Hybrid model combining multivariate regression and machine learning for the rapid prediction of interior temperatures affected by thermal diodes and solar cavities
BUILDING AND ENVIRONMENT(2022)
Abstract
Sustainable design often requires highly efficient building performance evaluations. This study proposed a hybrid model combining multivariate regression modelling (MRM) and machine learning modelling (MLM) for the rapid prediction of interior temperatures affected by heat pipe thermal diodes and solar cavities based on experimental data. A heat pipe thermal diode can promote unidirectional heat transmission from the solar cavity on the south side of our newly built experimental house to the indoor environment to increase the interior temperature and reduce the heating load in cold climates. Experimental data were collected and then imported, cleaned, and split according to MRM and MLM requirements, respectively. In MRM, linear multivariate formulas were generated according to the thermal diode's two different working conditions. In MLM, a machine-learning model was created and trained using the experimental data. The results our hybrid model produced were comprehensively evaluated via R-square, statistical discrepancies, and complex MRM analyses. The similarity between the pre-diction and experimental results clearly demonstrates our model's accuracy and efficiency. This research was an original attempt to integrate emerging computational tools and provide a means to perform highly efficient quantitative analysis of indoor thermal environments for environmental studies and sustainable designs in the early stages.
MoreTranslated text
Key words
Hybrid model,Multivariate regression,Machine learning,Heat pipe thermal diode,Solar cavity,Rapid prediction
AI Read Science
Must-Reading Tree
Example
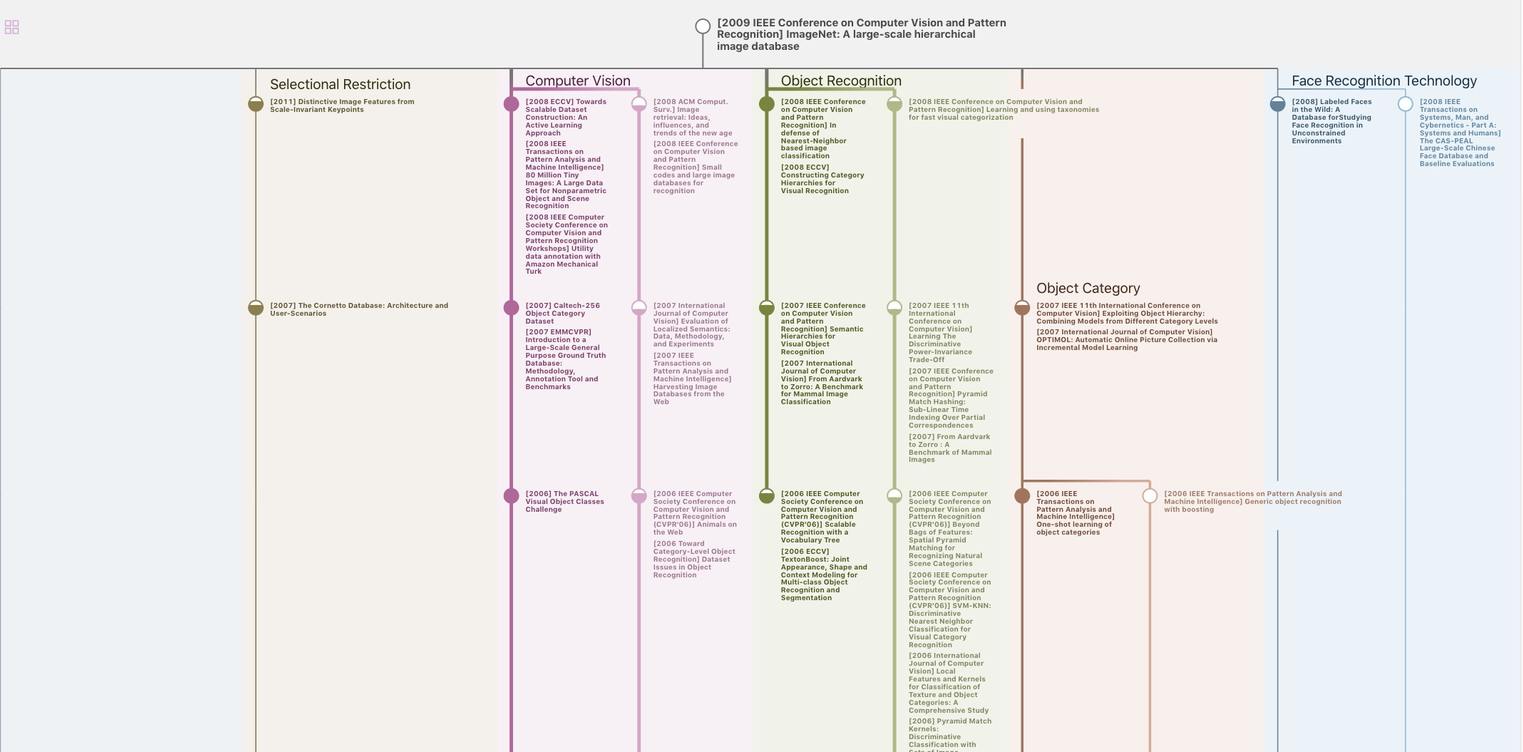
Generate MRT to find the research sequence of this paper
Chat Paper
Summary is being generated by the instructions you defined