Developing an Interpretable Machine Learning Model to Predict In-Hospital Mortality in Sepsis Patients: A Retrospective Study of MIMIC-IV
semanticscholar(2022)
摘要
Background: Risk stratification plays an essential role in the decision-making of sepsis management, whereas neither a single serum biomarker nor traditional scoring tools can satisfy the need to assess this heterogeneous population comprehensively. The study intended to develop an interpretable machine learning model for predicting in-hospital mortality in critically ill patients with sepsis. Methods: Adult patients fulfilling the definition of Sepsis-3 were included in the Medical Information Mart for Intensive Care (MIMIC)-IV database. Relevant clinical features were extracted within the first 24 hours in ICU, and missing data were analyzed and imputed. We randomly separated the dataset into train and test sub-cohort by the ratio of 7:3, then an outcome-balanced train dataset was synthesized for model training. Extreme gradient boosting (XGBoost) was employed when feature selection and hyperparameter tuning were performed afterward. The fine-tuned XGBoost model was then compared with stepwise logistic regression (LR) and established severity scores. Eventually, we inspected the interpretability of the new model using XGBoost feature importance and Shapley Additive exPlanations (SHAP) plot. Results: The final cohort had 24,573 patients, of which 3,785 patients died during hospitalization (15.4%). Ten iterations of multiple imputations were executed to fill missing data in all 91 incomplete variables. Subsequently, 10,572 patients formed the balanced dataset used for training. The XGBoost model showed greater discrimination than stepwise LR and severity scores such as Simplified Acute Physiology Score (SAPS)-III score (AUC: 0.849, 95% CI: 0.8386-0.8599; AUC: 0.618, 95% CI: 0.3927-0.8437; AUC: 0.803, 95% CI: 0.7898-0.8165 respectively). Based on model interpretation, some decisive factors, including elevated lactate and anion gap level, prolonged partial thromboplastin time, decreased urine volume, were greatly correlated with poor survival outcomes in sepsis. Conclusions: In the field of predicting the mortality risk of sepsis patients in hospitals, the XGBoost-based model demonstrated superior performance to stepwise LR and other scores. In addition, the model exhibited good interpretability and might provide valuable hints on future directions of clinical practice and research.
更多查看译文
关键词
sepsis patients,interpretable machine learning model,mortality,in-hospital
AI 理解论文
溯源树
样例
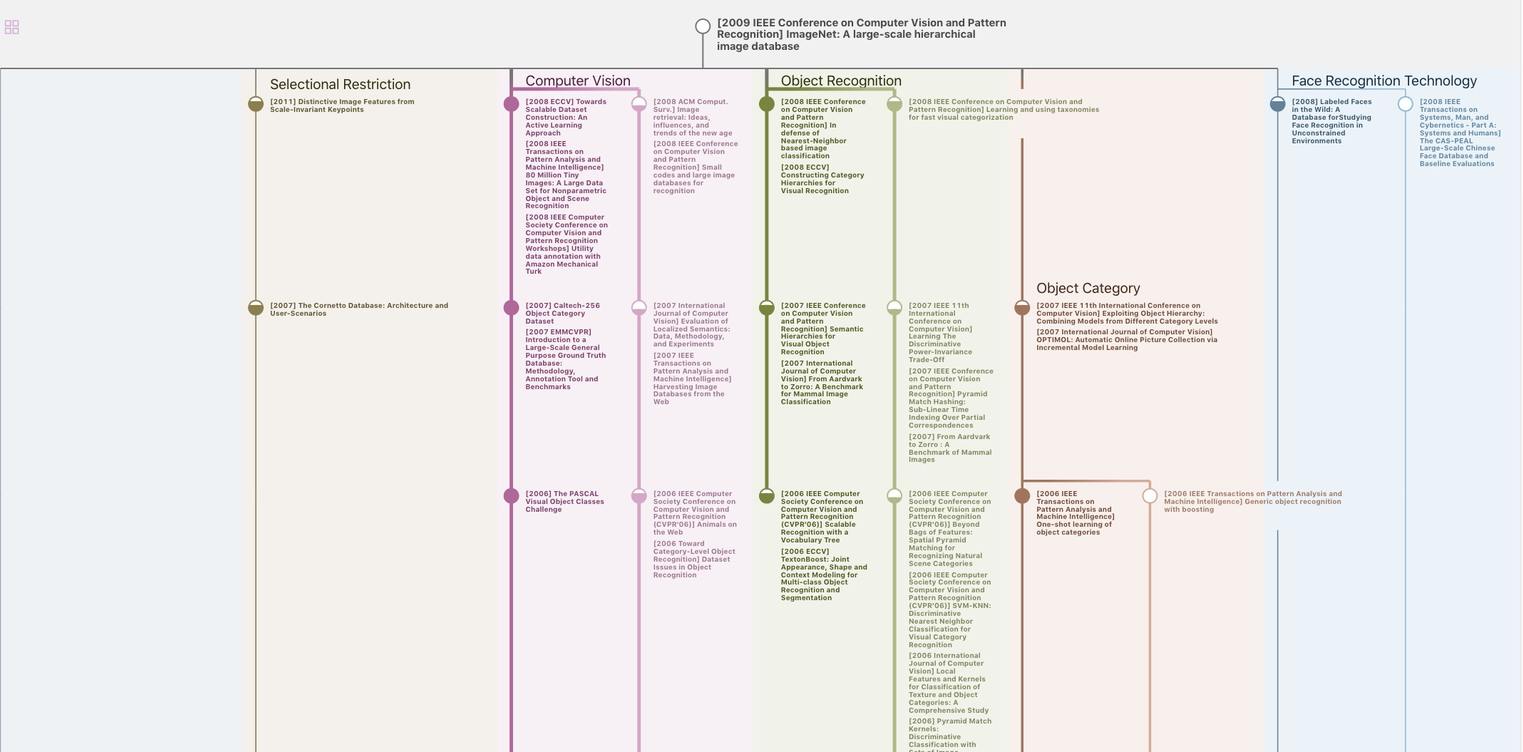
生成溯源树,研究论文发展脉络
Chat Paper
正在生成论文摘要