Diversifying Tire-Defect Image Generation Based on Generative Adversarial Network
IEEE TRANSACTIONS ON INSTRUMENTATION AND MEASUREMENT(2022)
摘要
With the development of data-driven models, deep learning has been increasingly applied in the field of defect detection. However, the performance of deep learning models is greatly restricted by costly labeling and sample scarcity. One of the best approaches to solve the data imbalance problem is increasing the quantity and diversity of defect samples. Meanwhile, the current models based on generative adversarial network (GAN) cannot readily control the category and shape of generated defect samples, which results in inefficient data augmentation. Thus, to simultaneously achieve the category and shape adjustability of defect samples, a novel model named diversifying tire-defect GAN (DTD-GAN) is proposed in this article by integrating the advantages of latent space decomposition and feature decoupling algorithm. In this model, an auxiliary classifier is designed to control the defect category by discrete latent codes, and the mutual information maximization approach is adopted to diversify the tire-defect shape via continuous latent codes that greatly improve the diversity of the generated tire-defect samples. To illustrate the effectiveness of the proposed DTD-GAN model, extensive experiments on image quality and diversity assessment are carried out on the images generated by this model and other state-of-the-art approaches, and the achieved improvement in deep learning model performance in a tire-defect detection task is also discussed.
更多查看译文
关键词
Tires,Generative adversarial networks,Feature extraction,Deep learning,Shape,Data models,Training,Data augmentation,feature decoupling,generative adversarial network (GAN),generative control,tire-defect detection
AI 理解论文
溯源树
样例
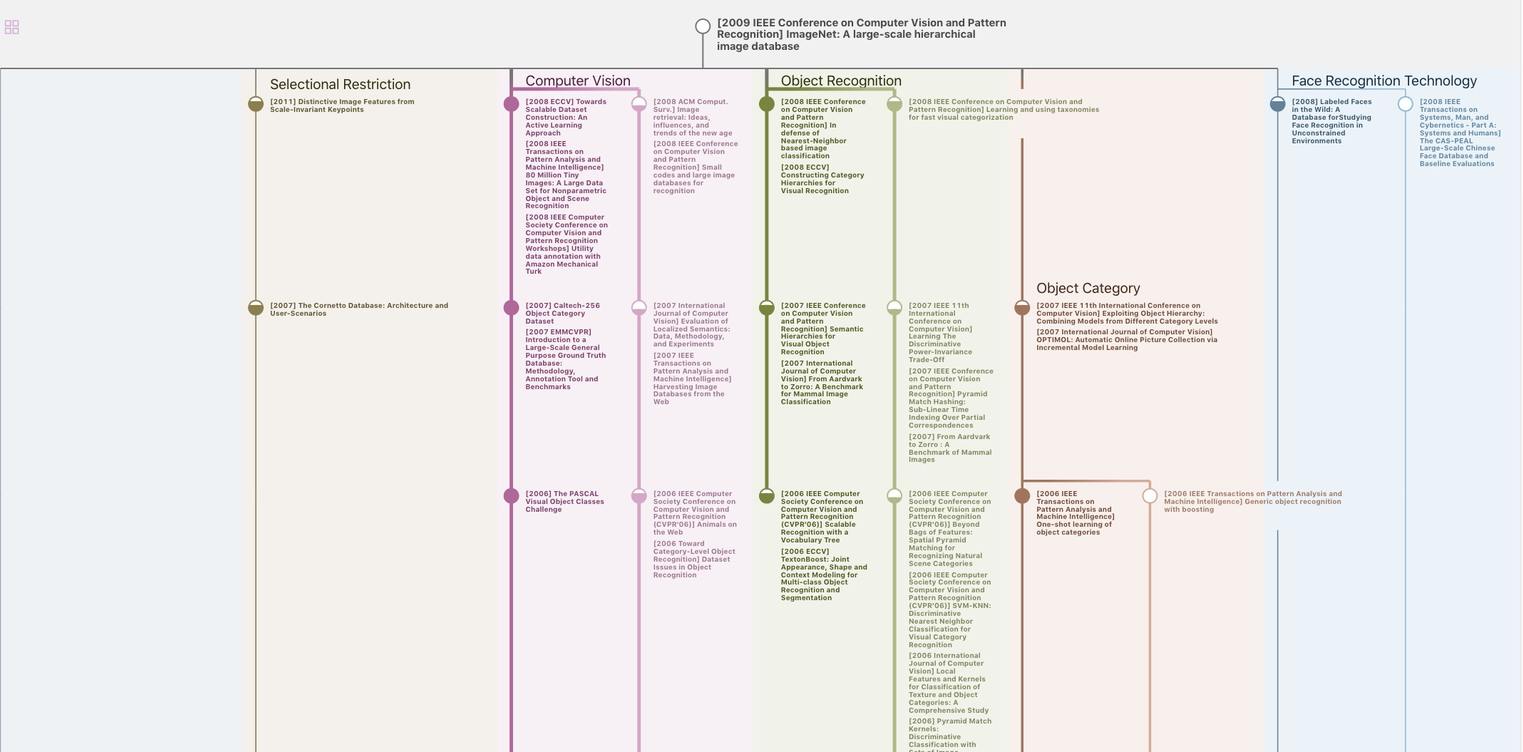
生成溯源树,研究论文发展脉络
Chat Paper
正在生成论文摘要