Overview of CapsNet Performance Evaluation Methods for Image Classification using a Dual Input Capsule Network as a Case Study
International Journal of Computing and Digital Systems(2022)
摘要
Performance evaluation is a critical part of deep learning (DL) that requires careful conduct to enhance confidence and reliability. Several metrics exist to evaluate DL models, however, choosing one for a given model is not trivial, since it is not a one-fit-all solution. Practically, accuracy is the most popularly used evaluation metric for capsule networks (CapsNets). This is problematic for sensitive applications (e.g. health), since accuracy is overly optimistic in the presence of class imbalance, and does not permit the exact reporting of a model’s risk of bias and potential usefulness. This paper, therefore, aims at demonstrating the usefulness of other metrics for performance evaluation as well as interpretability through the implementation of a custom capsule model. The metrics are effective in measuring the real performance of the models in terms of accuracy (93.03% for proposed model), number of parameters ( ≈ 4 million fewer for proposed model), ability to scale and fail-safe, and the effectiveness of the routing process when evaluated on the datasets. Evaluating a CapsNet model with all these metrics has the potential to enhance the practitioner’s confidence and also improve model understandability and reliability.
更多查看译文
关键词
dual input capsule network,capsnet performance evaluation methods,image classification,performance evaluation
AI 理解论文
溯源树
样例
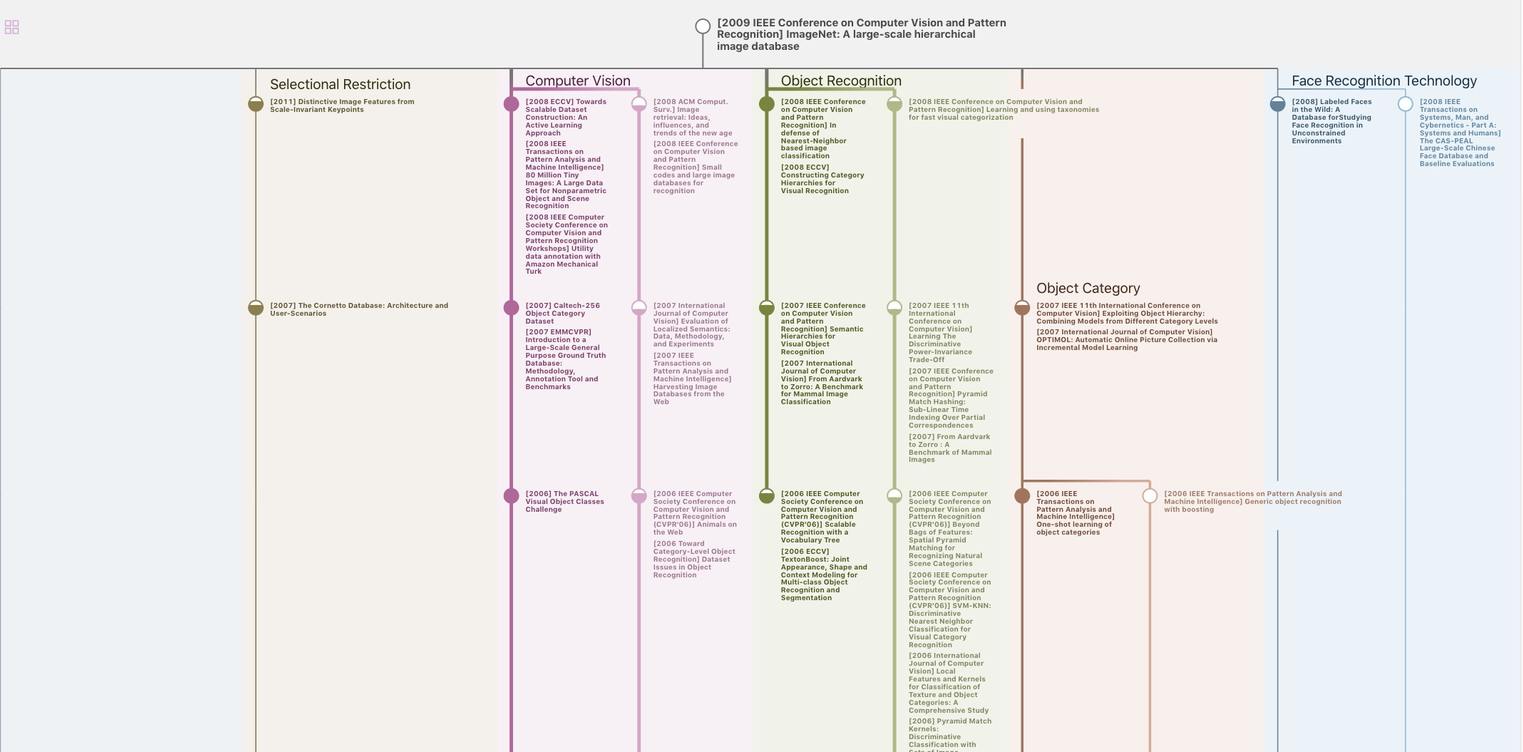
生成溯源树,研究论文发展脉络
Chat Paper
正在生成论文摘要