B-MEG: Bottlenecked-Microservices Extraction Using Graph Neural Networks
ACM/SPEC International Conference on Performance Engineering(2022)
摘要
The microservices architecture enables independent development andmaintenanceofapplicationcomponentsthrough itsfine-grained and modular design. This has enabled rapid adoption of microservices architecture to build latency-sensitive online applications. In such online applications, it is critical to detect and mitigate sources of performance degradation (bottlenecks). However, the modular design of microservices architecture leads to a large graph of inter-acting microservices whose influence on each other is non-trivial. In this preliminary work, we explore the effectiveness of Graph Neural Network models in detecting bottlenecks. Preliminary analysis shows that our framework, B-MEG, produces promising results, especially for applications with complex call graphs. B-MEG shows up to 15% and 14% improvements in accuracy and precision, respec-tively, and close to 10 × increase in recall for detecting bottlenecks compared to the technique used in existing work for bottleneck detection in microservices [33].
更多查看译文
关键词
microservices, anomaly detection, bottleneck detection, graph neural networks, dataset
AI 理解论文
溯源树
样例
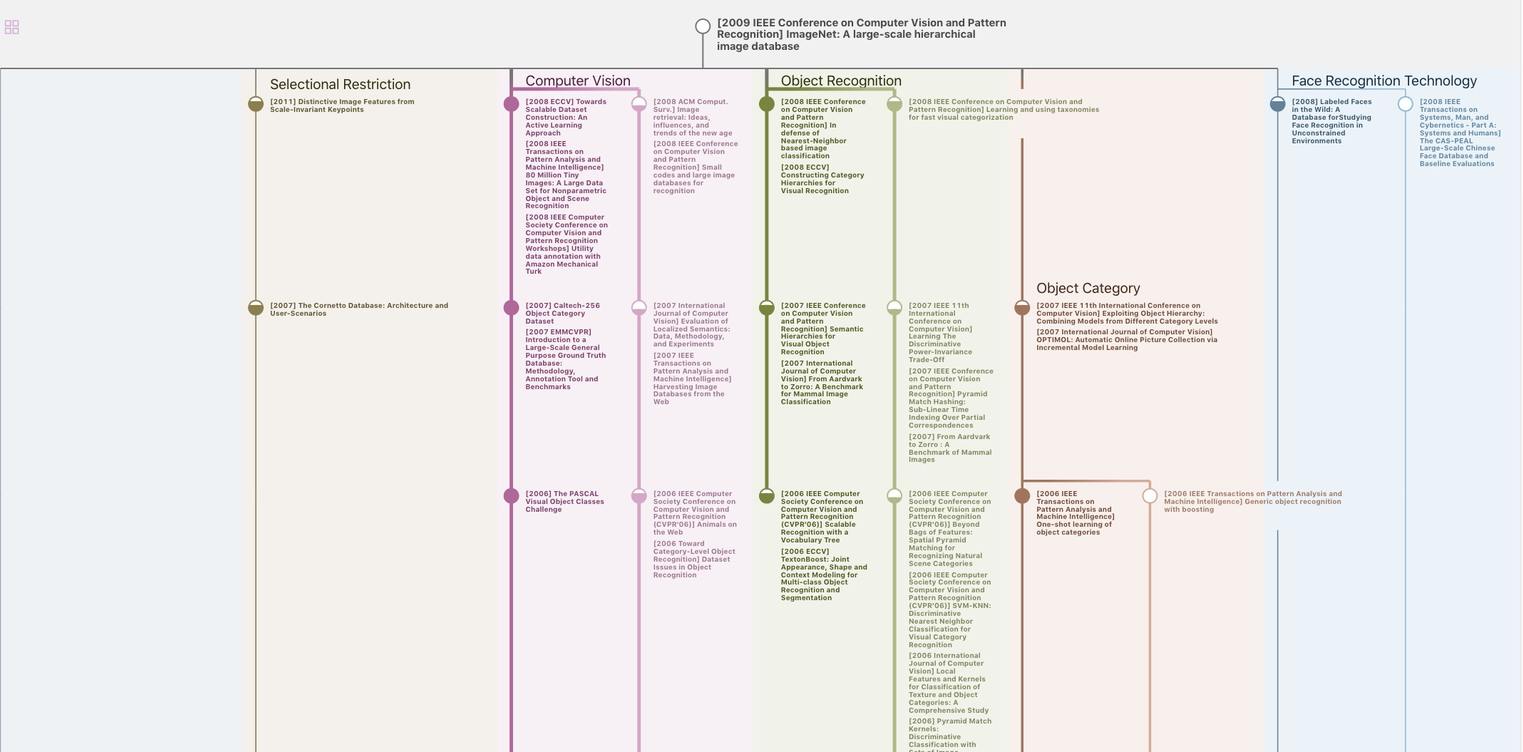
生成溯源树,研究论文发展脉络
Chat Paper
正在生成论文摘要