Data Augmentation with Adversarial Examples and Back-Translation
semanticscholar(2022)
摘要
Though question answering (QA) systems serve essential roles online and drive the websites of many Internet-based companies, they often perform poorly because of NLP models’ inability to learn deeply beyond their given training distribution. Here, we seek to improve upon basic QA models by implementing a robust QA system that can generalize to unseen, out-of-domain data. Our QA model is based on data augmentation, primarily with adversarial examples generated by a language model replacing one masked token in our original data, and backtranslation using Russian as a pivot language. Compared to the DistilBERT baseline dev F1 and EM of 47.72 and 30.63 respectively, our best data augmentation-based model achieved dev F1 and EM of 49.07 and 31.68, and test F1 and EM of 59.48 and 40.51.
更多查看译文
AI 理解论文
溯源树
样例
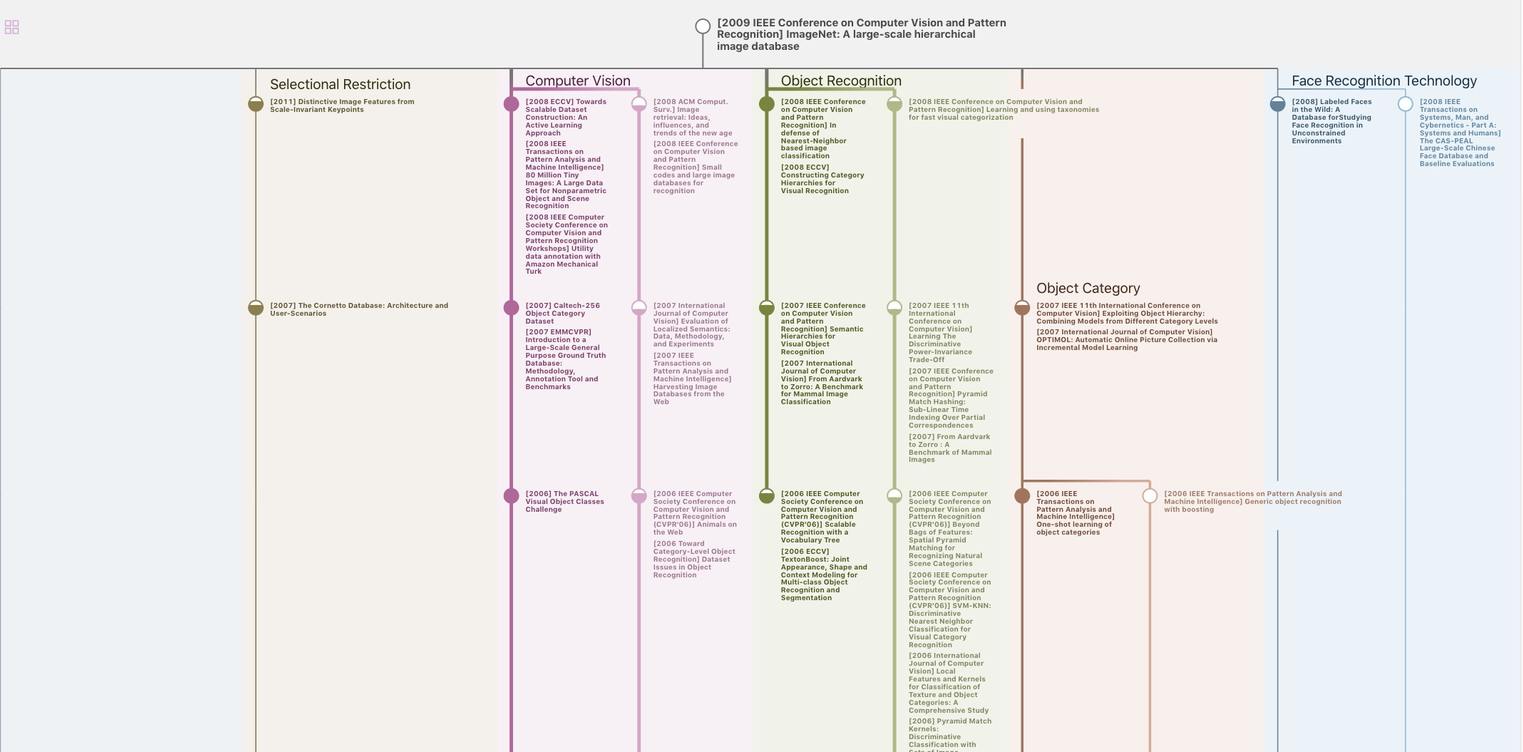
生成溯源树,研究论文发展脉络
Chat Paper
正在生成论文摘要