Short and Very Short Term Firm-level Load Forecasting for Warehouses: A Comparison of Machine Learning and Deep Learning Models
ENERGIES(2022)
摘要
Commercial buildings are a significant consumer of energy worldwide. Logistics facilities, and specifically warehouses, are a common building type yet under-researched in the demand-side energy forecasting literature. Warehouses have an idiosyncratic profile when compared to other commercial and industrial buildings with a significant reliance on a small number of energy systems. As such, warehouse owners and operators are increasingly entering in to energy performance contracts with energy service companies (ESCOs) to minimise environmental impact, reduce costs, and improve competitiveness. ESCOs and warehouse owners and operators require accurate forecasts of their energy consumption so that precautionary and mitigation measures can be taken. This paper explores the performance of three machine learning models (Support Vector Regression (SVR), Random Forest, and Extreme Gradient Boosting (XGBoost)), three deep learning models (Recurrent Neural Networks (RNN), Long Short-Term Memory (LSTM), and Gated Recurrent Unit (GRU)), and a classical time series model, Autoregressive Integrated Moving Average (ARIMA) for predicting daily energy consumption. The dataset comprises 8,040 records generated over an 11-month period from January to November 2020 from a non-refrigerated logistics facility located in Ireland. The grid search method was used to identify the best configurations for each model. The proposed XGBoost models outperform other models for both very short load forecasting (VSTLF) and short term load forecasting (STLF); the ARIMA model performed the worst.
更多查看译文
关键词
very short-term load forecasting, VSTLF, short-term load forecasting, STLF, deep learning, RNN, LSTM, GRU, machine learning, SVR, Random Forest, Extreme Gradient Boosting, energy consumption, ARIMA, time series prediction
AI 理解论文
溯源树
样例
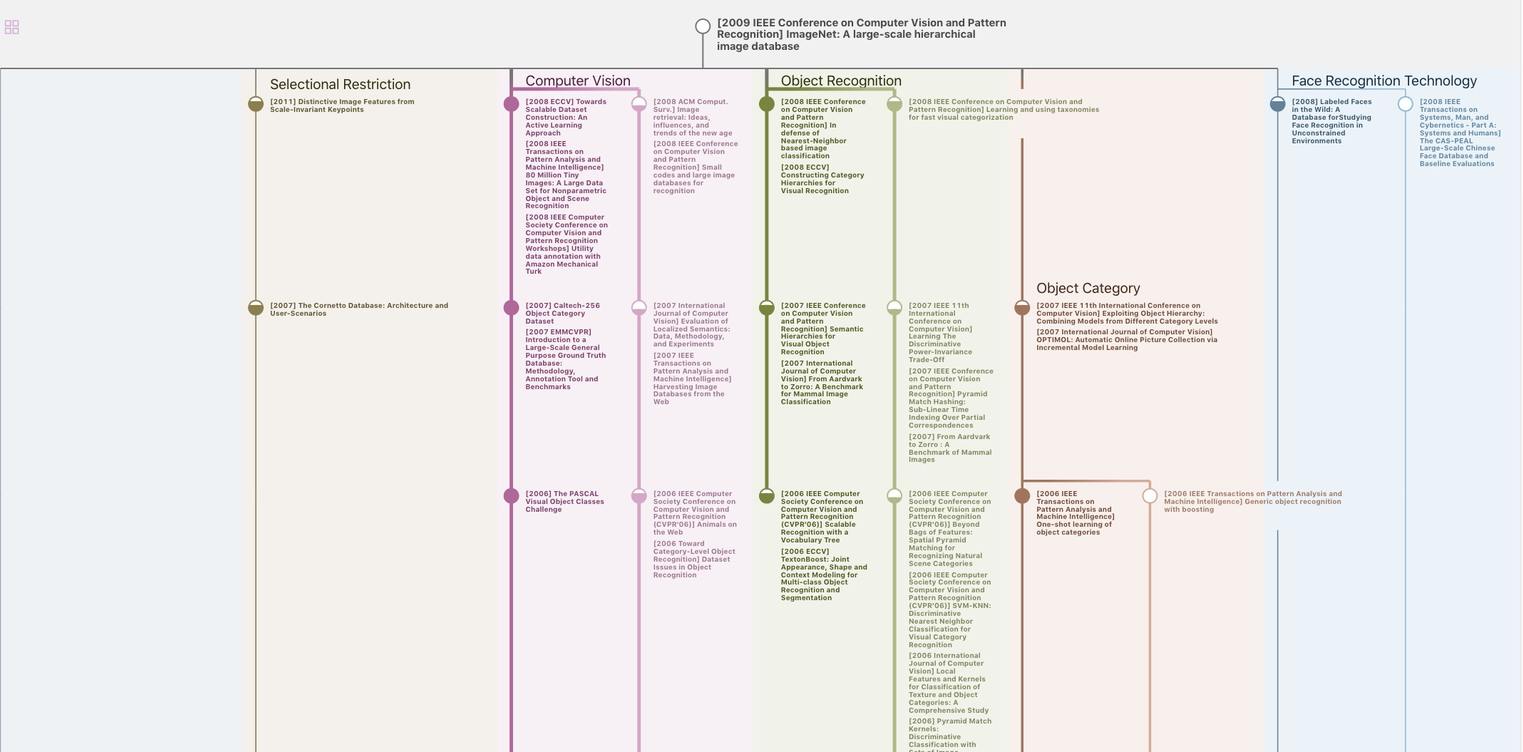
生成溯源树,研究论文发展脉络
Chat Paper
正在生成论文摘要