A reinforcement learning agent for mixed-numerology interference-aware slice spectrum allocation with non-deterministic and deterministic traffic
Computer Communications(2022)
摘要
5G RAN slicing is an essential tool to support the simultaneous coexistence of enhanced mobile broadband (eMBB) and ultra-reliable low-latency communications (URLLC) network slices on a shared mixed-numerology physical layer. Moreover, due to recent advance of the private network paradigm, RAN slicing assumes a central role to provide dedicated radio coverage to industry 4.0 applications as a standalone RAN. Unlike the stochastic traffic behavior characterizing URLLC slices in classical mobile networks, industrial networks support URLLC services with deterministic and periodic traffic patterns. Based on this alternative network characterization, we design a deep reinforcement learning (DRL) agent that simultaneously provides a spectrum allocation fulfilling the eMBB and URLLC service requirements and mitigates the inter-numerology interference (INI). Furthermore, by exploiting the information about the deterministic traffic patterns, we specialize the agent reward function to improve the spectrum allocation effectiveness for URLLC slices deployed in industrial environments. We assess the agent performance with respect to resource allocation schemes that are INI agnostic. Results reveal that the proposed solution outperforms the benchmark schemes in terms of service provisioning performance in both network scenarios (e.g. mobile and industrial) and showcase the benefit of INI mitigation.
更多查看译文
关键词
Network slicing,Reinforcement learning,Interference
AI 理解论文
溯源树
样例
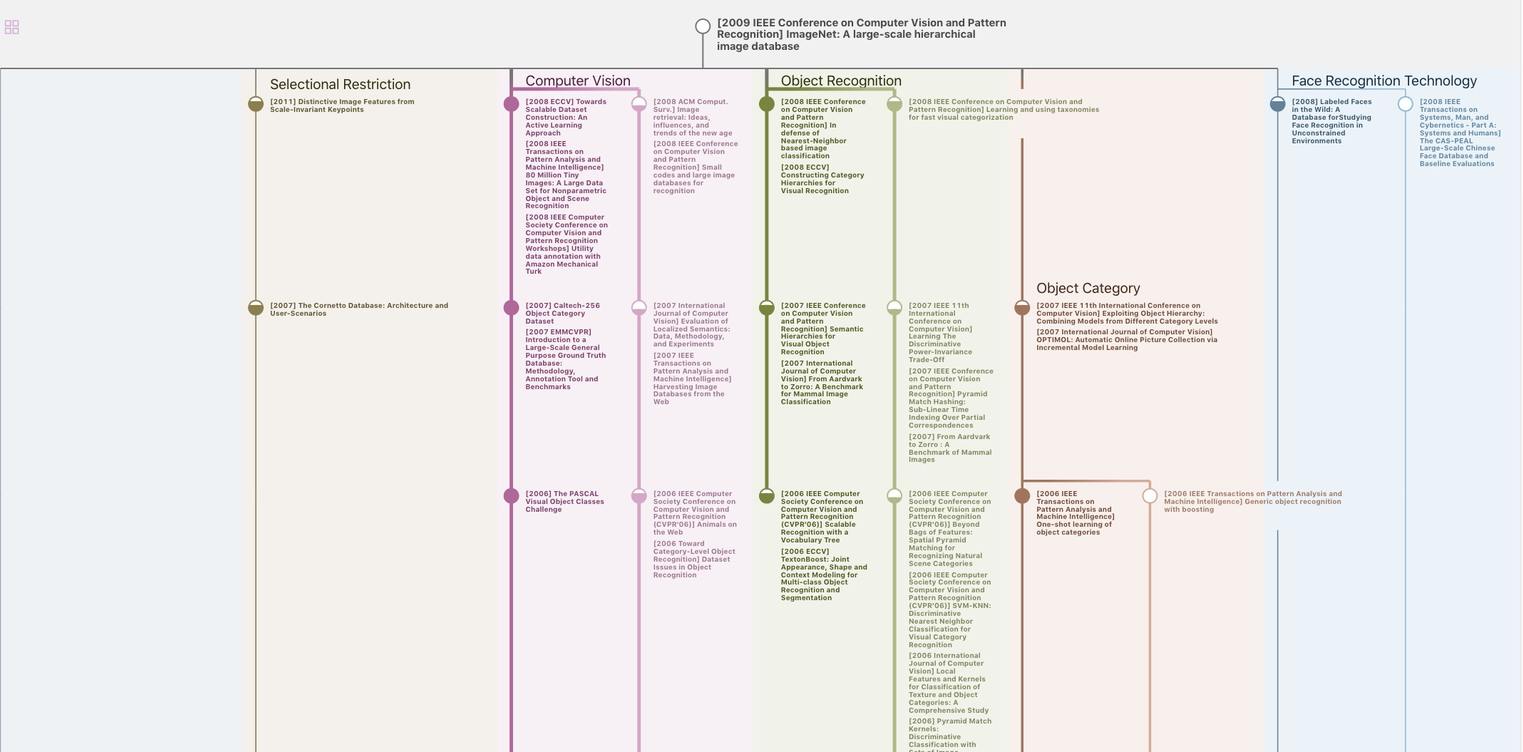
生成溯源树,研究论文发展脉络
Chat Paper
正在生成论文摘要