i) is an element of R-i(n) lens (i = 1, 2, ..., m) by proper projection matrices X-i is an element of R-n (x l)(i) so that the m reduced ones y(i) = X(i)(T)s(i) is an element of R-l have the "maximal correlation." Various measures of the correlation for y(i) (i = 1, 2, ..., m) in MCCA have been proposed. One of the earliest criteria is the sum of all traces of pair-wise correlation matrices between y(i) and y(i) subject to the orthogonality constraints on X-i , i = 1, 2, . , m. The resulting problem is to maximize a homogeneous quadratic function over the product of Stiefel manifolds and is referred to as the MAXBET problem. In this paper, the problem is first reformulated as a coupled nonlinear eigenvalue problem with eigenvector dependency (NEPv) and then solved by a novel self-consistent-field (SCF) iteration. Global and local convergences of the SCF iteration are studied and proven computational techniques in the standard eigenvalue problem are incorporated to yield more practical implementations. Besides the preliminary numerical evaluations on various types of synthetic problems, the efficiency of the SCF iteration is also demonstrated in an application to multi-view feature extraction for unsupervised learning.
更多查看译文
关键词
MAXBET,Multi-view canonical correlation analysis,Multi-view feature extraction,Nonlinear eigenvalue problem,Self-consistent-field iteration,Stiefel manifold
AI 理解论文
溯源树
样例
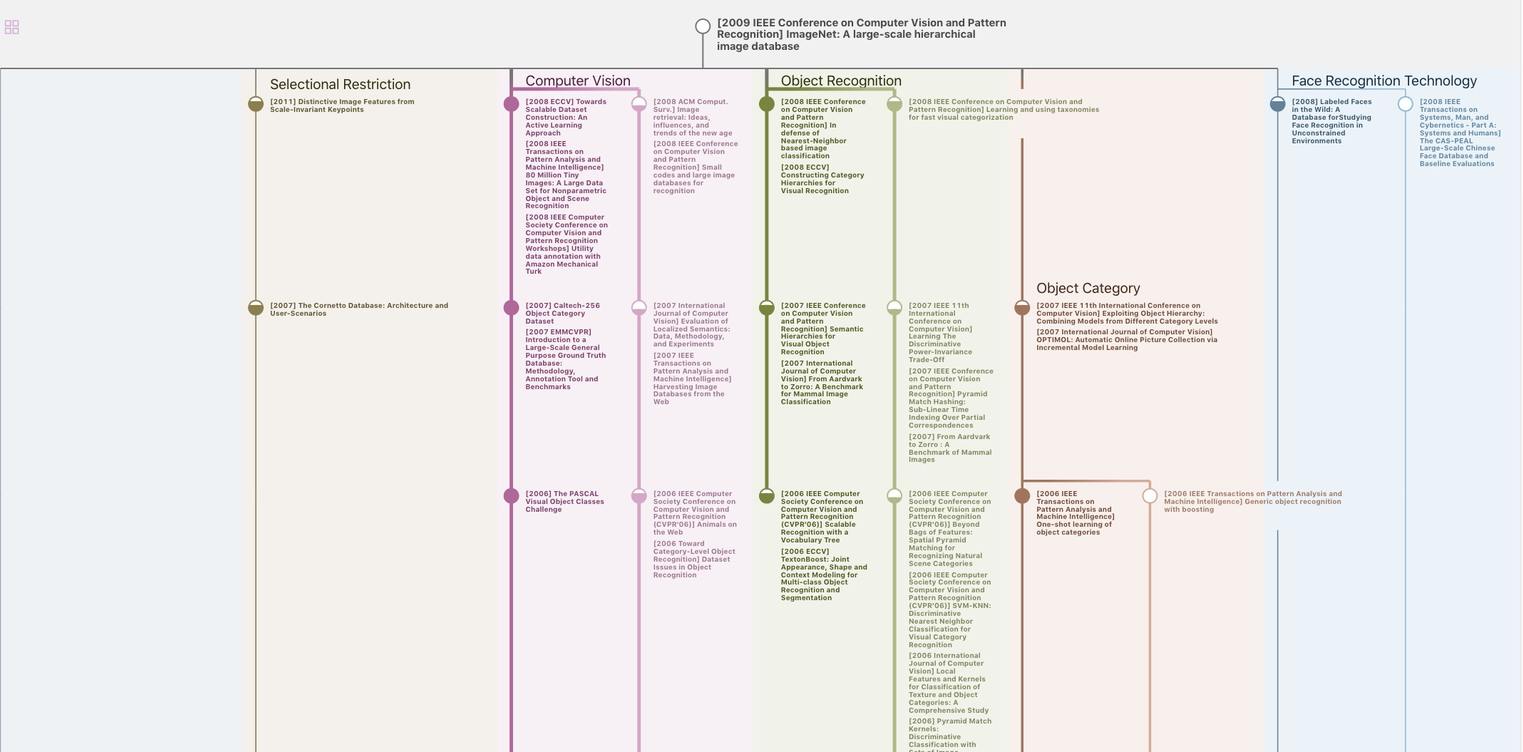
生成溯源树,研究论文发展脉络
Chat Paper
正在生成论文摘要