Can a Deep Progressive Learning Method Help to Achieve High Image Quality as Total-body PET Imaging?
Research Square (Research Square)(2021)
Abstract
Abstract Purpose To propose and validate a total-body PET (TB-PET) guided deep progressive learning method (DPR) for low-dose clinical imaging of standard axial field-of-view PET/CT scanner (SAFOV-PET).Methods List-mode raw data from a total of 182 scans were collected, including 100 patient scans from a TB-PET, and 15 phantom and 67 patient scans from a SAFOV-PET. Neural networks employed in DPR were trained with the high-quality images obtained from the TB-PET using a progressive learning strategy and evaluated on a SAFOV-PET through three stages of studies. The CTN phantom was firstly used to verify the effectiveness of protocols in DPR and OSEM algorithms. Subsequently, list-mode rawdata from retrospective and prospective PET oncological patients (n=26 and 41, respectively) were rebinned into short duration scans (referred as to DPR_full, DPR_1/2, DPR_1/3, and DPR_1/4), and reconstructed with DPR. Full-duration data were reconstructed with OSEM to generate images as a reference. In the retrospective study, the image quality was evaluated using the metrics of standard uptake value (SUV) and target-to-liver ratio (TLR) in lesions, and coefficient of variation (COV) in the liver, which provided evidence for the subsequent study with real-world low-dose injection. In the prospective study, the quantification accuracy was evaluated with the agreement of SUVs in the liver, the blood pool, and the muscle between the DPR and the OSEM images. Quantitative analysis was also performed with the SUV and the TLR in lesions, furthermore on small lesions with a diameter no more than 10mm. In addition, qualitative analysis was performed using a 5-point Likert scale on the following perspectives: contrast, noise, and diagnostic confidence. Results The protocols used in the study were verified to meet the EANM EARL2 requirements. In the retrospective study, the DPR group with one-third acquisition time can yield a comparable image quality to the reference. In the prospective study, good agreement of the SUVs between DPR and OSEM was found in all the selected background tissues even if the injected dose was reduced to 1/3. Both quantitative and qualitative results demonstrated that the DPR_1/3 group showed no significant difference with the reference regarding the liver COV and subjective scores. The lesion SUVs and TLRs in the DPR_1/3 group were significantly enhanced compared with the reference, even for small lesions. Conclusions The proposed DPR method can reduce the injected dose of SAFOV-PET scan by up to 2/3 in a real-world deployment while maintaining image quality.
MoreTranslated text
Key words
imaging,pet,high image quality,total-body
AI Read Science
Must-Reading Tree
Example
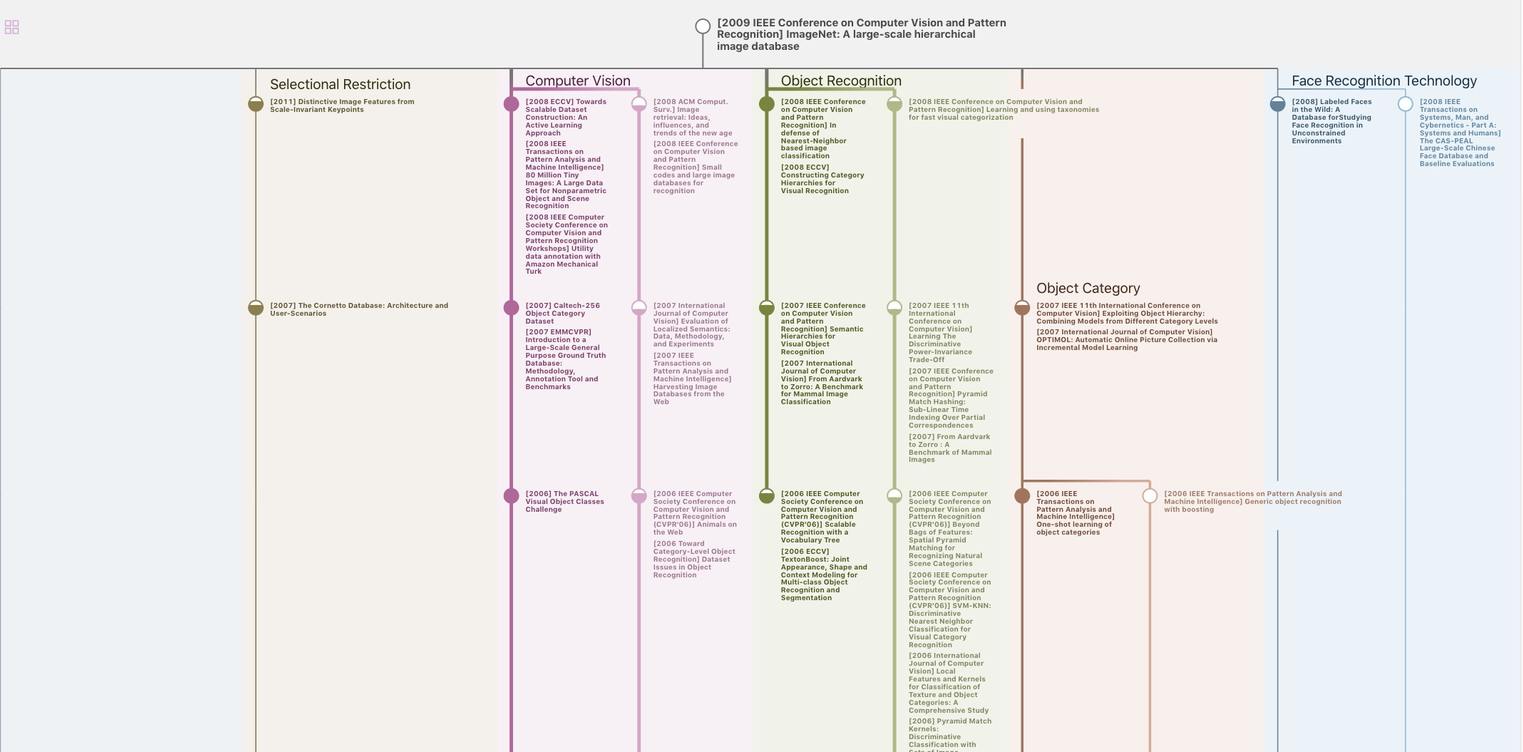
Generate MRT to find the research sequence of this paper
Chat Paper
Summary is being generated by the instructions you defined