Bias amplification of unobserved confounding in pharmacoepidemiological studies: There is no free lunch in restricting the sample to those with a particular drug indication
medRxiv(2022)
摘要
Estimating causal effects in observational pharmacoepidemiology is a challenging task, as it is often plagued by confounding by indication. Restricting the sample to those with an indication for drug use is a commonly performed procedure; indication-based sampling ensures that the exposed and unexposed are exchangeable on the indication - limiting the potential for confounding by indication. However, indication-based sampling has received little scrutiny, despite the hazards of exposure-related covariate control. Using causal diagrams, simulations, and empirical examples, we demonstrate that indication-based sampling in the presence of unobserved confounding can give rise to bias amplification, a self-inflicted phenomenon where one inflates pre-existing bias through inappropriate covariate control. Additionally, we show that indication-based sampling generally leads to a greater net bias than alternative approaches, such as regression adjustment. Finally, we expand on how bias amplification should be reasoned about when distinct clinically relevant effects on the outcome among those with an indication (effect-heterogeneity) exist. We conclude that studies using indication-based sampling should have robust justification - and that it should by no means be considered a 'free lunch' to adopt such approaches. As such, we suggest that future observational studies stay wary of bias amplification when considering drug indications.
更多查看译文
关键词
bias amplification,causal inference,confounding,pharmacoepidemiology
AI 理解论文
溯源树
样例
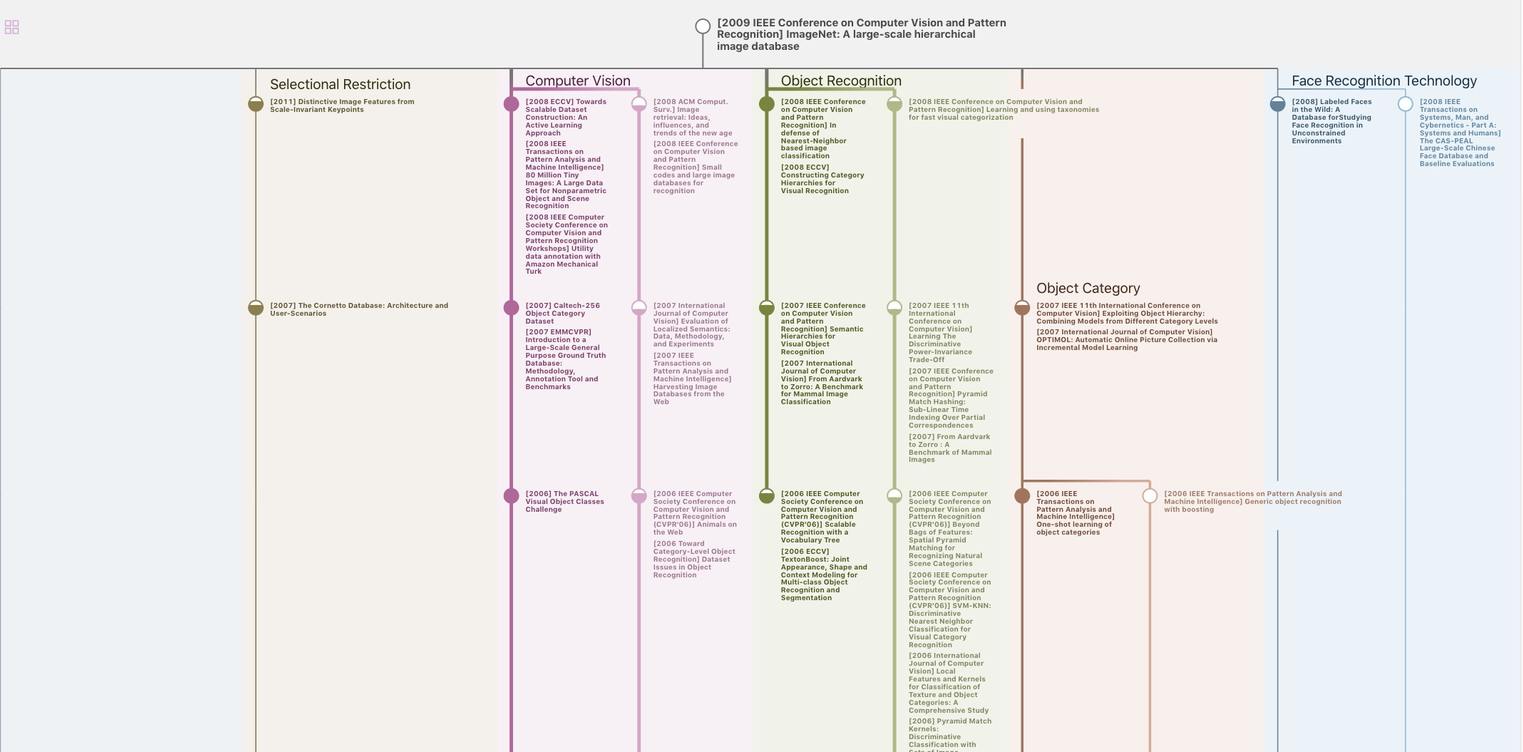
生成溯源树,研究论文发展脉络
Chat Paper
正在生成论文摘要