An explainability analysis of a sentiment prediction task using a transformer-based attention filter
semanticscholar(2021)
摘要
Transformer-based deep learning models have significantly improved the performance of machine learning in many natural language processing tasks in recent years. However, due to the computational complexity of the models’ attention mechanisms, the input length they can work with is currently limited. Inspired by the human tendency to ignore many words during a reading comprehension task, we experiment with the effect of removing tokens from a sequence for sentiment analysis. In this preliminary study, we analyze a length-reducing system based on layer-level attention scores. The expectation is that this allows us to discover what percentage of input length is required to obtain a reasonable amount of accuracy in a sentiment analysis task. We show that the filtering system based on BERT allows us to reduce sequences lengths of up to 99% in a sentiment analysis task while still obtaining 70% accuracy.
更多查看译文
AI 理解论文
溯源树
样例
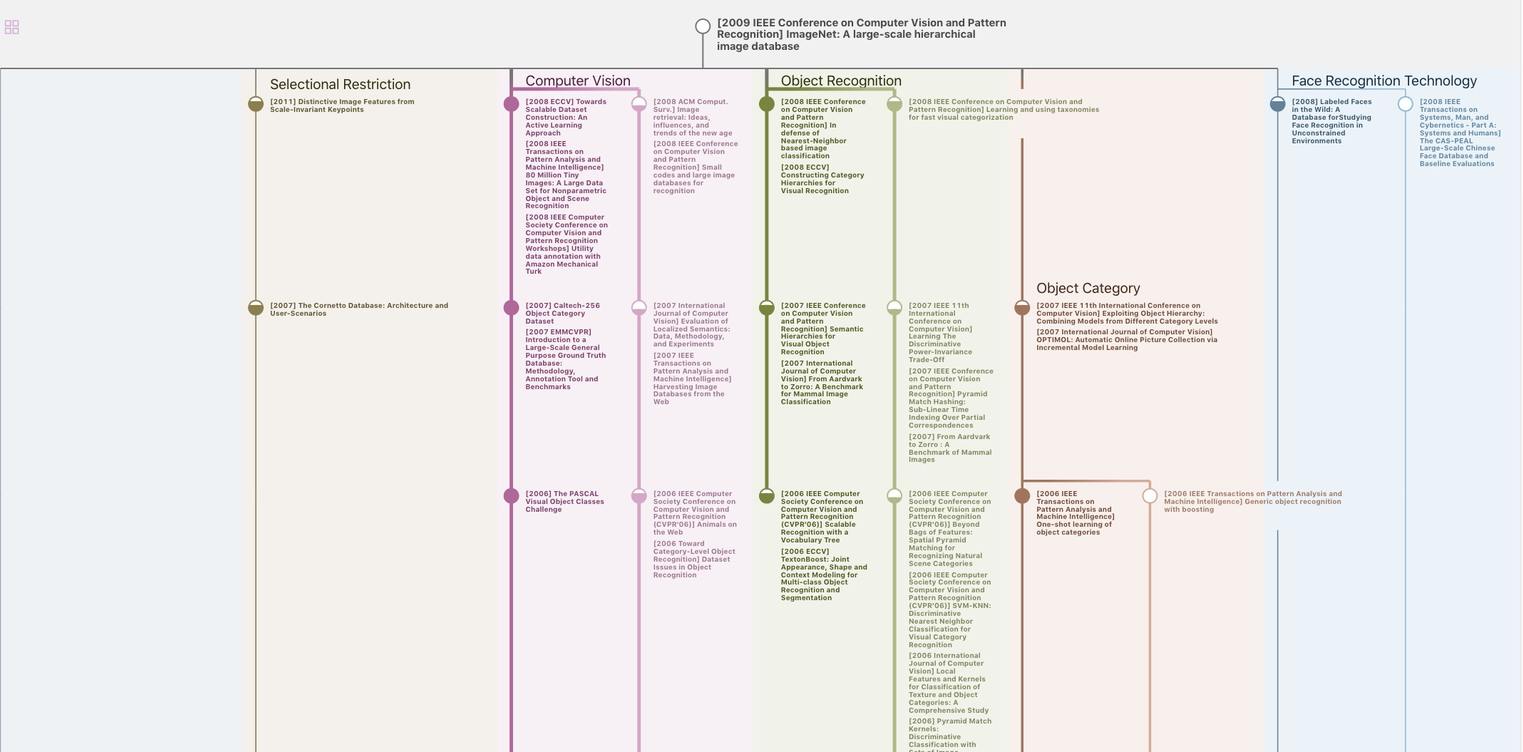
生成溯源树,研究论文发展脉络
Chat Paper
正在生成论文摘要